Compare commits
110 Commits
Author | SHA1 | Date |
---|---|---|
|
14208c6d60 | |
|
959ecf9be8 | |
|
5e8884fcf3 | |
|
00dbc71db9 | |
|
a8b40fa813 | |
|
8d5a38222b | |
|
5340e77e76 | |
|
f584cb25d1 | |
|
275af1ed9e | |
|
995428b426 | |
|
baf8270fc5 | |
|
e9faa50b9e | |
|
93a6513504 | |
|
93f3ed9895 | |
|
9c8f020b3f | |
|
3e60fd7738 | |
|
a9e9cfb220 | |
|
391512f68c | |
|
0c63e9a11b | |
|
2883b2243e | |
|
0465437abc | |
|
7917c5f7cc | |
|
4f14468e19 | |
|
1d5c7e1542 | |
|
79df82ebea | |
|
cd7d5f31b5 | |
|
c812e45f35 | |
|
9fe4c7fccf | |
|
18d7db35a7 | |
|
6eb03ecbff | |
|
98eeeb17af | |
|
994535fe3e | |
|
da9ffa9521 | |
|
c0682408c5 | |
|
5da818b9d9 | |
|
592312ab8c | |
|
39d7aff90a | |
|
6fb8a19fd5 | |
|
58e763fdb6 | |
|
d01860176e | |
|
016442272e | |
|
ff0e11866d | |
|
632409da1e | |
|
4e355e9ab9 | |
|
af1ad0aed8 | |
|
677227145e | |
|
dc94e87620 | |
|
dce3085231 | |
|
c2ce2e25a4 | |
|
78324506fb | |
|
d384aaaa1c | |
|
f1d6821d62 | |
|
8dd3441fcd | |
|
b902d3244c | |
|
1fa1620c5e | |
|
a1ae58ffa7 | |
|
bf4e4b0251 | |
|
6508a9160c | |
|
5a4a459ad5 | |
|
6294f64795 | |
|
55adec9f3f | |
|
14b7772475 | |
|
3e702b8a12 | |
|
8c012c5ab8 | |
|
6a1f2e4f48 | |
|
6978f89ec2 | |
|
71009f9f28 | |
|
4137e5bce6 | |
|
027e15201a | |
|
f2d81f88d3 | |
|
995dff00df | |
|
2e64be4b5d | |
|
6d4952c1bf | |
|
a3a86bf299 | |
|
b9d77f9fb5 | |
|
1e52055d65 | |
|
d52ea3133a | |
|
2389c97fb4 | |
|
3674875095 | |
|
54fcbb8cc7 | |
|
847fd202df | |
|
fafa862ba1 | |
|
91e73839f3 | |
|
c2e043bade | |
|
ec7f7b5041 | |
|
2c5c356ca0 | |
|
50a1dc0f34 | |
|
91c0768fe9 | |
|
f0e6d7c5bf | |
|
0cf9f4902d | |
|
8f715d2477 | |
|
835a986315 | |
|
fce3ab57ac | |
|
d7c837ba32 | |
|
1ebd0c90b6 | |
|
f5640ef197 | |
|
86cdb40906 | |
|
fa460ce101 | |
|
313d57dfa4 | |
|
9cdd6fcadf | |
|
fa08d4f670 | |
|
f83d29620b | |
|
d5c8b240cc | |
|
fe963ed543 | |
|
607be33781 | |
|
4fc13ed714 | |
|
9c8d0b05dc | |
|
405b331bdc | |
|
1c8f9338bb | |
|
d8f24e7b96 |
|
@ -15,3 +15,4 @@ pretrained
|
||||||
*.mp4
|
*.mp4
|
||||||
.DS_Store
|
.DS_Store
|
||||||
workspace/log_ngp.txt
|
workspace/log_ngp.txt
|
||||||
|
.idea
|
214
LICENSE
214
LICENSE
|
@ -1,21 +1,201 @@
|
||||||
MIT License
|
Apache License
|
||||||
|
Version 2.0, January 2004
|
||||||
|
http://www.apache.org/licenses/
|
||||||
|
|
||||||
Copyright (c) 2023 LiHengzhong
|
TERMS AND CONDITIONS FOR USE, REPRODUCTION, AND DISTRIBUTION
|
||||||
|
|
||||||
Permission is hereby granted, free of charge, to any person obtaining a copy
|
1. Definitions.
|
||||||
of this software and associated documentation files (the "Software"), to deal
|
|
||||||
in the Software without restriction, including without limitation the rights
|
|
||||||
to use, copy, modify, merge, publish, distribute, sublicense, and/or sell
|
|
||||||
copies of the Software, and to permit persons to whom the Software is
|
|
||||||
furnished to do so, subject to the following conditions:
|
|
||||||
|
|
||||||
The above copyright notice and this permission notice shall be included in all
|
"License" shall mean the terms and conditions for use, reproduction,
|
||||||
copies or substantial portions of the Software.
|
and distribution as defined by Sections 1 through 9 of this document.
|
||||||
|
|
||||||
THE SOFTWARE IS PROVIDED "AS IS", WITHOUT WARRANTY OF ANY KIND, EXPRESS OR
|
"Licensor" shall mean the copyright owner or entity authorized by
|
||||||
IMPLIED, INCLUDING BUT NOT LIMITED TO THE WARRANTIES OF MERCHANTABILITY,
|
the copyright owner that is granting the License.
|
||||||
FITNESS FOR A PARTICULAR PURPOSE AND NONINFRINGEMENT. IN NO EVENT SHALL THE
|
|
||||||
AUTHORS OR COPYRIGHT HOLDERS BE LIABLE FOR ANY CLAIM, DAMAGES OR OTHER
|
"Legal Entity" shall mean the union of the acting entity and all
|
||||||
LIABILITY, WHETHER IN AN ACTION OF CONTRACT, TORT OR OTHERWISE, ARISING FROM,
|
other entities that control, are controlled by, or are under common
|
||||||
OUT OF OR IN CONNECTION WITH THE SOFTWARE OR THE USE OR OTHER DEALINGS IN THE
|
control with that entity. For the purposes of this definition,
|
||||||
SOFTWARE.
|
"control" means (i) the power, direct or indirect, to cause the
|
||||||
|
direction or management of such entity, whether by contract or
|
||||||
|
otherwise, or (ii) ownership of fifty percent (50%) or more of the
|
||||||
|
outstanding shares, or (iii) beneficial ownership of such entity.
|
||||||
|
|
||||||
|
"You" (or "Your") shall mean an individual or Legal Entity
|
||||||
|
exercising permissions granted by this License.
|
||||||
|
|
||||||
|
"Source" form shall mean the preferred form for making modifications,
|
||||||
|
including but not limited to software source code, documentation
|
||||||
|
source, and configuration files.
|
||||||
|
|
||||||
|
"Object" form shall mean any form resulting from mechanical
|
||||||
|
transformation or translation of a Source form, including but
|
||||||
|
not limited to compiled object code, generated documentation,
|
||||||
|
and conversions to other media types.
|
||||||
|
|
||||||
|
"Work" shall mean the work of authorship, whether in Source or
|
||||||
|
Object form, made available under the License, as indicated by a
|
||||||
|
copyright notice that is included in or attached to the work
|
||||||
|
(an example is provided in the Appendix below).
|
||||||
|
|
||||||
|
"Derivative Works" shall mean any work, whether in Source or Object
|
||||||
|
form, that is based on (or derived from) the Work and for which the
|
||||||
|
editorial revisions, annotations, elaborations, or other modifications
|
||||||
|
represent, as a whole, an original work of authorship. For the purposes
|
||||||
|
of this License, Derivative Works shall not include works that remain
|
||||||
|
separable from, or merely link (or bind by name) to the interfaces of,
|
||||||
|
the Work and Derivative Works thereof.
|
||||||
|
|
||||||
|
"Contribution" shall mean any work of authorship, including
|
||||||
|
the original version of the Work and any modifications or additions
|
||||||
|
to that Work or Derivative Works thereof, that is intentionally
|
||||||
|
submitted to Licensor for inclusion in the Work by the copyright owner
|
||||||
|
or by an individual or Legal Entity authorized to submit on behalf of
|
||||||
|
the copyright owner. For the purposes of this definition, "submitted"
|
||||||
|
means any form of electronic, verbal, or written communication sent
|
||||||
|
to the Licensor or its representatives, including but not limited to
|
||||||
|
communication on electronic mailing lists, source code control systems,
|
||||||
|
and issue tracking systems that are managed by, or on behalf of, the
|
||||||
|
Licensor for the purpose of discussing and improving the Work, but
|
||||||
|
excluding communication that is conspicuously marked or otherwise
|
||||||
|
designated in writing by the copyright owner as "Not a Contribution."
|
||||||
|
|
||||||
|
"Contributor" shall mean Licensor and any individual or Legal Entity
|
||||||
|
on behalf of whom a Contribution has been received by Licensor and
|
||||||
|
subsequently incorporated within the Work.
|
||||||
|
|
||||||
|
2. Grant of Copyright License. Subject to the terms and conditions of
|
||||||
|
this License, each Contributor hereby grants to You a perpetual,
|
||||||
|
worldwide, non-exclusive, no-charge, royalty-free, irrevocable
|
||||||
|
copyright license to reproduce, prepare Derivative Works of,
|
||||||
|
publicly display, publicly perform, sublicense, and distribute the
|
||||||
|
Work and such Derivative Works in Source or Object form.
|
||||||
|
|
||||||
|
3. Grant of Patent License. Subject to the terms and conditions of
|
||||||
|
this License, each Contributor hereby grants to You a perpetual,
|
||||||
|
worldwide, non-exclusive, no-charge, royalty-free, irrevocable
|
||||||
|
(except as stated in this section) patent license to make, have made,
|
||||||
|
use, offer to sell, sell, import, and otherwise transfer the Work,
|
||||||
|
where such license applies only to those patent claims licensable
|
||||||
|
by such Contributor that are necessarily infringed by their
|
||||||
|
Contribution(s) alone or by combination of their Contribution(s)
|
||||||
|
with the Work to which such Contribution(s) was submitted. If You
|
||||||
|
institute patent litigation against any entity (including a
|
||||||
|
cross-claim or counterclaim in a lawsuit) alleging that the Work
|
||||||
|
or a Contribution incorporated within the Work constitutes direct
|
||||||
|
or contributory patent infringement, then any patent licenses
|
||||||
|
granted to You under this License for that Work shall terminate
|
||||||
|
as of the date such litigation is filed.
|
||||||
|
|
||||||
|
4. Redistribution. You may reproduce and distribute copies of the
|
||||||
|
Work or Derivative Works thereof in any medium, with or without
|
||||||
|
modifications, and in Source or Object form, provided that You
|
||||||
|
meet the following conditions:
|
||||||
|
|
||||||
|
(a) You must give any other recipients of the Work or
|
||||||
|
Derivative Works a copy of this License; and
|
||||||
|
|
||||||
|
(b) You must cause any modified files to carry prominent notices
|
||||||
|
stating that You changed the files; and
|
||||||
|
|
||||||
|
(c) You must retain, in the Source form of any Derivative Works
|
||||||
|
that You distribute, all copyright, patent, trademark, and
|
||||||
|
attribution notices from the Source form of the Work,
|
||||||
|
excluding those notices that do not pertain to any part of
|
||||||
|
the Derivative Works; and
|
||||||
|
|
||||||
|
(d) If the Work includes a "NOTICE" text file as part of its
|
||||||
|
distribution, then any Derivative Works that You distribute must
|
||||||
|
include a readable copy of the attribution notices contained
|
||||||
|
within such NOTICE file, excluding those notices that do not
|
||||||
|
pertain to any part of the Derivative Works, in at least one
|
||||||
|
of the following places: within a NOTICE text file distributed
|
||||||
|
as part of the Derivative Works; within the Source form or
|
||||||
|
documentation, if provided along with the Derivative Works; or,
|
||||||
|
within a display generated by the Derivative Works, if and
|
||||||
|
wherever such third-party notices normally appear. The contents
|
||||||
|
of the NOTICE file are for informational purposes only and
|
||||||
|
do not modify the License. You may add Your own attribution
|
||||||
|
notices within Derivative Works that You distribute, alongside
|
||||||
|
or as an addendum to the NOTICE text from the Work, provided
|
||||||
|
that such additional attribution notices cannot be construed
|
||||||
|
as modifying the License.
|
||||||
|
|
||||||
|
You may add Your own copyright statement to Your modifications and
|
||||||
|
may provide additional or different license terms and conditions
|
||||||
|
for use, reproduction, or distribution of Your modifications, or
|
||||||
|
for any such Derivative Works as a whole, provided Your use,
|
||||||
|
reproduction, and distribution of the Work otherwise complies with
|
||||||
|
the conditions stated in this License.
|
||||||
|
|
||||||
|
5. Submission of Contributions. Unless You explicitly state otherwise,
|
||||||
|
any Contribution intentionally submitted for inclusion in the Work
|
||||||
|
by You to the Licensor shall be under the terms and conditions of
|
||||||
|
this License, without any additional terms or conditions.
