add wav2lip stream
This commit is contained in:
parent
39d7aff90a
commit
592312ab8c
32
README.md
32
README.md
|
@ -1,10 +1,10 @@
|
|||
Real time interactive streaming digital human, realize audio video synchronous dialogue. It can basically achieve commercial effects.
|
||||
实时交互流式数字人,实现音视频同步对话。基本可以达到商用效果
|
||||
|
||||
[ernerf效果](https://www.bilibili.com/video/BV1PM4m1y7Q2/) [musetalk效果](https://www.bilibili.com/video/BV1gm421N7vQ/)
|
||||
[ernerf效果](https://www.bilibili.com/video/BV1PM4m1y7Q2/) [musetalk效果](https://www.bilibili.com/video/BV1gm421N7vQ/) [wav2lip效果](https://www.bilibili.com/video/BV1Bw4m1e74P/)
|
||||
|
||||
## Features
|
||||
1. 支持多种数字人模型: ernerf、musetalk
|
||||
1. 支持多种数字人模型: ernerf、musetalk、wav2lip
|
||||
2. 支持声音克隆
|
||||
3. 支持多种音频特征驱动:wav2vec、hubert
|
||||
4. 支持全身视频拼接
|
||||
|
@ -23,7 +23,7 @@ conda create -n nerfstream python=3.10
|
|||
conda activate nerfstream
|
||||
conda install pytorch==1.12.1 torchvision==0.13.1 cudatoolkit=11.3 -c pytorch
|
||||
pip install -r requirements.txt
|
||||
#如果只用musetalk模型,不需要安装下面的库
|
||||
#如果只用musetalk或者wav2lip模型,不需要安装下面的库
|
||||
pip install "git+https://github.com/facebookresearch/pytorch3d.git"
|
||||
pip install tensorflow-gpu==2.8.0
|
||||
pip install --upgrade "protobuf<=3.20.1"
|
||||
|
@ -171,13 +171,30 @@ cd MuseTalk
|
|||
python -m scripts.realtime_inference --inference_config configs/inference/realtime.yaml
|
||||
运行后将results/avatars下文件拷到本项目的data/avatars下
|
||||
```
|
||||
|
||||
### 3.10 模型用wav2lip
|
||||
暂不支持rtmp推送
|
||||
- 下载模型
|
||||
下载wav2lip运行需要的模型,网盘地址 https://drive.uc.cn/s/3683da52551a4
|
||||
将s3fd.pth拷到本项目wav2lip/face_detection/detection/sfd/s3fd.pth, 将wav2lip.pth拷到本项目的models下
|
||||
数字人模型文件 wav2lip_avatar1.tar.gz, 解压后将整个文件夹拷到本项目的data/avatars下
|
||||
- 运行
|
||||
python app.py --transport webrtc --model wav2lip --avatar_id wav2lip_avatar1
|
||||
用浏览器打开http://serverip:8010/webrtcapi.html
|
||||
可以设置--batch_size 提高显卡利用率,设置--avatar_id 运行不同的数字人
|
||||
#### 替换成自己的数字人
|
||||
```bash
|
||||
cd wav2lip
|
||||
python genavatar.py --video_path xxx.mp4
|
||||
运行后将results/avatars下文件拷到本项目的data/avatars下
|
||||
```
|
||||
|
||||
## 4. Docker Run
|
||||
不需要第1步的安装,直接运行。
|
||||
不需要前面的安装,直接运行。
|
||||
```
|
||||
docker run --gpus all -it --network=host --rm registry.cn-hangzhou.aliyuncs.com/lipku/nerfstream:v1.3
|
||||
docker run --gpus all -it --network=host --rm registry.cn-beijing.aliyuncs.com/codewithgpu2/lipku-metahuman-stream:TzZGB72JKt
|
||||
```
|
||||
docker版本已经不是最新代码,可以作为一个空环境,把最新代码拷进去运行。
|
||||
代码在/root/metahuman-stream,先git pull拉一下最新代码,然后执行命令同第2、3步
|
||||
|
||||
另外提供autodl镜像:
|
||||
https://www.codewithgpu.com/i/lipku/metahuman-stream/base
|
||||
|
@ -211,10 +228,11 @@ https://www.codewithgpu.com/i/lipku/metahuman-stream/base
|
|||
- [x] 声音克隆
|
||||
- [x] 数字人静音时用一段视频代替
|
||||
- [x] MuseTalk
|
||||
- [x] Wav2Lip
|
||||
- [ ] SyncTalk
|
||||
|
||||
如果本项目对你有帮助,帮忙点个star。也欢迎感兴趣的朋友一起来完善该项目。
|
||||
知识星球: https://t.zsxq.com/7NMyO
|
||||
知识星球: https://t.zsxq.com/7NMyO 沉淀高质量常见问题、最佳实践经验、问题解答
|
||||
微信公众号:数字人技术
|
||||
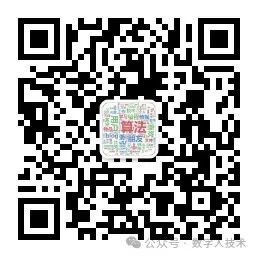
|
||||
|
||||
|
|
6
app.py
6
app.py
|
@ -295,7 +295,7 @@ if __name__ == '__main__':
|
|||
# parser.add_argument('--CHARACTER', type=str, default='test')
|
||||
# parser.add_argument('--EMOTION', type=str, default='default')
|
||||
|
||||
parser.add_argument('--model', type=str, default='ernerf') #musetalk
|
||||
parser.add_argument('--model', type=str, default='ernerf') #musetalk wav2lip
|
||||
|
||||
parser.add_argument('--transport', type=str, default='rtcpush') #rtmp webrtc rtcpush
|
||||
parser.add_argument('--push_url', type=str, default='http://localhost:1985/rtc/v1/whip/?app=live&stream=livestream') #rtmp://localhost/live/livestream
|
||||
|
@ -357,6 +357,10 @@ if __name__ == '__main__':
|
|||
from musereal import MuseReal
|
||||
print(opt)
|
||||
nerfreal = MuseReal(opt)
|
||||
elif opt.model == 'wav2lip':
|
||||
from lipreal import LipReal
|
||||
print(opt)
|
||||
nerfreal = LipReal(opt)
|
||||
|
||||
#txt_to_audio('我是中国人,我来自北京')
|
||||
if opt.transport=='rtmp':
|
||||
|
|
|
@ -0,0 +1,98 @@
|
|||
import time
|
||||
import torch
|
||||
import numpy as np
|
||||
import soundfile as sf
|
||||
import resampy
|
||||
|
||||
import queue
|
||||
from queue import Queue
|
||||
from io import BytesIO
|
||||
import multiprocessing as mp
|
||||
|
||||
from wav2lip import audio
|
||||
|
||||
class LipASR:
|
||||
def __init__(self, opt):
|
||||
self.opt = opt
|
||||
|
||||
self.fps = opt.fps # 20 ms per frame
|
||||