|
||||||
|
Notwithstanding the above, nothing herein shall supersede or modify
|
||||||
|
the terms of any separate license agreement you may have executed
|
||||||
|
with Licensor regarding such Contributions.
|
||||||
|
|
||||||
|
6. Trademarks. This License does not grant permission to use the trade
|
||||||
|
names, trademarks, service marks, or product names of the Licensor,
|
||||||
|
except as required for reasonable and customary use in describing the
|
||||||
|
origin of the Work and reproducing the content of the NOTICE file.
|
||||||
|
|
||||||
|
7. Disclaimer of Warranty. Unless required by applicable law or
|
||||||
|
agreed to in writing, Licensor provides the Work (and each
|
||||||
|
Contributor provides its Contributions) on an "AS IS" BASIS,
|
||||||
|
WITHOUT WARRANTIES OR CONDITIONS OF ANY KIND, either express or
|
||||||
|
implied, including, without limitation, any warranties or conditions
|
||||||
|
of TITLE, NON-INFRINGEMENT, MERCHANTABILITY, or FITNESS FOR A
|
||||||
|
PARTICULAR PURPOSE. You are solely responsible for determining the
|
||||||
|
appropriateness of using or redistributing the Work and assume any
|
||||||
|
risks associated with Your exercise of permissions under this License.
|
||||||
|
|
||||||
|
8. Limitation of Liability. In no event and under no legal theory,
|
||||||
|
whether in tort (including negligence), contract, or otherwise,
|
||||||
|
unless required by applicable law (such as deliberate and grossly
|
||||||
|
negligent acts) or agreed to in writing, shall any Contributor be
|
||||||
|
liable to You for damages, including any direct, indirect, special,
|
||||||
|
incidental, or consequential damages of any character arising as a
|
||||||
|
result of this License or out of the use or inability to use the
|
||||||
|
Work (including but not limited to damages for loss of goodwill,
|
||||||
|
work stoppage, computer failure or malfunction, or any and all
|
||||||
|
other commercial damages or losses), even if such Contributor
|
||||||
|
has been advised of the possibility of such damages.
|
||||||
|
|
||||||
|
9. Accepting Warranty or Additional Liability. While redistributing
|
||||||
|
the Work or Derivative Works thereof, You may choose to offer,
|
||||||
|
and charge a fee for, acceptance of support, warranty, indemnity,
|
||||||
|
or other liability obligations and/or rights consistent with this
|
||||||
|
License. However, in accepting such obligations, You may act only
|
||||||
|
on Your own behalf and on Your sole responsibility, not on behalf
|
||||||
|
of any other Contributor, and only if You agree to indemnify,
|
||||||
|
defend, and hold each Contributor harmless for any liability
|
||||||
|
incurred by, or claims asserted against, such Contributor by reason
|
||||||
|
of your accepting any such warranty or additional liability.
|
||||||
|
|
||||||
|
END OF TERMS AND CONDITIONS
|
||||||
|
|
||||||
|
APPENDIX: How to apply the Apache License to your work.
|
||||||
|
|
||||||
|
To apply the Apache License to your work, attach the following
|
||||||
|
boilerplate notice, with the fields enclosed by brackets "[]"
|
||||||
|
replaced with your own identifying information. (Don't include
|
||||||
|
the brackets!) The text should be enclosed in the appropriate
|
||||||
|
comment syntax for the file format. We also recommend that a
|
||||||
|
file or class name and description of purpose be included on the
|
||||||
|
same "printed page" as the copyright notice for easier
|
||||||
|
identification within third-party archives.
|
||||||
|
|
||||||
|
Copyright [yyyy] [name of copyright owner]
|
||||||
|
|
||||||
|
Licensed under the Apache License, Version 2.0 (the "License");
|
||||||
|
you may not use this file except in compliance with the License.
|
||||||
|
You may obtain a copy of the License at
|
||||||
|
|
||||||
|
http://www.apache.org/licenses/LICENSE-2.0
|
||||||
|
|
||||||
|
Unless required by applicable law or agreed to in writing, software
|
||||||
|
distributed under the License is distributed on an "AS IS" BASIS,
|
||||||
|
WITHOUT WARRANTIES OR CONDITIONS OF ANY KIND, either express or implied.
|
||||||
|
See the License for the specific language governing permissions and
|
||||||
|
limitations under the License.
|
||||||
|
|
152
README.md
152
README.md
|
@ -1,13 +1,17 @@
|
||||||
A streaming digital human based on the Ernerf model, realize audio video synchronous dialogue. It can basically achieve commercial effects.
|
Real time interactive streaming digital human, realize audio video synchronous dialogue. It can basically achieve commercial effects.
|
||||||
基于ernerf模型的流式数字人,实现音视频同步对话。基本可以达到商用效果
|
实时交互流式数字人,实现音视频同步对话。基本可以达到商用效果
|
||||||
|
|
||||||
[![Watch the video]](/assets/demo.mp4)
|
[ernerf效果](https://www.bilibili.com/video/BV1PM4m1y7Q2/) [musetalk效果](https://www.bilibili.com/video/BV1gm421N7vQ/) [wav2lip效果](https://www.bilibili.com/video/BV1Bw4m1e74P/)
|
||||||
|
|
||||||
|
## 为避免与3d数字人混淆,原项目metahuman-stream改名为livetalking,原有链接地址继续可用
|
||||||
|
|
||||||
## Features
|
## Features
|
||||||
1. 支持声音克隆
|
1. 支持多种数字人模型: ernerf、musetalk、wav2lip
|
||||||
2. 支持大模型对话
|
2. 支持声音克隆
|
||||||
3. 支持多种音频特征驱动:wav2vec、hubert
|
3. 支持数字人说话被打断
|
||||||
4. 支持全身视频拼接
|
4. 支持全身视频拼接
|
||||||
|
5. 支持rtmp和webrtc
|
||||||
|
6. 支持视频编排:不说话时播放自定义视频
|
||||||
|
|
||||||
## 1. Installation
|
## 1. Installation
|
||||||
|
|
||||||
|
@ -17,23 +21,28 @@ Tested on Ubuntu 20.04, Python3.10, Pytorch 1.12 and CUDA 11.3
|
||||||
|
|
||||||
```bash
|
```bash
|
||||||
conda create -n nerfstream python=3.10
|
conda create -n nerfstream python=3.10
|
||||||
conda install pytorch==1.12.1 torchvision==0.13.1 cudatoolkit=11.3 -c pytorch
|
|
||||||
conda activate nerfstream
|
conda activate nerfstream
|
||||||
|
conda install pytorch==1.12.1 torchvision==0.13.1 cudatoolkit=11.3 -c pytorch
|
||||||
pip install -r requirements.txt
|
pip install -r requirements.txt
|
||||||
|
#如果不训练ernerf模型,不需要安装下面的库
|
||||||
pip install "git+https://github.com/facebookresearch/pytorch3d.git"
|
pip install "git+https://github.com/facebookresearch/pytorch3d.git"
|
||||||
pip install tensorflow-gpu==2.8.0
|
pip install tensorflow-gpu==2.8.0
|
||||||
|
pip install --upgrade "protobuf<=3.20.1"
|
||||||
```
|
```
|
||||||
|
如果用pytorch2.1,torchvision用0.16(可以去torchvision官网根据pytorch版本找匹配的),cudatoolkit可以不用装
|
||||||
|
安装常见问题[FAQ](/assets/faq.md)
|
||||||
linux cuda环境搭建可以参考这篇文章 https://zhuanlan.zhihu.com/p/674972886
|
linux cuda环境搭建可以参考这篇文章 https://zhuanlan.zhihu.com/p/674972886
|
||||||
|
|
||||||
### 1.2 安装rtmpstream库
|
|
||||||
参照 https://github.com/lipku/python_rtmpstream
|
|
||||||
|
|
||||||
|
## 2. Quick Start
|
||||||
## 2. Run
|
默认采用ernerf模型,webrtc推流到srs
|
||||||
|
### 2.1 运行srs
|
||||||
### 2.1 运行rtmpserver (srs)
|
|
||||||
```
|
```
|
||||||
docker run --rm -it -p 1935:1935 -p 1985:1985 -p 8080:8080 registry.cn-hangzhou.aliyuncs.com/ossrs/srs:5
|
export CANDIDATE='<服务器外网ip>'
|
||||||
|
docker run --rm --env CANDIDATE=$CANDIDATE \
|
||||||
|
-p 1935:1935 -p 8080:8080 -p 1985:1985 -p 8000:8000/udp \
|
||||||
|
registry.cn-hangzhou.aliyuncs.com/ossrs/srs:5 \
|
||||||
|
objs/srs -c conf/rtc.conf
|
||||||
```
|
```
|
||||||
|
|
||||||
### 2.2 启动数字人:
|
### 2.2 启动数字人:
|
||||||
|
@ -47,106 +56,45 @@ python app.py
|
||||||
export HF_ENDPOINT=https://hf-mirror.com
|
export HF_ENDPOINT=https://hf-mirror.com
|
||||||
```
|
```
|
||||||
|
|
||||||
运行成功后,用vlc访问rtmp://serverip/live/livestream
|
用浏览器打开http://serverip:8010/rtcpushapi.html, 在文本框输入任意文字,提交。数字人播报该段文字
|
||||||
|
备注:服务端需要开放端口 tcp:8000,8010,1985; udp:8000
|
||||||
### 2.3 网页端数字人播报输入文字
|
|
||||||
安装并启动nginx
|
|
||||||
```
|
|
||||||
apt install nginx
|
|
||||||
nginx
|
|
||||||
```
|
|
||||||
将echo.html和mpegts-1.7.3.min.js拷到/var/www/html下
|
|
||||||
|
|
||||||
用浏览器打开http://serverip/echo.html, 在文本框输入任意文字,提交。数字人播报该段文字
|
|
||||||
|
|
||||||
## 3. More Usage
|
## 3. More Usage
|
||||||
### 3.1 使用LLM模型进行数字人对话
|
使用说明: <https://livetalking-doc.readthedocs.io/>
|
||||||
|
|
||||||
目前借鉴数字人对话系统[LinlyTalker](https://github.com/Kedreamix/Linly-Talker)的方式,LLM模型支持Chatgpt,Qwen和GeminiPro。需要在app.py中填入自己的api_key。
|
|
||||||
安装并启动nginx,将chat.html和mpegts-1.7.3.min.js拷到/var/www/html下
|
|
||||||
|
|
||||||
用浏览器打开http://serverip/chat.html
|
|
||||||
|
|
||||||
### 3.2 使用本地tts服务,支持声音克隆
|
|
||||||
运行xtts服务,参照 https://github.com/coqui-ai/xtts-streaming-server
|
|
||||||
```
|
|
||||||
docker run --gpus=all -e COQUI_TOS_AGREED=1 --rm -p 9000:80 ghcr.io/coqui-ai/xtts-streaming-server:latest
|
|
||||||
```
|
|
||||||
然后运行,其中ref.wav为需要克隆的声音文件
|
|
||||||
```
|
|
||||||
python app.py --tts xtts --ref_file data/ref.wav
|
|
||||||
```
|
|
||||||
|
|
||||||
### 3.3 音频特征用hubert
|
|
||||||
如果训练模型时用的hubert提取音频特征,用如下命令启动数字人
|
|
||||||
```
|
|
||||||
python app.py --asr_model facebook/hubert-large-ls960-ft
|
|
||||||
```
|
|
||||||
|
|
||||||
### 3.4 设置背景图片
|
|
||||||
```
|
|
||||||
python app.py --bg_img bg.jpg
|
|
||||||
```
|
|
||||||
|
|
||||||
### 3.5 全身视频拼接
|
|
||||||
#### 3.5.1 切割训练用的视频
|
|
||||||
```
|
|
||||||
ffmpeg -i fullbody.mp4 -vf crop="400:400:100:5" train.mp4
|
|
||||||
```
|
|
||||||
用train.mp4训练模型
|
|
||||||
#### 3.5.2 提取全身图片
|
|
||||||
```
|
|
||||||
ffmpeg -i fullbody.mp4 -vf fps=25 -qmin 1 -q:v 1 -start_number 0 data/fullbody/img/%d.jpg
|
|
||||||
```
|
|
||||||
#### 3.5.2 启动数字人
|
|
||||||
```
|
|
||||||
python app.py --fullbody --fullbody_img data/fullbody/img --fullbody_offset_x 100 --fullbody_offset_y 5 --fullbody_width 580 --fullbody_height 1080 --W 400 --H 400
|
|
||||||
```
|
|
||||||
- --fullbody_width、--fullbody_height 全身视频的宽、高
|
|
||||||
- --W、--H 训练视频的宽、高
|
|
||||||
- ernerf训练第三步torso如果训练的不好,在拼接处会有接缝。可以在上面的命令加上--torso_imgs data/xxx/torso_imgs,torso不用模型推理,直接用训练数据集里的torso图片。这种方式可能头颈处会有些人工痕迹。
|
|
||||||
|
|
||||||
## 4. Docker Run
|
## 4. Docker Run
|
||||||
不需要第1步的安装,直接运行。
|
不需要前面的安装,直接运行。
|
||||||
```
|
```
|
||||||
docker run --gpus all -it --network=host --rm registry.cn-hangzhou.aliyuncs.com/lipku/nerfstream:v1.3
|
docker run --gpus all -it --network=host --rm registry.cn-beijing.aliyuncs.com/codewithgpu2/lipku-metahuman-stream:vjo1Y6NJ3N
|
||||||
```
|
```
|
||||||
srs和nginx的运行同2.1和2.3
|
代码在/root/metahuman-stream,先git pull拉一下最新代码,然后执行命令同第2、3步
|
||||||
|
|
||||||
## 5. Data flow
|
提供如下镜像
|
||||||

|
- autodl镜像: <https://www.codewithgpu.com/i/lipku/metahuman-stream/base>
|
||||||
|
[autodl教程](autodl/README.md)
|
||||||
|
|
||||||
## 6. 数字人模型文件
|
|
||||||
可以替换成自己训练的模型(https://github.com/Fictionarry/ER-NeRF)
|
|
||||||
```python
|
|
||||||
.
|
|
||||||
├── data
|
|
||||||
│ ├── data_kf.json
|
|
||||||
│ ├── au.csv
|
|
||||||
│ ├── pretrained
|
|
||||||
│ └── └── ngp_kf.pth
|
|
||||||
|
|
||||||
```
|
## 5. 性能分析
|
||||||
|
|
||||||
## 7. 性能分析
|
|
||||||
1. 帧率
|
1. 帧率
|
||||||
在Tesla T4显卡上测试整体fps为18左右,如果去掉音视频编码推流,帧率在20左右。用4090显卡可以达到40多帧/秒。
|
在Tesla T4显卡上测试整体fps为18左右,如果去掉音视频编码推流,帧率在20左右。用4090显卡可以达到40多帧/秒。
|
||||||
优化:新开一个线程运行音视频编码推流
|
|
||||||
2. 延时
|
2. 延时
|
||||||
整体延时5s多
|
整体延时3s左右
|
||||||
(1)tts延时2s左右,目前用的edgetts,需要将每句话转完后一次性输入,可以优化tts改成流式输入
|
(1)tts延时1.7s左右,目前用的edgetts,需要将每句话转完后一次性输入,可以优化tts改成流式输入
|
||||||
(2)wav2vec延时1s多,需要缓存50帧音频做计算,可以通过-m设置context_size来减少延时
|
(2)wav2vec延时0.4s,需要缓存18帧音频做计算
|
||||||
(3)srs转发延时,设置srs服务器减少缓冲延时。具体配置可看 https://ossrs.net/lts/zh-cn/docs/v5/doc/low-latency, 配置了一个低延时版本
|
(3)srs转发延时,设置srs服务器减少缓冲延时。具体配置可看 https://ossrs.net/lts/zh-cn/docs/v5/doc/low-latency
|
||||||
```python
|
|
||||||
docker run --rm -it -p 1935:1935 -p 1985:1985 -p 8080:8080 registry.cn-hangzhou.aliyuncs.com/lipku/srs:v1.1
|
|
||||||
```
|
|
||||||
|
|
||||||
## 8. TODO
|
|
||||||
|
## 6. TODO
|
||||||
- [x] 添加chatgpt实现数字人对话
|
- [x] 添加chatgpt实现数字人对话
|
||||||
- [x] 声音克隆
|
- [x] 声音克隆
|
||||||
- [ ] 数字人静音时用一段视频代替
|
- [x] 数字人静音时用一段视频代替
|
||||||
|
- [x] MuseTalk
|
||||||
|
- [x] Wav2Lip
|
||||||
|
- [ ] TalkingGaussian
|
||||||
|
|
||||||
|
---
|
||||||
|
如果本项目对你有帮助,帮忙点个star。也欢迎感兴趣的朋友一起来完善该项目.
|
||||||
|
* 知识星球: https://t.zsxq.com/7NMyO 沉淀高质量常见问题、最佳实践经验、问题解答
|
||||||
|
* 微信公众号:数字人技术
|
||||||
|
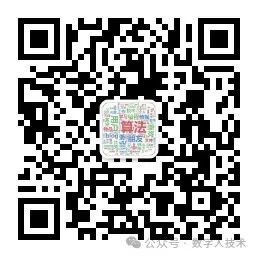
|
||||||
|
|
||||||
如果本项目对你有帮助,帮忙点个star。也欢迎感兴趣的朋友一起来完善该项目。
|
|
||||||
Email: lipku@foxmail.com
|
|
||||||
微信公众号:数字人技术
|
|
||||||
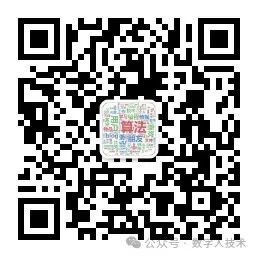
|
|
||||||
|
|
525
app.py
525
app.py
|
@ -1,5 +1,5 @@
|
||||||
# server.py
|
# server.py
|
||||||
from flask import Flask, request, jsonify
|
from flask import Flask, render_template,send_from_directory,request, jsonify
|
||||||
from flask_sockets import Sockets
|
from flask_sockets import Sockets
|
||||||
import base64
|
import base64
|
||||||
import time
|
import time
|
||||||
|
@ -10,96 +10,27 @@ from geventwebsocket.handler import WebSocketHandler
|
||||||
import os
|
import os
|
||||||
import re
|
import re
|
||||||
import numpy as np
|
import numpy as np
|
||||||
from threading import Thread
|
from threading import Thread,Event
|
||||||
import multiprocessing
|
import multiprocessing
|
||||||
|
|
||||||
|
from aiohttp import web
|
||||||
|
import aiohttp
|
||||||
|
import aiohttp_cors
|
||||||
|
from aiortc import RTCPeerConnection, RTCSessionDescription
|
||||||
|
from aiortc.rtcrtpsender import RTCRtpSender
|
||||||
|
from webrtc import HumanPlayer
|
||||||
|
|
||||||
import argparse
|
import argparse
|
||||||
from nerf_triplane.provider import NeRFDataset_Test
|
|
||||||
from nerf_triplane.utils import *
|
|
||||||
from nerf_triplane.network import NeRFNetwork
|
|
||||||
from nerfreal import NeRFReal
|
|
||||||
|
|
||||||
import shutil
|
import shutil
|
||||||
import asyncio
|
import asyncio
|
||||||
import edge_tts
|
import string
|
||||||
from typing import Iterator
|
|
||||||
|
|
||||||
import requests
|
|
||||||
|
|
||||||
app = Flask(__name__)
|
app = Flask(__name__)
|
||||||
sockets = Sockets(app)
|
sockets = Sockets(app)
|
||||||
global nerfreal
|
nerfreals = []
|
||||||
global tts_type
|
statreals = []
|
||||||
global gspeaker
|
|
||||||
|
|
||||||
|
|
||||||
async def main(voicename: str, text: str, render):
|
|
||||||
communicate = edge_tts.Communicate(text, voicename)
|
|
||||||
|
|
||||||
#with open(OUTPUT_FILE, "wb") as file:
|
|
||||||
async for chunk in communicate.stream():
|
|
||||||
if chunk["type"] == "audio":
|
|
||||||
render.push_audio(chunk["data"])
|
|
||||||
#file.write(chunk["data"])
|
|
||||||
elif chunk["type"] == "WordBoundary":
|
|
||||||
pass
|
|
||||||
|
|
||||||
def get_speaker(ref_audio,server_url):
|
|
||||||
files = {"wav_file": ("reference.wav", open(ref_audio, "rb"))}
|
|
||||||
response = requests.post(f"{server_url}/clone_speaker", files=files)
|
|
||||||
return response.json()
|
|
||||||
|
|
||||||
def xtts(text, speaker, language, server_url, stream_chunk_size) -> Iterator[bytes]:
|
|
||||||
start = time.perf_counter()
|
|
||||||
speaker["text"] = text
|
|
||||||
speaker["language"] = language
|
|
||||||
speaker["stream_chunk_size"] = stream_chunk_size # you can reduce it to get faster response, but degrade quality
|
|
||||||
res = requests.post(
|
|
||||||
f"{server_url}/tts_stream",
|
|
||||||
json=speaker,
|
|
||||||
stream=True,
|
|
||||||
)
|
|
||||||
end = time.perf_counter()
|
|
||||||
print(f"xtts Time to make POST: {end-start}s")
|
|
||||||
|
|
||||||
if res.status_code != 200:
|
|
||||||
print("Error:", res.text)
|
|
||||||
return
|
|
||||||
|
|
||||||
first = True
|
|
||||||
for chunk in res.iter_content(chunk_size=960):
|
|
||||||
if first:
|
|
||||||
end = time.perf_counter()
|
|
||||||
print(f"xtts Time to first chunk: {end-start}s")
|
|
||||||
first = False
|
|
||||||
if chunk:
|
|
||||||
yield chunk
|
|
||||||
|
|
||||||
print("xtts response.elapsed:", res.elapsed)
|
|
||||||
|
|
||||||
def stream_xtts(audio_stream,render):
|
|
||||||
for chunk in audio_stream:
|
|
||||||
if chunk is not None:
|
|
||||||
render.push_audio(chunk)
|
|
||||||
|
|
||||||
def txt_to_audio(text_):
|
|
||||||
if tts_type == "edgetts":
|
|
||||||
voicename = "zh-CN-YunxiaNeural"
|
|
||||||
text = text_
|
|
||||||
t = time.time()
|
|
||||||
asyncio.get_event_loop().run_until_complete(main(voicename,text,nerfreal))
|
|
||||||
print(f'-------edge tts time:{time.time()-t:.4f}s')
|
|
||||||
else: #xtts
|
|
||||||
stream_xtts(
|
|
||||||
xtts(
|
|
||||||
text_,
|
|
||||||
gspeaker,
|
|
||||||
"zh-cn", #en args.language,
|
|
||||||
"http://localhost:9000", #args.server_url,
|
|
||||||
"20" #args.stream_chunk_size
|
|
||||||
),
|
|
||||||
nerfreal
|
|
||||||
)
|
|
||||||
|
|
||||||
|
|
||||||
@sockets.route('/humanecho')
|
@sockets.route('/humanecho')
|
||||||
|
@ -119,16 +50,61 @@ def echo_socket(ws):
|
||||||
if not message or len(message)==0:
|
if not message or len(message)==0:
|
||||||
return '输入信息为空'
|
return '输入信息为空'
|
||||||
else:
|
else:
|
||||||
txt_to_audio(message)
|
nerfreal.put_msg_txt(message)
|
||||||
|
|
||||||
|
|
||||||
def llm_response(message):
|
# def llm_response(message):
|
||||||
from llm.LLM import LLM
|
# from llm.LLM import LLM
|
||||||
# llm = LLM().init_model('Gemini', model_path= 'gemini-pro',api_key='Your API Key', proxy_url=None)
|
# # llm = LLM().init_model('Gemini', model_path= 'gemini-pro',api_key='Your API Key', proxy_url=None)
|
||||||
llm = LLM().init_model('ChatGPT', model_path= 'gpt-3.5-turbo',api_key='Your API Key')
|
# # llm = LLM().init_model('ChatGPT', model_path= 'gpt-3.5-turbo',api_key='Your API Key')
|
||||||
response = llm.chat(message)
|
# llm = LLM().init_model('VllmGPT', model_path= 'THUDM/chatglm3-6b')
|
||||||
print(response)
|
# response = llm.chat(message)
|
||||||
return response
|
# print(response)
|
||||||
|
# return response
|
||||||
|
|
||||||
|
def llm_response(message,nerfreal):
|
||||||
|
start = time.perf_counter()
|
||||||
|
from openai import OpenAI
|
||||||
|
client = OpenAI(
|
||||||
|
# 如果您没有配置环境变量,请在此处用您的API Key进行替换
|
||||||
|
api_key=os.getenv("DASHSCOPE_API_KEY"),
|
||||||
|
# 填写DashScope SDK的base_url
|
||||||
|
base_url="https://dashscope.aliyuncs.com/compatible-mode/v1",
|
||||||
|
)
|
||||||
|
end = time.perf_counter()
|
||||||
|
print(f"llm Time init: {end-start}s")
|
||||||
|
completion = client.chat.completions.create(
|
||||||
|
model="qwen-plus",
|
||||||
|
messages=[{'role': 'system', 'content': 'You are a helpful assistant.'},
|
||||||
|
{'role': 'user', 'content': message}],
|
||||||
|
stream=True,
|
||||||
|
# 通过以下设置,在流式输出的最后一行展示token使用信息
|
||||||
|
stream_options={"include_usage": True}
|
||||||
|
)
|
||||||
|
result=""
|
||||||
|
first = True
|
||||||
|
for chunk in completion:
|
||||||
|
if len(chunk.choices)>0:
|
||||||
|
#print(chunk.choices[0].delta.content)
|
||||||
|
if first:
|
||||||
|
end = time.perf_counter()
|
||||||
|
print(f"llm Time to first chunk: {end-start}s")
|
||||||
|
first = False
|
||||||
|
msg = chunk.choices[0].delta.content
|
||||||
|
lastpos=0
|
||||||
|
#msglist = re.split('[,.!;:,。!?]',msg)
|
||||||
|
for i, char in enumerate(msg):
|
||||||
|
if char in ",.!;:,。!?:;" :
|
||||||
|
result = result+msg[lastpos:i+1]
|
||||||
|
lastpos = i+1
|
||||||
|
if len(result)>10:
|
||||||
|
print(result)
|
||||||
|
nerfreal.put_msg_txt(result)
|
||||||
|
result=""
|
||||||
|
result = result+msg[lastpos:]
|
||||||
|
end = time.perf_counter()
|
||||||
|
print(f"llm Time to last chunk: {end-start}s")
|
||||||
|
nerfreal.put_msg_txt(result)
|
||||||
|
|
||||||
@sockets.route('/humanchat')
|
@sockets.route('/humanchat')
|
||||||
def chat_socket(ws):
|
def chat_socket(ws):
|
||||||
|
@ -148,14 +124,185 @@ def chat_socket(ws):
|
||||||
return '输入信息为空'
|
return '输入信息为空'
|
||||||
else:
|
else:
|
||||||
res=llm_response(message)
|
res=llm_response(message)
|
||||||
txt_to_audio(res)
|
nerfreal.put_msg_txt(res)
|
||||||
|
|
||||||
def render():
|
#####webrtc###############################
|
||||||
nerfreal.render()
|
pcs = set()
|
||||||
|
|
||||||
|
|
||||||
|
#@app.route('/offer', methods=['POST'])
|
||||||
|
async def offer(request):
|
||||||
|
params = await request.json()
|
||||||
|
offer = RTCSessionDescription(sdp=params["sdp"], type=params["type"])
|
||||||
|
|
||||||
|
sessionid = len(nerfreals)
|
||||||
|
for index,value in enumerate(statreals):
|
||||||
|
if value == 0:
|
||||||
|
sessionid = index
|
||||||
|
break
|
||||||
|
if sessionid>=len(nerfreals):
|
||||||
|
print('reach max session')
|
||||||
|
return -1
|
||||||
|
statreals[sessionid] = 1
|
||||||
|
|
||||||
|
pc = RTCPeerConnection()
|
||||||
|
pcs.add(pc)
|
||||||
|
|
||||||
|
@pc.on("connectionstatechange")
|
||||||
|
async def on_connectionstatechange():
|
||||||
|
print("Connection state is %s" % pc.connectionState)
|
||||||
|
if pc.connectionState == "failed":
|
||||||
|
await pc.close()
|
||||||
|
pcs.discard(pc)
|
||||||
|
statreals[sessionid] = 0
|
||||||
|
if pc.connectionState == "closed":
|
||||||
|
pcs.discard(pc)
|
||||||
|
statreals[sessionid] = 0
|
||||||
|
|
||||||
|
player = HumanPlayer(nerfreals[sessionid])
|
||||||
|
audio_sender = pc.addTrack(player.audio)
|
||||||
|
video_sender = pc.addTrack(player.video)
|
||||||
|
capabilities = RTCRtpSender.getCapabilities("video")
|
||||||
|