self.sample_rate = 16000
|
||||
self.chunk = self.sample_rate // self.fps # 320 samples per chunk (20ms * 16000 / 1000)
|
||||
self.queue = Queue()
|
||||
# self.input_stream = BytesIO()
|
||||
self.output_queue = mp.Queue()
|
||||
|
||||
#self.audio_processor = audio_processor
|
||||
self.batch_size = opt.batch_size
|
||||
|
||||
self.frames = []
|
||||
self.stride_left_size = self.stride_right_size = 10
|
||||
self.context_size = 10
|
||||
self.audio_feats = []
|
||||
self.feat_queue = mp.Queue(5)
|
||||
|
||||
self.warm_up()
|
||||
|
||||
def put_audio_frame(self,audio_chunk): #16khz 20ms pcm
|
||||
self.queue.put(audio_chunk)
|
||||
|
||||
def __get_audio_frame(self):
|
||||
try:
|
||||
frame = self.queue.get(block=True,timeout=0.018)
|
||||
type = 0
|
||||
#print(f'[INFO] get frame {frame.shape}')
|
||||
except queue.Empty:
|
||||
frame = np.zeros(self.chunk, dtype=np.float32)
|
||||
type = 1
|
||||
|
||||
return frame,type
|
||||
|
||||
def get_audio_out(self): #get origin audio pcm to nerf
|
||||
return self.output_queue.get()
|
||||
|
||||
def warm_up(self):
|
||||
for _ in range(self.stride_left_size + self.stride_right_size):
|
||||
audio_frame,type=self.__get_audio_frame()
|
||||
self.frames.append(audio_frame)
|
||||
self.output_queue.put((audio_frame,type))
|
||||
for _ in range(self.stride_left_size):
|
||||
self.output_queue.get()
|
||||
|
||||
def run_step(self):
|
||||
############################################## extract audio feature ##############################################
|
||||
# get a frame of audio
|
||||
for _ in range(self.batch_size*2):
|
||||
frame,type = self.__get_audio_frame()
|
||||
self.frames.append(frame)
|
||||
# put to output
|
||||
self.output_queue.put((frame,type))
|
||||
# context not enough, do not run network.
|
||||
if len(self.frames) < self.stride_left_size + self.context_size + self.stride_right_size:
|
||||
return
|
||||
|
||||
inputs = np.concatenate(self.frames) # [N * chunk]
|
||||
mel = audio.melspectrogram(inputs)
|
||||
#print(mel.shape[0],mel.shape,len(mel[0]),len(self.frames))
|
||||
# cut off stride
|
||||
left = max(0, self.stride_left_size*80/50)
|
||||
right = min(len(mel[0]), len(mel[0]) - self.stride_right_size*80/50)
|
||||
mel_idx_multiplier = 80.*2/self.fps
|
||||
mel_step_size = 16
|
||||
i = 0
|
||||
mel_chunks = []
|
||||
while i < (len(self.frames)-self.stride_left_size-self.stride_right_size)/2:
|
||||
start_idx = int(left + i * mel_idx_multiplier)
|
||||
#print(start_idx)
|
||||
if start_idx + mel_step_size > len(mel[0]):
|
||||
mel_chunks.append(mel[:, len(mel[0]) - mel_step_size:])
|
||||
else:
|
||||
mel_chunks.append(mel[:, start_idx : start_idx + mel_step_size])
|
||||
i += 1
|
||||
self.feat_queue.put(mel_chunks)
|
||||
|
||||
# discard the old part to save memory
|
||||
self.frames = self.frames[-(self.stride_left_size + self.stride_right_size):]
|
||||
|
||||
|
||||
def get_next_feat(self,block,timeout):
|
||||
return self.feat_queue.get(block,timeout)
|
|
@ -0,0 +1,269 @@
|
|||
import math
|
||||
import torch
|
||||
import numpy as np
|
||||
|
||||
#from .utils import *
|
||||
import subprocess
|
||||
import os
|
||||
import time
|
||||
import cv2
|
||||
import glob
|
||||
import pickle
|
||||
import copy
|
||||
|
||||
import queue
|
||||
from queue import Queue
|
||||
from threading import Thread, Event
|
||||
from io import BytesIO
|
||||
import multiprocessing as mp
|
||||
|
||||
|
||||
from ttsreal import EdgeTTS,VoitsTTS,XTTS
|
||||
|
||||
from lipasr import LipASR
|
||||
import asyncio
|
||||
from av import AudioFrame, VideoFrame
|
||||
|
||||
from wav2lip.models import Wav2Lip
|
||||
|
||||
from tqdm import tqdm
|
||||
|
||||
device = 'cuda' if torch.cuda.is_available() else 'cpu'
|
||||
print('Using {} for inference.'.format(device))
|
||||
|
||||
def _load(checkpoint_path):
|
||||
if device == 'cuda':
|
||||
checkpoint = torch.load(checkpoint_path)
|
||||
else:
|
||||
checkpoint = torch.load(checkpoint_path,
|
||||
map_location=lambda storage, loc: storage)
|
||||
return checkpoint
|
||||
|
||||
def load_model(path):
|
||||
model = Wav2Lip()
|
||||
print("Load checkpoint from: {}".format(path))
|
||||
checkpoint = _load(path)
|
||||
s = checkpoint["state_dict"]
|
||||
new_s = {}
|
||||
for k, v in s.items():
|
||||
new_s[k.replace('module.', '')] = v
|
||||
model.load_state_dict(new_s)
|
||||
|
||||
model = model.to(device)
|
||||
return model.eval()
|
||||
|
||||
def read_imgs(img_list):
|
||||
frames = []
|
||||
print('reading images...')
|
||||
for img_path in tqdm(img_list):
|
||||
frame = cv2.imread(img_path)
|
||||
frames.append(frame)
|
||||
return frames
|
||||
|
||||
def __mirror_index(size, index):
|
||||
#size = len(self.coord_list_cycle)
|
||||
turn = index // size
|
||||
res = index % size
|
||||
if turn % 2 == 0:
|
||||
return res
|
||||
else:
|
||||
return size - res - 1
|
||||
|
||||
def inference(render_event,batch_size,face_imgs_path,audio_feat_queue,audio_out_queue,res_frame_queue):
|
||||
|
||||
model = load_model("./models/wav2lip.pth")
|
||||
input_face_list = glob.glob(os.path.join(face_imgs_path, '*.[jpJP][pnPN]*[gG]'))
|
||||
input_face_list = sorted(input_face_list, key=lambda x: int(os.path.splitext(os.path.basename(x))[0]))
|
||||
face_list_cycle = read_imgs(input_face_list)
|
||||
|
||||
#input_latent_list_cycle = torch.load(latents_out_path)
|
||||
length = len(face_list_cycle)
|
||||
index = 0
|
||||
count=0
|
||||
counttime=0
|
||||
print('start inference')
|
||||
while True:
|
||||
if render_event.is_set():
|
||||
starttime=time.perf_counter()
|
||||
mel_batch = []
|
||||
try:
|
||||
mel_batch = audio_feat_queue.get(block=True, timeout=1)
|
||||
except queue.Empty:
|
||||
continue
|
||||
|
||||
is_all_silence=True
|
||||
audio_frames = []
|
||||
for _ in range(batch_size*2):
|
||||
frame,type = audio_out_queue.get()
|
||||
audio_frames.append((frame,type))
|
||||
if type==0:
|
||||
is_all_silence=False
|
||||
|
||||
if is_all_silence:
|
||||
for i in range(batch_size):
|
||||
res_frame_queue.put((None,__mirror_index(length,index),audio_frames[i*2:i*2+2]))
|
||||
index = index + 1
|
||||
else:
|
||||
# print('infer=======')
|
||||
t=time.perf_counter()
|
||||
img_batch = []
|
||||
for i in range(batch_size):
|
||||
idx = __mirror_index(length,index+i)
|
||||
face = face_list_cycle[idx]
|
||||
img_batch.append(face)
|
||||
img_batch, mel_batch = np.asarray(img_batch), np.asarray(mel_batch)
|
||||
|
||||
img_masked = img_batch.copy()
|
||||
img_masked[:, face.shape[0]//2:] = 0
|
||||
|
||||
img_batch = np.concatenate((img_masked, img_batch), axis=3) / 255.
|
||||
mel_batch = np.reshape(mel_batch, [len(mel_batch), mel_batch.shape[1], mel_batch.shape[2], 1])
|
||||
|
||||
img_batch = torch.FloatTensor(np.transpose(img_batch, (0, 3, 1, 2))).to(device)
|
||||
mel_batch = torch.FloatTensor(np.transpose(mel_batch, (0, 3, 1, 2))).to(device)
|
||||
|
||||
with torch.no_grad():
|
||||
pred = model(mel_batch, img_batch)
|
||||
pred = pred.cpu().numpy().transpose(0, 2, 3, 1) * 255.
|
||||
|
||||
counttime += (time.perf_counter() - t)
|
||||
count += batch_size
|
||||
#_totalframe += 1
|
||||
if count>=100:
|
||||
print(f"------actual avg infer fps:{count/counttime:.4f}")
|
||||
count=0
|
||||
counttime=0
|
||||
for i,res_frame in enumerate(pred):
|
||||
#self.__pushmedia(res_frame,loop,audio_track,video_track)
|
||||
res_frame_queue.put((res_frame,__mirror_index(length,index),audio_frames[i*2:i*2+2]))
|
||||
index = index + 1
|
||||
#print('total batch time:',time.perf_counter()-starttime)
|
||||
else:
|
||||
time.sleep(1)
|
||||
print('musereal inference processor stop')
|
||||
|
||||
@torch.no_grad()
|
||||
class LipReal:
|
||||
def __init__(self, opt):
|
||||
self.opt = opt # shared with the trainer's opt to support in-place modification of rendering parameters.