preferences = list(filter(lambda x: x.name == "H264", capabilities.codecs))
|
||||||
|
preferences += list(filter(lambda x: x.name == "VP8", capabilities.codecs))
|
||||||
|
preferences += list(filter(lambda x: x.name == "rtx", capabilities.codecs))
|
||||||
|
transceiver = pc.getTransceivers()[1]
|
||||||
|
transceiver.setCodecPreferences(preferences)
|
||||||
|
|
||||||
|
await pc.setRemoteDescription(offer)
|
||||||
|
|
||||||
|
answer = await pc.createAnswer()
|
||||||
|
await pc.setLocalDescription(answer)
|
||||||
|
|
||||||
|
#return jsonify({"sdp": pc.localDescription.sdp, "type": pc.localDescription.type})
|
||||||
|
|
||||||
|
return web.Response(
|
||||||
|
content_type="application/json",
|
||||||
|
text=json.dumps(
|
||||||
|
{"sdp": pc.localDescription.sdp, "type": pc.localDescription.type, "sessionid":sessionid}
|
||||||
|
),
|
||||||
|
)
|
||||||
|
|
||||||
|
async def human(request):
|
||||||
|
params = await request.json()
|
||||||
|
|
||||||
|
sessionid = params.get('sessionid',0)
|
||||||
|
if params.get('interrupt'):
|
||||||
|
nerfreals[sessionid].pause_talk()
|
||||||
|
|
||||||
|
if params['type']=='echo':
|
||||||
|
nerfreals[sessionid].put_msg_txt(params['text'])
|
||||||
|
elif params['type']=='chat':
|
||||||
|
res=await asyncio.get_event_loop().run_in_executor(None, llm_response, params['text'],nerfreals[sessionid])
|
||||||
|
#nerfreals[sessionid].put_msg_txt(res)
|
||||||
|
|
||||||
|
return web.Response(
|
||||||
|
content_type="application/json",
|
||||||
|
text=json.dumps(
|
||||||
|
{"code": 0, "data":"ok"}
|
||||||
|
),
|
||||||
|
)
|
||||||
|
|
||||||
|
async def humanaudio(request):
|
||||||
|
try:
|
||||||
|
form= await request.post()
|
||||||
|
sessionid = int(form.get('sessionid',0))
|
||||||
|
fileobj = form["file"]
|
||||||
|
filename=fileobj.filename
|
||||||
|
filebytes=fileobj.file.read()
|
||||||
|
nerfreals[sessionid].put_audio_file(filebytes)
|
||||||
|
|
||||||
|
return web.Response(
|
||||||
|
content_type="application/json",
|
||||||
|
text=json.dumps(
|
||||||
|
{"code": 0, "msg":"ok"}
|
||||||
|
),
|
||||||
|
)
|
||||||
|
except Exception as e:
|
||||||
|
return web.Response(
|
||||||
|
content_type="application/json",
|
||||||
|
text=json.dumps(
|
||||||
|
{"code": -1, "msg":"err","data": ""+e.args[0]+""}
|
||||||
|
),
|
||||||
|
)
|
||||||
|
|
||||||
|
async def set_audiotype(request):
|
||||||
|
params = await request.json()
|
||||||
|
|
||||||
|
sessionid = params.get('sessionid',0)
|
||||||
|
nerfreals[sessionid].set_curr_state(params['audiotype'],params['reinit'])
|
||||||
|
|
||||||
|
return web.Response(
|
||||||
|
content_type="application/json",
|
||||||
|
text=json.dumps(
|
||||||
|
{"code": 0, "data":"ok"}
|
||||||
|
),
|
||||||
|
)
|
||||||
|
|
||||||
|
async def record(request):
|
||||||
|
params = await request.json()
|
||||||
|
|
||||||
|
sessionid = params.get('sessionid',0)
|
||||||
|
if params['type']=='start_record':
|
||||||
|
# nerfreals[sessionid].put_msg_txt(params['text'])
|
||||||
|
nerfreals[sessionid].start_recording("data/record_lasted.mp4")
|
||||||
|
elif params['type']=='end_record':
|
||||||
|
nerfreals[sessionid].stop_recording()
|
||||||
|
return web.Response(
|
||||||
|
content_type="application/json",
|
||||||
|
text=json.dumps(
|
||||||
|
{"code": 0, "data":"ok"}
|
||||||
|
),
|
||||||
|
)
|
||||||
|
|
||||||
|
async def is_speaking(request):
|
||||||
|
params = await request.json()
|
||||||
|
|
||||||
|
sessionid = params.get('sessionid',0)
|
||||||
|
return web.Response(
|
||||||
|
content_type="application/json",
|
||||||
|
text=json.dumps(
|
||||||
|
{"code": 0, "data": nerfreals[sessionid].is_speaking()}
|
||||||
|
),
|
||||||
|
)
|
||||||
|
|
||||||
|
|
||||||
|
async def on_shutdown(app):
|
||||||
|
# close peer connections
|
||||||
|
coros = [pc.close() for pc in pcs]
|
||||||
|
await asyncio.gather(*coros)
|
||||||
|
pcs.clear()
|
||||||
|
|
||||||
|
async def post(url,data):
|
||||||
|
try:
|
||||||
|
async with aiohttp.ClientSession() as session:
|
||||||
|
async with session.post(url,data=data) as response:
|
||||||
|
return await response.text()
|
||||||
|
except aiohttp.ClientError as e:
|
||||||
|
print(f'Error: {e}')
|
||||||
|
|
||||||
|
async def run(push_url):
|
||||||
|
pc = RTCPeerConnection()
|
||||||
|
pcs.add(pc)
|
||||||
|
|
||||||
|
@pc.on("connectionstatechange")
|
||||||
|
async def on_connectionstatechange():
|
||||||
|
print("Connection state is %s" % pc.connectionState)
|
||||||
|
if pc.connectionState == "failed":
|
||||||
|
await pc.close()
|
||||||
|
pcs.discard(pc)
|
||||||
|
|
||||||
|
player = HumanPlayer(nerfreals[0])
|
||||||
|
audio_sender = pc.addTrack(player.audio)
|
||||||
|
video_sender = pc.addTrack(player.video)
|
||||||
|
|
||||||
|
await pc.setLocalDescription(await pc.createOffer())
|
||||||
|
answer = await post(push_url,pc.localDescription.sdp)
|
||||||
|
await pc.setRemoteDescription(RTCSessionDescription(sdp=answer,type='answer'))
|
||||||
|
##########################################
|
||||||
|
# os.environ['MKL_SERVICE_FORCE_INTEL'] = '1'
|
||||||
|
# os.environ['MULTIPROCESSING_METHOD'] = 'forkserver'
|
||||||
if __name__ == '__main__':
|
if __name__ == '__main__':
|
||||||
|
multiprocessing.set_start_method('spawn')
|
||||||
parser = argparse.ArgumentParser()
|
parser = argparse.ArgumentParser()
|
||||||
parser.add_argument('--pose', type=str, default="data/data_kf.json", help="transforms.json, pose source")
|
parser.add_argument('--pose', type=str, default="data/data_kf.json", help="transforms.json, pose source")
|
||||||
parser.add_argument('--au', type=str, default="data/au.csv", help="eye blink area")
|
parser.add_argument('--au', type=str, default="data/au.csv", help="eye blink area")
|
||||||
|
@ -248,17 +395,16 @@ if __name__ == '__main__':
|
||||||
parser.add_argument('--asr_play', action='store_true', help="play out the audio")
|
parser.add_argument('--asr_play', action='store_true', help="play out the audio")
|
||||||
|
|
||||||
#parser.add_argument('--asr_model', type=str, default='deepspeech')
|
#parser.add_argument('--asr_model', type=str, default='deepspeech')
|
||||||
parser.add_argument('--asr_model', type=str, default='cpierse/wav2vec2-large-xlsr-53-esperanto') #facebook/hubert-large-ls960-ft
|
parser.add_argument('--asr_model', type=str, default='cpierse/wav2vec2-large-xlsr-53-esperanto') #
|
||||||
# parser.add_argument('--asr_model', type=str, default='facebook/wav2vec2-large-960h-lv60-self')
|
# parser.add_argument('--asr_model', type=str, default='facebook/wav2vec2-large-960h-lv60-self')
|
||||||
|
# parser.add_argument('--asr_model', type=str, default='facebook/hubert-large-ls960-ft')
|
||||||
parser.add_argument('--push_url', type=str, default='rtmp://localhost/live/livestream')
|
|
||||||
|
|
||||||
parser.add_argument('--asr_save_feats', action='store_true')
|
parser.add_argument('--asr_save_feats', action='store_true')
|
||||||
# audio FPS
|
# audio FPS
|
||||||
parser.add_argument('--fps', type=int, default=50)
|
parser.add_argument('--fps', type=int, default=50)
|
||||||
# sliding window left-middle-right length (unit: 20ms)
|
# sliding window left-middle-right length (unit: 20ms)
|
||||||
parser.add_argument('-l', type=int, default=10)
|
parser.add_argument('-l', type=int, default=10)
|
||||||
parser.add_argument('-m', type=int, default=50)
|
parser.add_argument('-m', type=int, default=8)
|
||||||
parser.add_argument('-r', type=int, default=10)
|
parser.add_argument('-r', type=int, default=10)
|
||||||
|
|
||||||
parser.add_argument('--fullbody', action='store_true', help="fullbody human")
|
parser.add_argument('--fullbody', action='store_true', help="fullbody human")
|
||||||
|
@ -268,69 +414,156 @@ if __name__ == '__main__':
|
||||||
parser.add_argument('--fullbody_offset_x', type=int, default=0)
|
parser.add_argument('--fullbody_offset_x', type=int, default=0)
|
||||||
parser.add_argument('--fullbody_offset_y', type=int, default=0)
|
parser.add_argument('--fullbody_offset_y', type=int, default=0)
|
||||||
|
|
||||||
parser.add_argument('--tts', type=str, default='edgetts') #xtts
|
#musetalk opt
|
||||||
parser.add_argument('--ref_file', type=str, default=None)
|
parser.add_argument('--avatar_id', type=str, default='avator_1')
|
||||||
parser.add_argument('--xtts_server', type=str, default='http://localhost:9000')
|
parser.add_argument('--bbox_shift', type=int, default=5)
|
||||||
|
parser.add_argument('--batch_size', type=int, default=16)
|
||||||
|
|
||||||
|
# parser.add_argument('--customvideo', action='store_true', help="custom video")
|
||||||
|
# parser.add_argument('--customvideo_img', type=str, default='data/customvideo/img')
|
||||||
|
# parser.add_argument('--customvideo_imgnum', type=int, default=1)
|
||||||
|
|
||||||
|
parser.add_argument('--customvideo_config', type=str, default='')
|
||||||
|
|
||||||
|
parser.add_argument('--tts', type=str, default='edgetts') #xtts gpt-sovits cosyvoice
|
||||||
|
parser.add_argument('--REF_FILE', type=str, default=None)
|
||||||
|
parser.add_argument('--REF_TEXT', type=str, default=None)
|
||||||
|
parser.add_argument('--TTS_SERVER', type=str, default='http://127.0.0.1:9880') # http://localhost:9000
|
||||||
|
# parser.add_argument('--CHARACTER', type=str, default='test')
|
||||||
|
# parser.add_argument('--EMOTION', type=str, default='default')
|
||||||
|
|
||||||
|
parser.add_argument('--model', type=str, default='ernerf') #musetalk wav2lip
|
||||||
|
|
||||||
|
parser.add_argument('--transport', type=str, default='rtcpush') #rtmp webrtc rtcpush
|
||||||
|
parser.add_argument('--push_url', type=str, default='http://localhost:1985/rtc/v1/whip/?app=live&stream=livestream') #rtmp://localhost/live/livestream
|
||||||
|
|
||||||
|
parser.add_argument('--max_session', type=int, default=1) #multi session count
|
||||||
|
parser.add_argument('--listenport', type=int, default=8010)
|
||||||
|
|
||||||
opt = parser.parse_args()
|
opt = parser.parse_args()
|
||||||
app.config.from_object(opt)
|
#app.config.from_object(opt)
|
||||||
#print(app.config['xtts_server'])
|
#print(app.config)
|
||||||
|
opt.customopt = []
|
||||||
|
if opt.customvideo_config!='':
|
||||||
|
with open(opt.customvideo_config,'r') as file:
|
||||||
|
opt.customopt = json.load(file)
|
||||||
|
|
||||||
tts_type = opt.tts
|
if opt.model == 'ernerf':
|
||||||
if tts_type == "xtts":
|
from ernerf.nerf_triplane.provider import NeRFDataset_Test
|
||||||
print("Computing the latents for a new reference...")