|
||||
self.W = opt.W
|
||||
self.H = opt.H
|
||||
|
||||
self.fps = opt.fps # 20 ms per frame
|
||||
|
||||
#### musetalk
|
||||
self.avatar_id = opt.avatar_id
|
||||
self.avatar_path = f"./data/avatars/{self.avatar_id}"
|
||||
self.full_imgs_path = f"{self.avatar_path}/full_imgs"
|
||||
self.face_imgs_path = f"{self.avatar_path}/face_imgs"
|
||||
self.coords_path = f"{self.avatar_path}/coords.pkl"
|
||||
self.batch_size = opt.batch_size
|
||||
self.idx = 0
|
||||
self.res_frame_queue = mp.Queue(self.batch_size*2)
|
||||
#self.__loadmodels()
|
||||
self.__loadavatar()
|
||||
|
||||
self.asr = LipASR(opt)
|
||||
if opt.tts == "edgetts":
|
||||
self.tts = EdgeTTS(opt,self)
|
||||
elif opt.tts == "gpt-sovits":
|
||||
self.tts = VoitsTTS(opt,self)
|
||||
elif opt.tts == "xtts":
|
||||
self.tts = XTTS(opt,self)
|
||||
#self.__warm_up()
|
||||
|
||||
self.render_event = mp.Event()
|
||||
mp.Process(target=inference, args=(self.render_event,self.batch_size,self.face_imgs_path,
|
||||
self.asr.feat_queue,self.asr.output_queue,self.res_frame_queue,
|
||||
)).start()
|
||||
|
||||
# def __loadmodels(self):
|
||||
# # load model weights
|
||||
# self.audio_processor, self.vae, self.unet, self.pe = load_all_model()
|
||||
# device = torch.device("cuda" if torch.cuda.is_available() else "cpu")
|
||||
# self.timesteps = torch.tensor([0], device=device)
|
||||
# self.pe = self.pe.half()
|
||||
# self.vae.vae = self.vae.vae.half()
|
||||
# self.unet.model = self.unet.model.half()
|
||||
|
||||
def __loadavatar(self):
|
||||
with open(self.coords_path, 'rb') as f:
|
||||
self.coord_list_cycle = pickle.load(f)
|
||||
input_img_list = glob.glob(os.path.join(self.full_imgs_path, '*.[jpJP][pnPN]*[gG]'))
|
||||
input_img_list = sorted(input_img_list, key=lambda x: int(os.path.splitext(os.path.basename(x))[0]))
|
||||
self.frame_list_cycle = read_imgs(input_img_list)
|
||||
|
||||
|
||||
def put_msg_txt(self,msg):
|
||||
self.tts.put_msg_txt(msg)
|
||||
|
||||
def put_audio_frame(self,audio_chunk): #16khz 20ms pcm
|
||||
self.asr.put_audio_frame(audio_chunk)
|
||||
|
||||
|
||||
def process_frames(self,quit_event,loop=None,audio_track=None,video_track=None):
|
||||
|
||||
while not quit_event.is_set():
|
||||
try:
|
||||
res_frame,idx,audio_frames = self.res_frame_queue.get(block=True, timeout=1)
|
||||
except queue.Empty:
|
||||
continue
|
||||
if audio_frames[0][1]==1 and audio_frames[1][1]==1: #全为静音数据,只需要取fullimg
|
||||
combine_frame = self.frame_list_cycle[idx]
|
||||
else:
|
||||
bbox = self.coord_list_cycle[idx]
|
||||
combine_frame = copy.deepcopy(self.frame_list_cycle[idx])
|
||||
y1, y2, x1, x2 = bbox
|
||||
try:
|
||||
res_frame = cv2.resize(res_frame.astype(np.uint8),(x2-x1,y2-y1))
|
||||
except:
|
||||
continue
|
||||
#combine_frame = get_image(ori_frame,res_frame,bbox)
|
||||
#t=time.perf_counter()
|
||||
combine_frame[y1:y2, x1:x2] = res_frame
|
||||
#print('blending time:',time.perf_counter()-t)
|
||||
|
||||
image = combine_frame #(outputs['image'] * 255).astype(np.uint8)
|
||||
new_frame = VideoFrame.from_ndarray(image, format="bgr24")
|
||||
asyncio.run_coroutine_threadsafe(video_track._queue.put(new_frame), loop)
|
||||
|
||||
for audio_frame in audio_frames:
|
||||
frame,type = audio_frame
|
||||
frame = (frame * 32767).astype(np.int16)
|
||||
new_frame = AudioFrame(format='s16', layout='mono', samples=frame.shape[0])
|
||||
new_frame.planes[0].update(frame.tobytes())
|
||||
new_frame.sample_rate=16000
|
||||
# if audio_track._queue.qsize()>10:
|
||||
# time.sleep(0.1)
|
||||
asyncio.run_coroutine_threadsafe(audio_track._queue.put(new_frame), loop)
|
||||
print('musereal process_frames thread stop')
|
||||
|
||||
def render(self,quit_event,loop=None,audio_track=None,video_track=None):
|
||||
#if self.opt.asr:
|
||||
# self.asr.warm_up()
|
||||
|
||||
self.tts.render(quit_event)
|
||||
process_thread = Thread(target=self.process_frames, args=(quit_event,loop,audio_track,video_track))
|
||||
process_thread.start()
|
||||
|
||||
self.render_event.set() #start infer process render
|
||||
count=0
|
||||
totaltime=0
|
||||
_starttime=time.perf_counter()
|
||||
#_totalframe=0
|
||||
while not quit_event.is_set():
|
||||
# update texture every frame
|
||||
# audio stream thread...
|
||||
t = time.perf_counter()
|
||||
self.asr.run_step()
|
||||
|
||||
if video_track._queue.qsize()>=2*self.opt.batch_size:
|
||||
print('sleep qsize=',video_track._queue.qsize())
|
||||
time.sleep(0.04*self.opt.batch_size*1.5)
|
||||
|
||||
# delay = _starttime+_totalframe*0.04-time.perf_counter() #40ms
|
||||
# if delay > 0:
|
||||
# time.sleep(delay)
|
||||
self.render_event.clear() #end infer process render
|
||||
print('musereal thread stop')
|
||||
|
|
@ -38,3 +38,5 @@ ffmpeg-python
|
|||
omegaconf
|
||||
diffusers
|
||||
accelerate
|
||||
|
||||
librosa
|
||||
|
|
|
@ -4,7 +4,7 @@ import numpy as np
|
|||
# import tensorflow as tf
|
||||
from scipy import signal
|
||||
from scipy.io import wavfile
|
||||
from hparams import hparams as hp
|
||||
from .hparams import hparams as hp
|
||||
|
||||
def load_wav(path, sr):
|
||||
return librosa.core.load(path, sr=sr)[0]
|
||||
|
@ -97,7 +97,7 @@ def _linear_to_mel(spectogram):
|
|||
|
||||
def _build_mel_basis():
|
||||
assert hp.fmax <= hp.sample_rate // 2
|
||||
return librosa.filters.mel(hp.sample_rate, hp.n_fft, n_mels=hp.num_mels,
|
||||
return librosa.filters.mel(sr=float(hp.sample_rate), n_fft=hp.n_fft, n_mels=hp.num_mels,
|
||||
fmin=hp.fmin, fmax=hp.fmax)
|
||||
|
||||
def _amp_to_db(x):
|
||||
|
|
|
@ -0,0 +1,125 @@
|
|||
from os import listdir, path
|
||||
import numpy as np
|
||||
import scipy, cv2, os, sys, argparse
|
||||
import json, subprocess, random, string
|
||||
from tqdm import tqdm
|
||||
from glob import glob
|
||||
import torch
|
||||
import pickle
|
||||
import face_detection
|
||||
|
||||
|
||||
parser = argparse.ArgumentParser(description='Inference code to lip-sync videos in the wild using Wav2Lip models')
|
||||
parser.add_argument('--img_size', default=96, type=int)
|
||||
parser.add_argument('--avatar_id', default='wav2lip_avatar1', type=str)
|
||||
parser.add_argument('--video_path', default='', type=str)
|
||||
parser.add_argument('--nosmooth', default=False, action='store_true',
|
||||
help='Prevent smoothing face detections over a short temporal window')
|
||||
parser.add_argument('--pads', nargs='+', type=int, default=[0, 10, 0, 0],
|
||||
help='Padding (top, bottom, left, right). Please adjust to include chin at least')
|
||||
parser.add_argument('--face_det_batch_size', type=int,
|
||||
help='Batch size for face detection', default=16)
|
||||
args = parser.parse_args()
|
||||
|
||||
device = 'cuda' if torch.cuda.is_available() else 'cpu'
|
||||
print('Using {} for inference.'.format(device))
|
||||
|
||||
def osmakedirs(path_list):
|
||||
for path in path_list:
|
||||
os.makedirs(path) if not os.path.exists(path) else None
|
||||
|
||||
def video2imgs(vid_path, save_path, ext = '.png',cut_frame = 10000000):
|
||||
cap = cv2.VideoCapture(vid_path)
|
||||
count = 0
|
||||
while True:
|
||||
if count > cut_frame:
|
||||
break
|
||||
ret, frame = cap.read()
|
||||
if ret:
|
||||
cv2.imwrite(f"{save_path}/{count:08d}.png", frame)
|
||||
count += 1
|
||||
else:
|
||||
break
|
||||
|
||||
def read_imgs(img_list):
|
||||
frames = []
|
||||
print('reading images...')
|
||||
for img_path in tqdm(img_list):
|
||||
frame = cv2.imread(img_path)
|
||||
frames.append(frame)
|
||||
return frames
|
||||
|
||||
def get_smoothened_boxes(boxes, T):
|
||||
for i in range(len(boxes)):
|
||||
if i + T > len(boxes):
|
||||
window = boxes[len(boxes) - T:]
|
||||
else:
|
||||
window = boxes[i : i + T]
|
||||
boxes[i] = np.mean(window, axis=0)
|
||||
return boxes
|
||||
|
||||
def face_detect(images):
|
||||
detector = face_detection.FaceAlignment(face_detection.LandmarksType._2D,
|
||||
flip_input=False, device=device)
|
||||
|
||||
batch_size = args.face_det_batch_size
|
||||
|
||||
while 1:
|
||||
predictions = []
|
||||
try:
|
||||
for i in tqdm(range(0, len(images), batch_size)):
|
||||
predictions.extend(detector.get_detections_for_batch(np.array(images[i:i + batch_size])))
|
||||
except RuntimeError:
|
||||
if batch_size == 1:
|
||||
raise RuntimeError('Image too big to run face detection on GPU. Please use the --resize_factor argument')
|
||||
batch_size //= 2
|
||||
print('Recovering from OOM error; New batch size: {}'.format(batch_size))
|
||||
continue
|
||||
break
|
||||
|
||||
results = []
|
||||
pady1, pady2, padx1, padx2 = args.pads
|
||||
for rect, image in zip(predictions, images):
|
||||
if rect is None:
|
||||
cv2.imwrite('temp/faulty_frame.jpg', image) # check this frame where the face was not detected.
|
||||
raise ValueError('Face not detected! Ensure the video contains a face in all the frames.')