|
from ernerf.nerf_triplane.utils import *
|
||||||
gspeaker = get_speaker(opt.ref_file, opt.xtts_server)
|
from ernerf.nerf_triplane.network import NeRFNetwork
|
||||||
|
from nerfreal import NeRFReal
|
||||||
|
# assert test mode
|
||||||
|
opt.test = True
|
||||||
|
opt.test_train = False
|
||||||
|
#opt.train_camera =True
|
||||||
|
# explicit smoothing
|
||||||
|
opt.smooth_path = True
|
||||||
|
opt.smooth_lips = True
|
||||||
|
|
||||||
# assert test mode
|
assert opt.pose != '', 'Must provide a pose source'
|
||||||
opt.test = True
|
|
||||||
opt.test_train = False
|
|
||||||
#opt.train_camera =True
|
|
||||||
# explicit smoothing
|
|
||||||
opt.smooth_path = True
|
|
||||||
opt.smooth_lips = True
|
|
||||||
|
|
||||||
assert opt.pose != '', 'Must provide a pose source'
|
# if opt.O:
|
||||||
|
opt.fp16 = True
|
||||||
|
opt.cuda_ray = True
|
||||||
|
opt.exp_eye = True
|
||||||
|
opt.smooth_eye = True
|
||||||
|
|
||||||
# if opt.O:
|
if opt.torso_imgs=='': #no img,use model output
|
||||||
opt.fp16 = True
|
opt.torso = True
|
||||||
opt.cuda_ray = True
|
|
||||||
opt.exp_eye = True
|
|
||||||
opt.smooth_eye = True
|
|
||||||
|
|
||||||
if opt.torso_imgs=='': #no img,use model output
|
# assert opt.cuda_ray, "Only support CUDA ray mode."
|
||||||
opt.torso = True
|
opt.asr = True
|
||||||
|
|
||||||
# assert opt.cuda_ray, "Only support CUDA ray mode."
|
if opt.patch_size > 1:
|
||||||
opt.asr = True
|
# assert opt.patch_size > 16, "patch_size should > 16 to run LPIPS loss."
|
||||||
|
assert opt.num_rays % (opt.patch_size ** 2) == 0, "patch_size ** 2 should be dividable by num_rays."
|
||||||
|
seed_everything(opt.seed)
|
||||||
|
print(opt)
|
||||||
|
|
||||||
if opt.patch_size > 1:
|
device = torch.device('cuda' if torch.cuda.is_available() else 'cpu')
|
||||||
# assert opt.patch_size > 16, "patch_size should > 16 to run LPIPS loss."
|
model = NeRFNetwork(opt)
|
||||||
assert opt.num_rays % (opt.patch_size ** 2) == 0, "patch_size ** 2 should be dividable by num_rays."
|
|
||||||
seed_everything(opt.seed)
|
|
||||||
print(opt)
|
|
||||||
|
|
||||||
device = torch.device('cuda' if torch.cuda.is_available() else 'cpu')
|
criterion = torch.nn.MSELoss(reduction='none')
|
||||||
model = NeRFNetwork(opt)
|
metrics = [] # use no metric in GUI for faster initialization...
|
||||||
|
print(model)
|
||||||
|
trainer = Trainer('ngp', opt, model, device=device, workspace=opt.workspace, criterion=criterion, fp16=opt.fp16, metrics=metrics, use_checkpoint=opt.ckpt)
|
||||||
|
|
||||||
criterion = torch.nn.MSELoss(reduction='none')
|
test_loader = NeRFDataset_Test(opt, device=device).dataloader()
|
||||||
metrics = [] # use no metric in GUI for faster initialization...
|
model.aud_features = test_loader._data.auds
|
||||||
print(model)
|
model.eye_areas = test_loader._data.eye_area
|
||||||
trainer = Trainer('ngp', opt, model, device=device, workspace=opt.workspace, criterion=criterion, fp16=opt.fp16, metrics=metrics, use_checkpoint=opt.ckpt)
|
|
||||||
|
|
||||||
test_loader = NeRFDataset_Test(opt, device=device).dataloader()
|
# we still need test_loader to provide audio features for testing.
|
||||||
model.aud_features = test_loader._data.auds
|
for _ in range(opt.max_session):
|
||||||
model.eye_areas = test_loader._data.eye_area
|
nerfreal = NeRFReal(opt, trainer, test_loader)
|
||||||
|
nerfreals.append(nerfreal)
|
||||||
|
elif opt.model == 'musetalk':
|
||||||
|
from musereal import MuseReal
|
||||||
|
print(opt)
|
||||||
|
for _ in range(opt.max_session):
|
||||||
|
nerfreal = MuseReal(opt)
|
||||||
|
nerfreals.append(nerfreal)
|
||||||
|
elif opt.model == 'wav2lip':
|
||||||
|
from lipreal import LipReal
|
||||||
|
print(opt)
|
||||||
|
for _ in range(opt.max_session):
|
||||||
|
nerfreal = LipReal(opt)
|
||||||
|
nerfreals.append(nerfreal)
|
||||||
|
|
||||||
|
for _ in range(opt.max_session):
|
||||||
|
statreals.append(0)
|
||||||
|
|
||||||
# we still need test_loader to provide audio features for testing.
|
if opt.transport=='rtmp':
|
||||||
nerfreal = NeRFReal(opt, trainer, test_loader)
|
thread_quit = Event()
|
||||||
#txt_to_audio('我是中国人,我来自北京')
|
rendthrd = Thread(target=nerfreals[0].render,args=(thread_quit,))
|
||||||
rendthrd = Thread(target=render)
|
rendthrd.start()
|
||||||
rendthrd.start()
|
|
||||||
|
|
||||||
#############################################################################
|
#############################################################################
|
||||||
print('start websocket server')
|
appasync = web.Application()
|
||||||
|
appasync.on_shutdown.append(on_shutdown)
|
||||||
|
appasync.router.add_post("/offer", offer)
|
||||||
|
appasync.router.add_post("/human", human)
|
||||||
|
appasync.router.add_post("/humanaudio", humanaudio)
|
||||||
|
appasync.router.add_post("/set_audiotype", set_audiotype)
|
||||||
|
appasync.router.add_post("/record", record)
|
||||||
|
appasync.router.add_post("/is_speaking", is_speaking)
|
||||||
|
appasync.router.add_static('/',path='web')
|
||||||
|
|
||||||
server = pywsgi.WSGIServer(('0.0.0.0', 8000), app, handler_class=WebSocketHandler)
|
# Configure default CORS settings.
|
||||||
server.serve_forever()
|
cors = aiohttp_cors.setup(appasync, defaults={
|
||||||
|
"*": aiohttp_cors.ResourceOptions(
|
||||||
|
allow_credentials=True,
|
||||||
|
expose_headers="*",
|
||||||
|
allow_headers="*",
|
||||||
|
)
|
||||||
|
})
|
||||||
|
# Configure CORS on all routes.
|
||||||
|
for route in list(appasync.router.routes()):
|
||||||
|
cors.add(route)
|
||||||
|
|
||||||
|
pagename='webrtcapi.html'
|
||||||
|
if opt.transport=='rtmp':
|
||||||
|
pagename='echoapi.html'
|
||||||
|
elif opt.transport=='rtcpush':
|
||||||
|
pagename='rtcpushapi.html'
|
||||||
|
print('start http server; http://<serverip>:'+str(opt.listenport)+'/'+pagename)
|
||||||
|
def run_server(runner):
|
||||||
|
loop = asyncio.new_event_loop()
|
||||||
|
asyncio.set_event_loop(loop)
|
||||||
|
loop.run_until_complete(runner.setup())
|
||||||
|
site = web.TCPSite(runner, '0.0.0.0', opt.listenport)
|
||||||
|
loop.run_until_complete(site.start())
|
||||||
|
if opt.transport=='rtcpush':
|
||||||
|
loop.run_until_complete(run(opt.push_url))
|
||||||
|
loop.run_forever()
|
||||||
|
#Thread(target=run_server, args=(web.AppRunner(appasync),)).start()
|
||||||
|
run_server(web.AppRunner(appasync))
|
||||||
|
|
||||||
|
#app.on_shutdown.append(on_shutdown)
|
||||||
|
#app.router.add_post("/offer", offer)
|
||||||
|
|
||||||
|
# print('start websocket server')
|
||||||
|
# server = pywsgi.WSGIServer(('0.0.0.0', 8000), app, handler_class=WebSocketHandler)
|
||||||
|
# server.serve_forever()
|
||||||
|
|
||||||
|
|
496
asrreal.py
496
asrreal.py
|
@ -1,496 +0,0 @@
|
||||||
import time
|
|
||||||
import numpy as np
|
|
||||||
import torch
|
|
||||||
import torch.nn.functional as F
|
|
||||||
from transformers import AutoModelForCTC, AutoProcessor, Wav2Vec2Processor, HubertModel
|
|
||||||
|
|
||||||
#import pyaudio
|
|
||||||
import soundfile as sf
|
|
||||||
import resampy
|
|
||||||
|
|
||||||
import queue
|
|
||||||
from queue import Queue
|
|
||||||
#from collections import deque
|
|
||||||
from threading import Thread, Event
|
|
||||||
from io import BytesIO
|
|
||||||
|
|
||||||
|
|
||||||
def _read_frame(stream, exit_event, queue, chunk):
|
|
||||||
|
|
||||||
while True:
|
|
||||||
if exit_event.is_set():
|
|
||||||
print(f'[INFO] read frame thread ends')
|
|
||||||
break
|
|
||||||
frame = stream.read(chunk, exception_on_overflow=False)
|
|
||||||
frame = np.frombuffer(frame, dtype=np.int16).astype(np.float32) / 32767 # [chunk]
|
|
||||||
queue.put(frame)
|
|
||||||
|
|
||||||
def _play_frame(stream, exit_event, queue, chunk):
|
|
||||||
|
|
||||||
while True:
|
|
||||||
if exit_event.is_set():
|
|
||||||
print(f'[INFO] play frame thread ends')
|
|
||||||
break
|
|
||||||
frame = queue.get()
|
|
||||||
frame = (frame * 32767).astype(np.int16).tobytes()
|
|
||||||
stream.write(frame, chunk)
|
|
||||||
|
|
||||||
class ASR:
|
|
||||||
def __init__(self, opt):
|
|
||||||
|
|
||||||
self.opt = opt
|
|
||||||
|
|
||||||
self.play = opt.asr_play #false
|
|
||||||
|
|
||||||
self.device = 'cuda' if torch.cuda.is_available() else 'cpu'
|
|
||||||
self.fps = opt.fps # 20 ms per frame
|
|
||||||
self.sample_rate = 16000
|
|
||||||
self.chunk = self.sample_rate // self.fps # 320 samples per chunk (20ms * 16000 / 1000)
|
|
||||||
self.mode = 'live' if opt.asr_wav == '' else 'file'
|
|
||||||
|
|
||||||
if 'esperanto' in self.opt.asr_model:
|
|
||||||
self.audio_dim = 44
|
|
||||||
elif 'deepspeech' in self.opt.asr_model:
|
|
||||||
self.audio_dim = 29
|
|
||||||
elif 'hubert' in self.opt.asr_model:
|
|
||||||
self.audio_dim = 1024
|
|
||||||
else:
|
|
||||||
self.audio_dim = 32
|
|
||||||
|
|
||||||
# prepare context cache
|
|
||||||
# each segment is (stride_left + ctx + stride_right) * 20ms, latency should be (ctx + stride_right) * 20ms
|
|
||||||
self.context_size = opt.m
|
|
||||||
self.stride_left_size = opt.l
|
|
||||||
self.stride_right_size = opt.r
|
|
||||||
self.text = '[START]\n'
|
|
||||||
self.terminated = False
|
|
||||||
self.frames = []
|
|
||||||
self.inwarm = False
|
|
||||||
|
|
||||||
# pad left frames
|
|
||||||
if self.stride_left_size > 0:
|
|
||||||
self.frames.extend([np.zeros(self.chunk, dtype=np.float32)] * self.stride_left_size)
|
|
||||||
|
|
||||||
|
|
||||||
self.exit_event = Event()
|
|
||||||
#self.audio_instance = pyaudio.PyAudio() #not need
|
|
||||||
|
|
||||||
# create input stream
|
|
||||||
if self.mode == 'file': #live mode
|
|
||||||
self.file_stream = self.create_file_stream()
|
|
||||||
else:
|
|
||||||
self.queue = Queue()
|
|
||||||
self.input_stream = BytesIO()
|
|
||||||
self.output_queue = Queue()
|
|
||||||
# start a background process to read frames
|
|
||||||
#self.input_stream = self.audio_instance.open(format=pyaudio.paInt16, channels=1, rate=self.sample_rate, input=True, output=False, frames_per_buffer=self.chunk)
|
|
||||||
#self.queue = Queue()
|
|
||||||
#self.process_read_frame = Thread(target=_read_frame, args=(self.input_stream, self.exit_event, self.queue, self.chunk))
|
|
||||||
|
|
||||||
# play out the audio too...?
|
|
||||||
if self.play:
|
|
||||||
self.output_stream = self.audio_instance.open(format=pyaudio.paInt16, channels=1, rate=self.sample_rate, input=False, output=True, frames_per_buffer=self.chunk)
|
|
||||||
self.output_queue = Queue()
|
|
||||||
self.process_play_frame = Thread(target=_play_frame, args=(self.output_stream, self.exit_event, self.output_queue, self.chunk))
|
|
||||||
|
|
||||||
# current location of audio
|
|
||||||
self.idx = 0
|
|
||||||
|
|
||||||
# create wav2vec model
|
|
||||||
print(f'[INFO] loading ASR model {self.opt.asr_model}...')
|
|
||||||
if 'hubert' in self.opt.asr_model:
|
|
||||||
self.processor = Wav2Vec2Processor.from_pretrained(opt.asr_model)
|
|
||||||
self.model = HubertModel.from_pretrained(opt.asr_model).to(self.device)
|
|
||||||
else:
|
|
||||||
self.processor = AutoProcessor.from_pretrained(opt.asr_model)
|
|
||||||
self.model = AutoModelForCTC.from_pretrained(opt.asr_model).to(self.device)
|
|
||||||
|
|
||||||
# prepare to save logits
|
|
||||||
if self.opt.asr_save_feats:
|
|
||||||
self.all_feats = []
|
|
||||||
|
|
||||||
# the extracted features
|
|
||||||
# use a loop queue to efficiently record endless features: [f--t---][-------][-------]
|
|
||||||
self.feat_buffer_size = 4
|
|
||||||
self.feat_buffer_idx = 0
|
|
||||||
self.feat_queue = torch.zeros(self.feat_buffer_size * self.context_size, self.audio_dim, dtype=torch.float32, device=self.device)
|
|
||||||
|
|
||||||
# TODO: hard coded 16 and 8 window size...
|
|
||||||
self.front = self.feat_buffer_size * self.context_size - 8 # fake padding
|
|
||||||
self.tail = 8
|
|
||||||
# attention window...
|
|
||||||
self.att_feats = [torch.zeros(self.audio_dim, 16, dtype=torch.float32, device=self.device)] * 4 # 4 zero padding...
|
|
||||||
|
|
||||||
# warm up steps needed: mid + right + window_size + attention_size
|
|
||||||
self.warm_up_steps = self.context_size + self.stride_right_size + self.stride_left_size #+ 8 + 2 * 3
|
|
||||||
|
|
||||||
self.listening = False
|
|
||||||
self.playing = False
|
|
||||||
|
|
||||||
def listen(self):
|
|
||||||
# start
|
|
||||||
if self.mode == 'live' and not self.listening:
|
|
||||||
print(f'[INFO] starting read frame thread...')
|
|
||||||
self.process_read_frame.start()
|
|
||||||
self.listening = True
|
|
||||||
|
|
||||||
if self.play and not self.playing:
|
|
||||||
print(f'[INFO] starting play frame thread...')
|
|
||||||
self.process_play_frame.start()
|
|
||||||
self.playing = True
|
|
||||||
|
|
||||||
def stop(self):
|
|
||||||
|
|
||||||
self.exit_event.set()
|
|
||||||
|
|
||||||
if self.play:
|
|
||||||
self.output_stream.stop_stream()
|
|
||||||
self.output_stream.close()
|
|
||||||
if self.playing:
|
|
||||||
self.process_play_frame.join()
|
|
||||||
self.playing = False
|
|
||||||
|
|
||||||
if self.mode == 'live':
|
|
||||||
#self.input_stream.stop_stream() todo
|
|
||||||
self.input_stream.close()
|
|
||||||
if self.listening:
|
|
||||||
self.process_read_frame.join()
|
|
||||||
self.listening = False
|
|
||||||
|
|
||||||
|
|
||||||
def __enter__(self):
|
|
||||||
return self
|
|
||||||
|
|
||||||
def __exit__(self, exc_type, exc_value, traceback):
|
|
||||||
|
|
||||||
self.stop()
|
|
||||||
|
|
||||||
if self.mode == 'live':
|
|
||||||
# live mode: also print the result text.
|
|
||||||
self.text += '\n[END]'
|
|
||||||
print(self.text)
|
|
||||||
|
|
||||||
def get_next_feat(self):
|
|
||||||
# return a [1/8, 16] window, for the next input to nerf side.
|
|
||||||
|
|
||||||
while len(self.att_feats) < 8:
|
|
||||||
# [------f+++t-----]
|
|
||||||
if self.front < self.tail:
|
|
||||||
feat = self.feat_queue[self.front:self.tail]
|
|
||||||
# [++t-----------f+]
|
|
||||||
else:
|
|
||||||
feat = torch.cat([self.feat_queue[self.front:], self.feat_queue[:self.tail]], dim=0)
|
|
||||||
|
|
||||||
self.front = (self.front + 2) % self.feat_queue.shape[0]
|
|
||||||
self.tail = (self.tail + 2) % self.feat_queue.shape[0]
|
|
||||||
|
|
||||||
# print(self.front, self.tail, feat.shape)
|
|
||||||
|
|
||||||
self.att_feats.append(feat.permute(1, 0))
|
|
||||||
|
|
||||||
att_feat = torch.stack(self.att_feats, dim=0) # [8, 44, 16]
|
|
||||||
|
|
||||||
# discard old
|
|
||||||
self.att_feats = self.att_feats[1:]
|
|
||||||
|
|
||||||
return att_feat
|
|
||||||
|
|
||||||
def run_step(self):
|
|
||||||
|
|
||||||
if self.terminated:
|
|
||||||
return
|
|
||||||
|
|
||||||
# get a frame of audio
|
|
||||||
frame = self.get_audio_frame()
|
|
||||||
|
|
||||||
# the last frame
|
|
||||||
if frame is None:
|
|
||||||
# terminate, but always run the network for the left frames
|
|
||||||
self.terminated = True
|
|
||||||
else:
|
|
||||||
self.frames.append(frame)
|
|
||||||
# put to output
|
|
||||||
self.output_queue.put(frame)
|
|
||||||
# context not enough, do not run network.
|
|
||||||
if len(self.frames) < self.stride_left_size + self.context_size + self.stride_right_size:
|
|
||||||
return
|
|
||||||
|
|
||||||
inputs = np.concatenate(self.frames) # [N * chunk]
|
|
||||||
|
|
||||||
# discard the old part to save memory
|
|
||||||
if not self.terminated:
|
|
||||||
self.frames = self.frames[-(self.stride_left_size + self.stride_right_size):]
|
|
||||||
|
|
||||||
print(f'[INFO] frame_to_text... ')
|
|
||||||
#t = time.time()
|
|
||||||
logits, labels, text = self.frame_to_text(inputs)
|
|
||||||
#print(f'-------wav2vec time:{time.time()-t:.4f}s')
|
|
||||||
feats = logits # better lips-sync than labels
|
|
||||||
|
|
||||||
# save feats
|
|
||||||
if self.opt.asr_save_feats:
|
|
||||||
self.all_feats.append(feats)
|
|
||||||
|
|
||||||
# record the feats efficiently.. (no concat, constant memory)
|
|
||||||
start = self.feat_buffer_idx * self.context_size
|
|
||||||
end = start + feats.shape[0]
|
|
||||||
self.feat_queue[start:end] = feats
|
|
||||||
self.feat_buffer_idx = (self.feat_buffer_idx + 1) % self.feat_buffer_size
|
|
||||||
|
|
||||||
# very naive, just concat the text output.
|
|
||||||
#if text != '':
|
|
||||||
# self.text = self.text + ' ' + text
|
|
||||||
|
|
||||||
# will only run once at ternimation
|
|
||||||
if self.terminated:
|
|
||||||
self.text += '\n[END]'
|
|
||||||
print(self.text)
|
|
||||||
if self.opt.asr_save_feats:
|
|
||||||
print(f'[INFO] save all feats for training purpose... ')
|
|
||||||
feats = torch.cat(self.all_feats, dim=0) # [N, C]
|
|
||||||
# print('[INFO] before unfold', feats.shape)
|
|
||||||
window_size = 16
|
|
||||||
padding = window_size // 2
|
|
||||||
feats = feats.view(-1, self.audio_dim).permute(1, 0).contiguous() # [C, M]
|
|
||||||
feats = feats.view(1, self.audio_dim, -1, 1) # [1, C, M, 1]
|
|
||||||
unfold_feats = F.unfold(feats, kernel_size=(window_size, 1), padding=(padding, 0), stride=(2, 1)) # [1, C * window_size, M / 2 + 1]
|
|
||||||
unfold_feats = unfold_feats.view(self.audio_dim, window_size, -1).permute(2, 1, 0).contiguous() # [C, window_size, M / 2 + 1] --> [M / 2 + 1, window_size, C]
|
|
||||||
# print('[INFO] after unfold', unfold_feats.shape)
|
|
||||||
# save to a npy file
|
|
||||||
if 'esperanto' in self.opt.asr_model:
|
|
||||||
output_path = self.opt.asr_wav.replace('.wav', '_eo.npy')
|
|
||||||
else:
|
|
||||||
output_path = self.opt.asr_wav.replace('.wav', '.npy')
|
|
||||||
np.save(output_path, unfold_feats.cpu().numpy())
|
|
||||||
print(f"[INFO] saved logits to {output_path}")
|
|
||||||
|
|
||||||
'''
|
|
||||||
def create_file_stream(self):
|
|
||||||
|
|
||||||
stream, sample_rate = sf.read(self.opt.asr_wav) # [T*sample_rate,] float64
|
|
||||||
stream = stream.astype(np.float32)
|
|
||||||
|
|
||||||
if stream.ndim > 1:
|
|
||||||
print(f'[WARN] audio has {stream.shape[1]} channels, only use the first.')