|
||||
|
||||
y1 = max(0, rect[1] - pady1)
|
||||
y2 = min(image.shape[0], rect[3] + pady2)
|
||||
x1 = max(0, rect[0] - padx1)
|
||||
x2 = min(image.shape[1], rect[2] + padx2)
|
||||
|
||||
results.append([x1, y1, x2, y2])
|
||||
|
||||
boxes = np.array(results)
|
||||
if not args.nosmooth: boxes = get_smoothened_boxes(boxes, T=5)
|
||||
results = [[image[y1: y2, x1:x2], (y1, y2, x1, x2)] for image, (x1, y1, x2, y2) in zip(images, boxes)]
|
||||
|
||||
del detector
|
||||
return results
|
||||
|
||||
if __name__ == "__main__":
|
||||
avatar_path = f"./results/avatars/{args.avatar_id}"
|
||||
full_imgs_path = f"{avatar_path}/full_imgs"
|
||||
face_imgs_path = f"{avatar_path}/face_imgs"
|
||||
coords_path = f"{avatar_path}/coords.pkl"
|
||||
osmakedirs([avatar_path,full_imgs_path,face_imgs_path])
|
||||
print(args)
|
||||
|
||||
#if os.path.isfile(args.video_path):
|
||||
video2imgs(args.video_path, full_imgs_path, ext = 'png')
|
||||
input_img_list = sorted(glob(os.path.join(full_imgs_path, '*.[jpJP][pnPN]*[gG]')))
|
||||
|
||||
frames = read_imgs(input_img_list)
|
||||
face_det_results = face_detect(frames)
|
||||
coord_list = []
|
||||
idx = 0
|
||||
for frame,coords in face_det_results:
|
||||
#x1, y1, x2, y2 = bbox
|
||||
resized_crop_frame = cv2.resize(frame,(args.img_size, args.img_size)) #,interpolation = cv2.INTER_LANCZOS4)
|
||||
cv2.imwrite(f"{face_imgs_path}/{idx:08d}.png", resized_crop_frame)
|
||||
coord_list.append(coords)
|
||||
idx = idx + 1
|
||||
|
||||
with open(coords_path, 'wb') as f:
|
||||
pickle.dump(coord_list, f)
|
399
webrtc.py
399
webrtc.py
|
@ -1,194 +1,205 @@
|
|||
|
||||
import asyncio
|
||||
import json
|
||||
import logging
|
||||
import threading
|
||||
import time
|
||||
from typing import Tuple, Dict, Optional, Set, Union
|
||||
from av.frame import Frame
|
||||
from av.packet import Packet
|
||||
from av import AudioFrame
|
||||
import fractions
|
||||
import numpy as np
|
||||
|
||||
AUDIO_PTIME = 0.020 # 20ms audio packetization
|
||||
VIDEO_CLOCK_RATE = 90000
|
||||
VIDEO_PTIME = 1 / 25 # 30fps
|
||||
VIDEO_TIME_BASE = fractions.Fraction(1, VIDEO_CLOCK_RATE)
|
||||
SAMPLE_RATE = 16000
|
||||
AUDIO_TIME_BASE = fractions.Fraction(1, SAMPLE_RATE)
|
||||
|
||||
#from aiortc.contrib.media import MediaPlayer, MediaRelay
|
||||
#from aiortc.rtcrtpsender import RTCRtpSender
|
||||
from aiortc import (
|
||||
MediaStreamTrack,
|
||||
)
|
||||
|
||||
logging.basicConfig()
|
||||
logger = logging.getLogger(__name__)
|
||||
|
||||
|
||||
class PlayerStreamTrack(MediaStreamTrack):
|
||||
"""
|
||||
A video track that returns an animated flag.
|
||||
"""
|
||||
|
||||
def __init__(self, player, kind):
|
||||
super().__init__() # don't forget this!
|
||||
self.kind = kind
|
||||
self._player = player
|
||||
self._queue = asyncio.Queue()
|
||||
if self.kind == 'video':
|
||||
self.framecount = 0
|
||||
self.lasttime = time.perf_counter()
|
||||
self.totaltime = 0
|
||||
|
||||
_start: float
|
||||
_timestamp: int
|
||||
|
||||
async def next_timestamp(self) -> Tuple[int, fractions.Fraction]:
|
||||
if self.readyState != "live":
|
||||
raise Exception
|
||||
|
||||
if self.kind == 'video':
|
||||
if hasattr(self, "_timestamp"):
|
||||
# self._timestamp = (time.time()-self._start) * VIDEO_CLOCK_RATE
|
||||
self._timestamp += int(VIDEO_PTIME * VIDEO_CLOCK_RATE)
|
||||
wait = self._start + (self._timestamp / VIDEO_CLOCK_RATE) - time.time()
|
||||
if wait>0:
|
||||
await asyncio.sleep(wait)
|
||||
else:
|
||||
self._start = time.time()
|
||||
self._timestamp = 0
|
||||
print('video start:',self._start)
|
||||
return self._timestamp, VIDEO_TIME_BASE
|
||||
else: #audio
|
||||
if hasattr(self, "_timestamp"):
|
||||
# self._timestamp = (time.time()-self._start) * SAMPLE_RATE
|
||||
self._timestamp += int(AUDIO_PTIME * SAMPLE_RATE)
|
||||
wait = self._start + (self._timestamp / SAMPLE_RATE) - time.time()
|
||||
if wait>0:
|
||||
await asyncio.sleep(wait)
|
||||
else:
|
||||
self._start = time.time()
|
||||
self._timestamp = 0
|
||||
print('audio start:',self._start)
|
||||
return self._timestamp, AUDIO_TIME_BASE
|
||||
|
||||
async def recv(self) -> Union[Frame, Packet]:
|
||||
# frame = self.frames[self.counter % 30]
|
||||
self._player._start(self)
|
||||
# if self.kind == 'video':
|
||||
# frame = await self._queue.get()
|
||||
# else: #audio
|
||||
# if hasattr(self, "_timestamp"):
|
||||
# wait = self._start + self._timestamp / SAMPLE_RATE + AUDIO_PTIME - time.time()
|
||||
# if wait>0:
|
||||
# await asyncio.sleep(wait)
|
||||
# if self._queue.qsize()<1:
|
||||
# #frame = AudioFrame(format='s16', layout='mono', samples=320)
|
||||
# audio = np.zeros((1, 320), dtype=np.int16)
|
||||
# frame = AudioFrame.from_ndarray(audio, layout='mono', format='s16')
|
||||
# frame.sample_rate=16000
|
||||
# else:
|
||||
# frame = await self._queue.get()
|
||||
# else:
|
||||
# frame = await self._queue.get()
|
||||
frame = await self._queue.get()
|
||||
pts, time_base = await self.next_timestamp()
|
||||
frame.pts = pts
|
||||
frame.time_base = time_base
|
||||
if frame is None:
|
||||
self.stop()
|
||||
raise Exception
|
||||
if self.kind == 'video':
|
||||
self.totaltime += (time.perf_counter() - self.lasttime)
|
||||
self.framecount += 1
|
||||
self.lasttime = time.perf_counter()
|
||||
if self.framecount==100:
|
||||
print(f"------actual avg final fps:{self.framecount/self.totaltime:.4f}")
|
||||
self.framecount = 0
|
||||
self.totaltime=0
|
||||
return frame
|
||||
|
||||
def stop(self):
|
||||
super().stop()
|
||||
if self._player is not None:
|
||||
self._player._stop(self)
|
||||
self._player = None
|
||||
|
||||
def player_worker_thread(
|
||||
quit_event,
|
||||
loop,
|
||||
container,
|
||||
audio_track,
|
||||
video_track
|
||||
):
|
||||
container.render(quit_event,loop,audio_track,video_track)
|
||||
|
||||
class HumanPlayer:
|
||||
|
||||
def __init__(
|
||||
self, nerfreal, format=None, options=None, timeout=None, loop=False, decode=True
|
||||
):
|
||||
self.__thread: Optional[threading.Thread] = None
|
||||
self.__thread_quit: Optional[threading.Event] = None
|
||||
|
||||
# examine streams
|
||||
self.__started: Set[PlayerStreamTrack] = set()
|
||||
self.__audio: Optional[PlayerStreamTrack] = None
|
||||
self.__video: Optional[PlayerStreamTrack] = None
|
||||
|
||||
self.__audio = PlayerStreamTrack(self, kind="audio")
|
||||
self.__video = PlayerStreamTrack(self, kind="video")
|
||||
|
||||
self.__container = nerfreal
|
||||
|
||||
|
||||
@property
|
||||
def audio(self) -> MediaStreamTrack:
|
||||
"""
|
||||
A :class:`aiortc.MediaStreamTrack` instance if the file contains audio.
|
||||
"""
|
||||
return self.__audio
|
||||
|
||||
@property
|
||||
def video(self) -> MediaStreamTrack:
|
||||
"""
|
||||
A :class:`aiortc.MediaStreamTrack` instance if the file contains video.
|
||||
"""
|
||||
return self.__video
|
||||
|
||||
def _start(self, track: PlayerStreamTrack) -> None:
|
||||
self.__started.add(track)
|
||||
if self.__thread is None:
|
||||
self.__log_debug("Starting worker thread")
|
||||
self.__thread_quit = threading.Event()
|
||||
self.__thread = threading.Thread(
|
||||
name="media-player",
|
||||
target=player_worker_thread,
|
||||
args=(
|
||||
self.__thread_quit,
|
||||
asyncio.get_event_loop(),
|
||||
self.__container,
|
||||
self.__audio,
|
||||
self.__video
|
||||
),
|
||||
)
|
||||
self.__thread.start()
|
||||
|
||||
def _stop(self, track: PlayerStreamTrack) -> None:
|
||||
self.__started.discard(track)
|
||||
|
||||
if not self.__started and self.__thread is not None:
|
||||
self.__log_debug("Stopping worker thread")
|
||||
self.__thread_quit.set()
|
||||
self.__thread.join()
|
||||
self.__thread = None
|
||||
|
||||
if not self.__started and self.__container is not None:
|
||||
#self.__container.close()
|
||||
self.__container = None
|
||||
|
||||
def __log_debug(self, msg: str, *args) -> None:
|
||||
logger.debug(f"HumanPlayer {msg}", *args)
|
||||
|
||||
import asyncio
|
||||
import json
|
||||
import logging
|
||||
import threading
|
||||
import time
|
||||
from typing import Tuple, Dict, Optional, Set, Union
|
||||
from av.frame import Frame
|
||||
from av.packet import Packet
|
||||
from av import AudioFrame
|
||||
import fractions
|
||||
import numpy as np
|
||||
|
||||
AUDIO_PTIME = 0.020 # 20ms audio packetization
|
||||
VIDEO_CLOCK_RATE = 90000
|
||||
VIDEO_PTIME = 1 / 25 # 30fps
|
||||
VIDEO_TIME_BASE = fractions.Fraction(1, VIDEO_CLOCK_RATE)
|
||||
SAMPLE_RATE = 16000
|
||||
AUDIO_TIME_BASE = fractions.Fraction(1, SAMPLE_RATE)
|
||||
|
||||
#from aiortc.contrib.media import MediaPlayer, MediaRelay
|
||||
#from aiortc.rtcrtpsender import RTCRtpSender
|
||||
from aiortc import (
|
||||
MediaStreamTrack,
|
||||
)
|
||||
|
||||
logging.basicConfig()
|
||||
logger = logging.getLogger(__name__)
|
||||
|
||||
|
||||
class PlayerStreamTrack(MediaStreamTrack):
|
||||
"""
|
||||
A video track that returns an animated flag.
|
||||
"""
|
||||
|
||||
def __init__(self, player, kind):
|
||||
super().__init__() # don't forget this!