|
|
||||||
stream = stream[:, 0]
|
|
||||||
|
|
||||||
if sample_rate != self.sample_rate:
|
|
||||||
print(f'[WARN] audio sample rate is {sample_rate}, resampling into {self.sample_rate}.')
|
|
||||||
stream = resampy.resample(x=stream, sr_orig=sample_rate, sr_new=self.sample_rate)
|
|
||||||
|
|
||||||
print(f'[INFO] loaded audio stream {self.opt.asr_wav}: {stream.shape}')
|
|
||||||
|
|
||||||
return stream
|
|
||||||
|
|
||||||
|
|
||||||
def create_pyaudio_stream(self):
|
|
||||||
|
|
||||||
import pyaudio
|
|
||||||
|
|
||||||
print(f'[INFO] creating live audio stream ...')
|
|
||||||
|
|
||||||
audio = pyaudio.PyAudio()
|
|
||||||
|
|
||||||
# get devices
|
|
||||||
info = audio.get_host_api_info_by_index(0)
|
|
||||||
n_devices = info.get('deviceCount')
|
|
||||||
|
|
||||||
for i in range(0, n_devices):
|
|
||||||
if (audio.get_device_info_by_host_api_device_index(0, i).get('maxInputChannels')) > 0:
|
|
||||||
name = audio.get_device_info_by_host_api_device_index(0, i).get('name')
|
|
||||||
print(f'[INFO] choose audio device {name}, id {i}')
|
|
||||||
break
|
|
||||||
|
|
||||||
# get stream
|
|
||||||
stream = audio.open(input_device_index=i,
|
|
||||||
format=pyaudio.paInt16,
|
|
||||||
channels=1,
|
|
||||||
rate=self.sample_rate,
|
|
||||||
input=True,
|
|
||||||
frames_per_buffer=self.chunk)
|
|
||||||
|
|
||||||
return audio, stream
|
|
||||||
'''
|
|
||||||
|
|
||||||
def get_audio_frame(self):
|
|
||||||
|
|
||||||
if self.inwarm: # warm up
|
|
||||||
return np.zeros(self.chunk, dtype=np.float32)
|
|
||||||
|
|
||||||
if self.mode == 'file':
|
|
||||||
|
|
||||||
if self.idx < self.file_stream.shape[0]:
|
|
||||||
frame = self.file_stream[self.idx: self.idx + self.chunk]
|
|
||||||
self.idx = self.idx + self.chunk
|
|
||||||
return frame
|
|
||||||
else:
|
|
||||||
return None
|
|
||||||
|
|
||||||
else:
|
|
||||||
try:
|
|
||||||
frame = self.queue.get(block=False)
|
|
||||||
print(f'[INFO] get frame {frame.shape}')
|
|
||||||
except queue.Empty:
|
|
||||||
frame = np.zeros(self.chunk, dtype=np.float32)
|
|
||||||
|
|
||||||
self.idx = self.idx + self.chunk
|
|
||||||
|
|
||||||
return frame
|
|
||||||
|
|
||||||
|
|
||||||
def frame_to_text(self, frame):
|
|
||||||
# frame: [N * 320], N = (context_size + 2 * stride_size)
|
|
||||||
|
|
||||||
inputs = self.processor(frame, sampling_rate=self.sample_rate, return_tensors="pt", padding=True)
|
|
||||||
|
|
||||||
with torch.no_grad():
|
|
||||||
result = self.model(inputs.input_values.to(self.device))
|
|
||||||
if 'hubert' in self.opt.asr_model:
|
|
||||||
logits = result.last_hidden_state # [B=1, T=pts//320, hid=1024]
|
|
||||||
else:
|
|
||||||
logits = result.logits # [1, N - 1, 32]
|
|
||||||
#print('logits.shape:',logits.shape)
|
|
||||||
|
|
||||||
# cut off stride
|
|
||||||
left = max(0, self.stride_left_size)
|
|
||||||
right = min(logits.shape[1], logits.shape[1] - self.stride_right_size + 1) # +1 to make sure output is the same length as input.
|
|
||||||
|
|
||||||
# do not cut right if terminated.
|
|
||||||
if self.terminated:
|
|
||||||
right = logits.shape[1]
|
|
||||||
|
|
||||||
logits = logits[:, left:right]
|
|
||||||
|
|
||||||
# print(frame.shape, inputs.input_values.shape, logits.shape)
|
|
||||||
|
|
||||||
#predicted_ids = torch.argmax(logits, dim=-1)
|
|
||||||
#transcription = self.processor.batch_decode(predicted_ids)[0].lower()
|
|
||||||
|
|
||||||
|
|
||||||
# for esperanto
|
|
||||||
# labels = np.array(['ŭ', '»', 'c', 'ĵ', 'ñ', '”', '„', '“', 'ǔ', 'o', 'ĝ', 'm', 'k', 'd', 'a', 'ŝ', 'z', 'i', '«', '—', '‘', 'ĥ', 'f', 'y', 'h', 'j', '|', 'r', 'u', 'ĉ', 's', '–', 'fi', 'l', 'p', '’', 'g', 'v', 't', 'b', 'n', 'e', '[UNK]', '[PAD]'])
|
|
||||||
|
|
||||||
# labels = np.array([' ', ' ', ' ', '-', '|', 'E', 'T', 'A', 'O', 'N', 'I', 'H', 'S', 'R', 'D', 'L', 'U', 'M', 'W', 'C', 'F', 'G', 'Y', 'P', 'B', 'V', 'K', "'", 'X', 'J', 'Q', 'Z'])
|
|
||||||
# print(''.join(labels[predicted_ids[0].detach().cpu().long().numpy()]))
|
|
||||||
# print(predicted_ids[0])
|
|
||||||
# print(transcription)
|
|
||||||
|
|
||||||
return logits[0], None,None #predicted_ids[0], transcription # [N,]
|
|
||||||
|
|
||||||
def create_bytes_stream(self,byte_stream):
|
|
||||||
#byte_stream=BytesIO(buffer)
|
|
||||||
stream, sample_rate = sf.read(byte_stream) # [T*sample_rate,] float64
|
|
||||||
print(f'[INFO]tts audio stream {sample_rate}: {stream.shape}')
|
|
||||||
stream = stream.astype(np.float32)
|
|
||||||
|
|
||||||
if stream.ndim > 1:
|
|
||||||
print(f'[WARN] audio has {stream.shape[1]} channels, only use the first.')
|
|
||||||
stream = stream[:, 0]
|
|
||||||
|
|
||||||
if sample_rate != self.sample_rate and stream.shape[0]>0:
|
|
||||||
print(f'[WARN] audio sample rate is {sample_rate}, resampling into {self.sample_rate}.')
|
|
||||||
stream = resampy.resample(x=stream, sr_orig=sample_rate, sr_new=self.sample_rate)
|
|
||||||
|
|
||||||
return stream
|
|
||||||
|
|
||||||
def push_audio(self,buffer):
|
|
||||||
print(f'[INFO] push_audio {len(buffer)}')
|
|
||||||
if self.opt.tts == "xtts":
|
|
||||||
if len(buffer)>0:
|
|
||||||
stream = np.frombuffer(buffer, dtype=np.int16).astype(np.float32) / 32767
|
|
||||||
stream = resampy.resample(x=stream, sr_orig=24000, sr_new=self.sample_rate)
|
|
||||||
#byte_stream=BytesIO(buffer)
|
|
||||||
#stream = self.create_bytes_stream(byte_stream)
|
|
||||||
streamlen = stream.shape[0]
|
|
||||||
idx=0
|
|
||||||
while streamlen >= self.chunk:
|
|
||||||
self.queue.put(stream[idx:idx+self.chunk])
|
|
||||||
streamlen -= self.chunk
|
|
||||||
idx += self.chunk
|
|
||||||
# if streamlen>0: #skip last frame(not 20ms)
|
|
||||||
# self.queue.put(stream[idx:])
|
|
||||||
else: #edge tts
|
|
||||||
self.input_stream.write(buffer)
|
|
||||||
if len(buffer)<=0:
|
|
||||||
self.input_stream.seek(0)
|
|
||||||
stream = self.create_bytes_stream(self.input_stream)
|
|
||||||
streamlen = stream.shape[0]
|
|
||||||
idx=0
|
|
||||||
while streamlen >= self.chunk:
|
|
||||||
self.queue.put(stream[idx:idx+self.chunk])
|
|
||||||
streamlen -= self.chunk
|
|
||||||
idx += self.chunk
|
|
||||||
#if streamlen>0: #skip last frame(not 20ms)
|
|
||||||
# self.queue.put(stream[idx:])
|
|
||||||
self.input_stream.seek(0)
|
|
||||||
self.input_stream.truncate()
|
|
||||||
|
|
||||||
def get_audio_out(self):
|
|
||||||
return self.output_queue.get()
|
|
||||||
|
|
||||||
def run(self):
|
|
||||||
|
|
||||||
self.listen()
|
|
||||||
|
|
||||||
while not self.terminated:
|
|
||||||
self.run_step()
|
|
||||||
|
|
||||||
def clear_queue(self):
|
|
||||||
# clear the queue, to reduce potential latency...
|
|
||||||
print(f'[INFO] clear queue')
|
|
||||||
if self.mode == 'live':
|
|
||||||
self.queue.queue.clear()
|
|
||||||
if self.play:
|
|
||||||
self.output_queue.queue.clear()
|
|
||||||
|
|
||||||
def warm_up(self):
|
|
||||||
|
|
||||||
#self.listen()
|
|
||||||
|
|
||||||
self.inwarm = True
|
|
||||||
print(f'[INFO] warm up ASR live model, expected latency = {self.warm_up_steps / self.fps:.6f}s')
|
|
||||||
t = time.time()
|
|
||||||
for _ in range(self.warm_up_steps):
|
|
||||||
self.run_step()
|
|
||||||
if torch.cuda.is_available():
|
|
||||||
torch.cuda.synchronize()
|
|
||||||
t = time.time() - t
|
|
||||||
print(f'[INFO] warm-up done, actual latency = {t:.6f}s')
|
|
||||||
self.inwarm = False
|
|
||||||
|
|
||||||
#self.clear_queue()
|
|
||||||
|
|
||||||
|
|
||||||
|
|
||||||
|
|
||||||
if __name__ == '__main__':
|
|
||||||
import argparse
|
|
||||||
|
|
||||||
parser = argparse.ArgumentParser()
|
|
||||||
parser.add_argument('--wav', type=str, default='')
|
|
||||||
parser.add_argument('--play', action='store_true', help="play out the audio")
|
|
||||||
|
|
||||||
parser.add_argument('--model', type=str, default='cpierse/wav2vec2-large-xlsr-53-esperanto')
|
|
||||||
# parser.add_argument('--model', type=str, default='facebook/wav2vec2-large-960h-lv60-self')
|
|
||||||
|
|
||||||
parser.add_argument('--save_feats', action='store_true')
|
|
||||||
# audio FPS
|
|
||||||
parser.add_argument('--fps', type=int, default=50)
|
|
||||||
# sliding window left-middle-right length.
|
|
||||||
parser.add_argument('-l', type=int, default=10)
|
|
||||||
parser.add_argument('-m', type=int, default=50)
|
|
||||||
parser.add_argument('-r', type=int, default=10)
|
|
||||||
|
|
||||||
opt = parser.parse_args()
|
|
||||||
|
|
||||||
# fix
|
|
||||||
opt.asr_wav = opt.wav
|
|
||||||
opt.asr_play = opt.play
|
|
||||||
opt.asr_model = opt.model
|
|
||||||
opt.asr_save_feats = opt.save_feats
|
|
||||||
|
|
||||||
if 'deepspeech' in opt.asr_model:
|
|
||||||
raise ValueError("DeepSpeech features should not use this code to extract...")
|
|
||||||
|
|
||||||
with ASR(opt) as asr:
|
|
||||||
asr.run()
|
|
|
@ -0,0 +1,65 @@
|
||||||
|
1. pytorch3d安装不成功\
|
||||||
|
下载源码编译
|
||||||
|
|
||||||
|
```bash
|
||||||
|
git clone https://github.com/facebookresearch/pytorch3d.git
|
||||||
|
python setup.py install
|
||||||
|
```
|
||||||
|
|
||||||
|
2. websocket连接报错\
|
||||||
|
修改python/site-packages/flask\_sockets.py
|
||||||
|
|
||||||
|
```python
|
||||||
|
self.url_map.add(Rule(rule, endpoint=f)) 改成
|
||||||
|
self.url_map.add(Rule(rule, endpoint=f, websocket=True))
|
||||||
|
```
|
||||||
|
|
||||||
|
3. protobuf版本过高
|
||||||
|
|
||||||
|
```bash
|
||||||
|
pip uninstall protobuf
|
||||||
|
pip install protobuf==3.20.1
|
||||||
|
```
|
||||||
|
|
||||||
|
4. 数字人不眨眼\
|
||||||
|
训练模型时添加如下步骤
|
||||||
|
|
||||||
|
> Obtain AU45 for eyes blinking.\
|
||||||
|
> Run FeatureExtraction in OpenFace, rename and move the output CSV file to data/\<ID>/au.csv.