|
||||
self.kind = kind
|
||||
self._player = player
|
||||
self._queue = asyncio.Queue()
|
||||
self.timelist = [] #记录最近包的时间戳
|
||||
if self.kind == 'video':
|
||||
self.framecount = 0
|
||||
self.lasttime = time.perf_counter()
|
||||
self.totaltime = 0
|
||||
|
||||
_start: float
|
||||
_timestamp: int
|
||||
|
||||
async def next_timestamp(self) -> Tuple[int, fractions.Fraction]:
|
||||
if self.readyState != "live":
|
||||
raise Exception
|
||||
|
||||
if self.kind == 'video':
|
||||
if hasattr(self, "_timestamp"):
|
||||
#self._timestamp = (time.time()-self._start) * VIDEO_CLOCK_RATE
|
||||
self._timestamp += int(VIDEO_PTIME * VIDEO_CLOCK_RATE)
|
||||
# wait = self._start + (self._timestamp / VIDEO_CLOCK_RATE) - time.time()
|
||||
wait = self.timelist[0] + len(self.timelist)*VIDEO_PTIME - time.time()
|
||||
if wait>0:
|
||||
await asyncio.sleep(wait)
|
||||
self.timelist.append(time.time())
|
||||
if len(self.timelist)>100:
|
||||
self.timelist.pop(0)
|
||||
else:
|
||||
self._start = time.time()
|
||||
self._timestamp = 0
|
||||
self.timelist.append(self._start)
|
||||
print('video start:',self._start)
|
||||
return self._timestamp, VIDEO_TIME_BASE
|
||||
else: #audio
|
||||
if hasattr(self, "_timestamp"):
|
||||
#self._timestamp = (time.time()-self._start) * SAMPLE_RATE
|
||||
self._timestamp += int(AUDIO_PTIME * SAMPLE_RATE)
|
||||
# wait = self._start + (self._timestamp / SAMPLE_RATE) - time.time()
|
||||
wait = self.timelist[0] + len(self.timelist)*AUDIO_PTIME - time.time()
|
||||
if wait>0:
|
||||
await asyncio.sleep(wait)
|
||||
self.timelist.append(time.time())
|
||||
if len(self.timelist)>200:
|
||||
self.timelist.pop(0)
|
||||
else:
|
||||
self._start = time.time()
|
||||
self._timestamp = 0
|
||||
self.timelist.append(self._start)
|
||||
print('audio start:',self._start)
|
||||
return self._timestamp, AUDIO_TIME_BASE
|
||||
|
||||
async def recv(self) -> Union[Frame, Packet]:
|
||||
# frame = self.frames[self.counter % 30]
|
||||
self._player._start(self)
|
||||
# if self.kind == 'video':
|
||||
# frame = await self._queue.get()
|
||||
# else: #audio
|
||||
# if hasattr(self, "_timestamp"):
|
||||
# wait = self._start + self._timestamp / SAMPLE_RATE + AUDIO_PTIME - time.time()
|
||||
# if wait>0:
|
||||
# await asyncio.sleep(wait)
|
||||
# if self._queue.qsize()<1:
|
||||
# #frame = AudioFrame(format='s16', layout='mono', samples=320)
|
||||
# audio = np.zeros((1, 320), dtype=np.int16)
|
||||
# frame = AudioFrame.from_ndarray(audio, layout='mono', format='s16')
|
||||
# frame.sample_rate=16000
|
||||
# else:
|
||||
# frame = await self._queue.get()
|
||||
# else:
|
||||
# frame = await self._queue.get()
|
||||
frame = await self._queue.get()
|
||||
pts, time_base = await self.next_timestamp()
|
||||
frame.pts = pts
|
||||
frame.time_base = time_base
|
||||
if frame is None:
|
||||
self.stop()
|
||||
raise Exception
|
||||
if self.kind == 'video':
|
||||
self.totaltime += (time.perf_counter() - self.lasttime)
|
||||
self.framecount += 1
|
||||
self.lasttime = time.perf_counter()
|
||||
if self.framecount==100:
|
||||
print(f"------actual avg final fps:{self.framecount/self.totaltime:.4f}")
|
||||
self.framecount = 0
|
||||
self.totaltime=0
|
||||
return frame
|
||||
|
||||
def stop(self):
|
||||
super().stop()
|
||||
if self._player is not None:
|
||||
self._player._stop(self)
|
||||
self._player = None
|
||||
|
||||
def player_worker_thread(
|
||||
quit_event,
|
||||
loop,
|
||||
container,
|
||||
audio_track,
|
||||
video_track
|
||||
):
|
||||
container.render(quit_event,loop,audio_track,video_track)
|
||||
|
||||
class HumanPlayer:
|
||||
|
||||
def __init__(
|
||||
self, nerfreal, format=None, options=None, timeout=None, loop=False, decode=True
|
||||
):
|
||||
self.__thread: Optional[threading.Thread] = None
|
||||
self.__thread_quit: Optional[threading.Event] = None
|
||||
|
||||
# examine streams
|
||||
self.__started: Set[PlayerStreamTrack] = set()
|
||||
self.__audio: Optional[PlayerStreamTrack] = None
|
||||
self.__video: Optional[PlayerStreamTrack] = None
|
||||
|
||||
self.__audio = PlayerStreamTrack(self, kind="audio")
|
||||
self.__video = PlayerStreamTrack(self, kind="video")
|
||||
|
||||
self.__container = nerfreal
|
||||
|
||||
|
||||
@property
|
||||
def audio(self) -> MediaStreamTrack:
|
||||
"""
|
||||
A :class:`aiortc.MediaStreamTrack` instance if the file contains audio.
|
||||
"""
|
||||
return self.__audio
|
||||
|
||||
@property
|
||||
def video(self) -> MediaStreamTrack:
|
||||
"""
|
||||
A :class:`aiortc.MediaStreamTrack` instance if the file contains video.
|
||||
"""
|
||||
return self.__video
|
||||
|
||||
def _start(self, track: PlayerStreamTrack) -> None:
|
||||
self.__started.add(track)
|
||||
if self.__thread is None:
|
||||
self.__log_debug("Starting worker thread")
|
||||
self.__thread_quit = threading.Event()
|
||||
self.__thread = threading.Thread(
|
||||
name="media-player",
|
||||
target=player_worker_thread,
|
||||
args=(
|
||||
self.__thread_quit,
|
||||
asyncio.get_event_loop(),
|
||||
self.__container,
|
||||
self.__audio,
|
||||
self.__video
|
||||
),
|
||||
)
|
||||
self.__thread.start()
|
||||
|
||||
def _stop(self, track: PlayerStreamTrack) -> None:
|
||||
self.__started.discard(track)
|
||||
|
||||
if not self.__started and self.__thread is not None:
|
||||
self.__log_debug("Stopping worker thread")
|
||||
self.__thread_quit.set()
|
||||
self.__thread.join()
|
||||
self.__thread = None
|
||||
|
||||
if not self.__started and self.__container is not None:
|
||||
#self.__container.close()
|
||||
self.__container = None
|
||||
|
||||
def __log_debug(self, msg: str, *args) -> None:
|
||||
logger.debug(f"HumanPlayer {msg}", *args)
|
||||
|
|
Loading…
Reference in New Issue