|
||||||
|
|
||||||
|
将au.csv拷到本项目的data目录下
|
||||||
|
|
||||||
|
5. 数字人添加背景图片
|
||||||
|
|
||||||
|
```bash
|
||||||
|
python app.py --bg_img bc.jpg
|
||||||
|
```
|
||||||
|
|
||||||
|
6. 用自己训练的模型报错维度不匹配\
|
||||||
|
训练模型时用wav2vec提取音频特征
|
||||||
|
|
||||||
|
```bash
|
||||||
|
python main.py data/ --workspace workspace/ -O --iters 100000 --asr_model cpierse/wav2vec2-large-xlsr-53-esperanto
|
||||||
|
```
|
||||||
|
|
||||||
|
7. rtmp推流时ffmpeg版本不对
|
||||||
|
网上版友反馈是需要4.2.2版本。我也不确定具体哪些版本不行。原则是运行一下ffmpeg,打印的信息里需要有libx264,如果没有肯定不行
|
||||||
|
```
|
||||||
|
--enable-libx264
|
||||||
|
```
|
||||||
|
8. 替换自己训练的模型
|
||||||
|
```python
|
||||||
|
.
|
||||||
|
├── data
|
||||||
|
│ ├── data_kf.json (对应训练数据中的transforms_train.json)
|
||||||
|
│ ├── au.csv
|
||||||
|
│ ├── pretrained
|
||||||
|
│ └── └── ngp_kf.pth (对应训练后的模型ngp_ep00xx.pth)
|
||||||
|
|
||||||
|
```
|
||||||
|
|
||||||
|
|
||||||
|
其他参考
|
||||||
|
https://github.com/lipku/metahuman-stream/issues/43#issuecomment-2008930101
|
||||||
|
|
||||||
|
|
|
@ -0,0 +1,69 @@
|
||||||
|
import time
|
||||||
|
import numpy as np
|
||||||
|
|
||||||
|
import queue
|
||||||
|
from queue import Queue
|
||||||
|
import multiprocessing as mp
|
||||||
|
|
||||||
|
|
||||||
|
class BaseASR:
|
||||||
|
def __init__(self, opt, parent=None):
|
||||||
|
self.opt = opt
|
||||||
|
self.parent = parent
|
||||||
|
|
||||||
|
self.fps = opt.fps # 20 ms per frame
|
||||||
|
self.sample_rate = 16000
|
||||||
|
self.chunk = self.sample_rate // self.fps # 320 samples per chunk (20ms * 16000 / 1000)
|
||||||
|
self.queue = Queue()
|
||||||
|
self.output_queue = mp.Queue()
|
||||||
|
|
||||||
|
self.batch_size = opt.batch_size
|
||||||
|
|
||||||
|
self.frames = []
|
||||||
|
self.stride_left_size = opt.l
|
||||||
|
self.stride_right_size = opt.r
|
||||||
|
#self.context_size = 10
|
||||||
|
self.feat_queue = mp.Queue(2)
|
||||||
|
|
||||||
|
#self.warm_up()
|
||||||
|
|
||||||
|
def pause_talk(self):
|
||||||
|
self.queue.queue.clear()
|
||||||
|
|
||||||
|
def put_audio_frame(self,audio_chunk): #16khz 20ms pcm
|
||||||
|
self.queue.put(audio_chunk)
|
||||||
|
|
||||||
|
def get_audio_frame(self):
|
||||||
|
try:
|
||||||
|
frame = self.queue.get(block=True,timeout=0.01)
|
||||||
|
type = 0
|
||||||
|
#print(f'[INFO] get frame {frame.shape}')
|
||||||
|
except queue.Empty:
|
||||||
|
if self.parent and self.parent.curr_state>1: #播放自定义音频
|
||||||
|
frame = self.parent.get_audio_stream(self.parent.curr_state)
|
||||||
|
type = self.parent.curr_state
|
||||||
|
else:
|
||||||
|
frame = np.zeros(self.chunk, dtype=np.float32)
|
||||||
|
type = 1
|
||||||
|
|
||||||
|
return frame,type
|
||||||
|
|
||||||
|
def is_audio_frame_empty(self)->bool:
|
||||||
|
return self.queue.empty()
|
||||||
|
|
||||||
|
def get_audio_out(self): #get origin audio pcm to nerf
|
||||||
|
return self.output_queue.get()
|
||||||
|
|
||||||
|
def warm_up(self):
|
||||||
|
for _ in range(self.stride_left_size + self.stride_right_size):
|
||||||
|
audio_frame,type=self.get_audio_frame()
|
||||||
|
self.frames.append(audio_frame)
|
||||||
|
self.output_queue.put((audio_frame,type))
|
||||||
|
for _ in range(self.stride_left_size):
|
||||||
|
self.output_queue.get()
|
||||||
|
|
||||||
|
def run_step(self):
|
||||||
|
pass
|
||||||
|
|
||||||
|
def get_next_feat(self,block,timeout):
|
||||||
|
return self.feat_queue.get(block,timeout)
|
|
@ -0,0 +1,207 @@
|
||||||
|
import math
|
||||||
|
import torch
|
||||||
|
import numpy as np
|
||||||
|
|
||||||
|
import os
|
||||||
|
import time
|
||||||
|
import cv2
|
||||||
|
import glob
|
||||||
|
import pickle
|
||||||
|
import copy
|
||||||
|
import resampy
|
||||||
|
|
||||||
|
import queue
|
||||||
|
from queue import Queue
|
||||||
|
from threading import Thread, Event
|
||||||
|
from io import BytesIO
|
||||||
|
import soundfile as sf
|
||||||
|
|
||||||
|
import av
|
||||||
|
from fractions import Fraction
|
||||||
|
|
||||||
|
from ttsreal import EdgeTTS,VoitsTTS,XTTS,CosyVoiceTTS
|
||||||
|
|
||||||
|
from tqdm import tqdm
|
||||||
|
def read_imgs(img_list):
|
||||||
|
frames = []
|
||||||
|
print('reading images...')
|
||||||
|
for img_path in tqdm(img_list):
|
||||||
|
frame = cv2.imread(img_path)
|
||||||
|
frames.append(frame)
|
||||||
|
return frames
|
||||||
|
|
||||||
|
class BaseReal:
|
||||||
|
def __init__(self, opt):
|
||||||
|
self.opt = opt
|
||||||
|
self.sample_rate = 16000
|
||||||
|
self.chunk = self.sample_rate // opt.fps # 320 samples per chunk (20ms * 16000 / 1000)
|
||||||
|
|
||||||
|
if opt.tts == "edgetts":
|
||||||
|
self.tts = EdgeTTS(opt,self)
|
||||||
|
elif opt.tts == "gpt-sovits":
|
||||||
|
self.tts = VoitsTTS(opt,self)
|
||||||
|
elif opt.tts == "xtts":
|
||||||
|
self.tts = XTTS(opt,self)
|
||||||
|
elif opt.tts == "cosyvoice":
|
||||||
|
self.tts = CosyVoiceTTS(opt,self)
|
||||||
|
|
||||||
|
self.speaking = False
|
||||||
|
|
||||||
|
self.recording = False
|
||||||
|
self.recordq_video = Queue()
|
||||||
|
self.recordq_audio = Queue()
|
||||||
|
|
||||||
|
self.curr_state=0
|
||||||
|
self.custom_img_cycle = {}
|
||||||
|
self.custom_audio_cycle = {}
|
||||||
|
self.custom_audio_index = {}
|
||||||
|
self.custom_index = {}
|
||||||
|
self.custom_opt = {}
|
||||||
|
self.__loadcustom()
|
||||||
|
|
||||||
|
def put_msg_txt(self,msg):
|
||||||
|
self.tts.put_msg_txt(msg)
|
||||||
|
|
||||||
|
def put_audio_frame(self,audio_chunk): #16khz 20ms pcm
|
||||||
|
self.asr.put_audio_frame(audio_chunk)
|
||||||
|
|
||||||
|
def put_audio_file(self,filebyte):
|
||||||
|
input_stream = BytesIO(filebyte)
|
||||||
|
stream = self.__create_bytes_stream(input_stream)
|
||||||
|
streamlen = stream.shape[0]
|
||||||
|
idx=0
|
||||||
|
while streamlen >= self.chunk: #and self.state==State.RUNNING
|
||||||
|
self.put_audio_frame(stream[idx:idx+self.chunk])
|
||||||
|
streamlen -= self.chunk
|
||||||
|
idx += self.chunk
|
||||||
|
|
||||||
|
def __create_bytes_stream(self,byte_stream):
|
||||||
|
#byte_stream=BytesIO(buffer)
|
||||||
|
stream, sample_rate = sf.read(byte_stream) # [T*sample_rate,] float64
|
||||||
|
print(f'[INFO]put audio stream {sample_rate}: {stream.shape}')
|
||||||
|
stream = stream.astype(np.float32)
|
||||||
|
|
||||||
|
if stream.ndim > 1:
|
||||||
|
print(f'[WARN] audio has {stream.shape[1]} channels, only use the first.')
|
||||||
|
stream = stream[:, 0]
|
||||||
|
|
||||||
|
if sample_rate != self.sample_rate and stream.shape[0]>0:
|
||||||
|
print(f'[WARN] audio sample rate is {sample_rate}, resampling into {self.sample_rate}.')
|
||||||
|
stream = resampy.resample(x=stream, sr_orig=sample_rate, sr_new=self.sample_rate)
|
||||||
|
|
||||||
|
return stream
|
||||||
|
|
||||||
|
def pause_talk(self):
|
||||||
|
self.tts.pause_talk()
|
||||||
|
self.asr.pause_talk()
|
||||||
|
|
||||||
|
def is_speaking(self)->bool:
|
||||||
|
return self.speaking
|
||||||
|
|
||||||
|
def __loadcustom(self):
|
||||||
|
for item in self.opt.customopt:
|
||||||
|
print(item)
|
||||||
|
input_img_list = glob.glob(os.path.join(item['imgpath'], '*.[jpJP][pnPN]*[gG]'))
|
||||||
|
input_img_list = sorted(input_img_list, key=lambda x: int(os.path.splitext(os.path.basename(x))[0]))
|
||||||
|
self.custom_img_cycle[item['audiotype']] = read_imgs(input_img_list)
|
||||||
|
self.custom_audio_cycle[item['audiotype']], sample_rate = sf.read(item['audiopath'], dtype='float32')
|
||||||
|
self.custom_audio_index[item['audiotype']] = 0
|
||||||
|
self.custom_index[item['audiotype']] = 0
|
||||||
|
self.custom_opt[item['audiotype']] = item
|
||||||
|
|
||||||
|
def init_customindex(self):
|
||||||
|
self.curr_state=0
|
||||||
|
for key in self.custom_audio_index:
|
||||||
|
self.custom_audio_index[key]=0
|
||||||
|
for key in self.custom_index:
|
||||||
|
self.custom_index[key]=0
|
||||||
|
|
||||||
|
def start_recording(self,path):
|
||||||
|
"""开始录制视频"""
|
||||||
|
if self.recording:
|
||||||
|
return
|
||||||
|
self.recording = True
|
||||||
|
self.recordq_video.queue.clear()
|
||||||
|
self.recordq_audio.queue.clear()
|
||||||
|
self.container = av.open(path, mode="w")
|
||||||
|
|
||||||
|
process_thread = Thread(target=self.record_frame, args=())
|
||||||
|
process_thread.start()
|
||||||
|
|
||||||
|
def record_frame(self):
|
||||||
|
videostream = self.container.add_stream("libx264", rate=25)
|
||||||
|
videostream.codec_context.time_base = Fraction(1, 25)
|
||||||
|
audiostream = self.container.add_stream("aac")
|
||||||
|
audiostream.codec_context.time_base = Fraction(1, 16000)
|
||||||
|
init = True
|
||||||
|
framenum = 0
|
||||||
|
while self.recording:
|
||||||
|
try:
|
||||||
|
videoframe = self.recordq_video.get(block=True, timeout=1)
|
||||||
|
videoframe.pts = framenum #int(round(framenum*0.04 / videostream.codec_context.time_base))
|
||||||
|
videoframe.dts = videoframe.pts
|
||||||
|
if init:
|
||||||
|
videostream.width = videoframe.width
|
||||||
|
videostream.height = videoframe.height
|
||||||
|
init = False
|
||||||
|
for packet in videostream.encode(videoframe):
|
||||||
|
self.container.mux(packet)
|
||||||
|
for k in range(2):
|
||||||
|
audioframe = self.recordq_audio.get(block=True, timeout=1)
|
||||||
|
audioframe.pts = int(round((framenum*2+k)*0.02 / audiostream.codec_context.time_base))
|
||||||
|
audioframe.dts = audioframe.pts
|
||||||
|
for packet in audiostream.encode(audioframe):
|
||||||
|
self.container.mux(packet)
|
||||||
|
framenum += 1
|
||||||
|
except queue.Empty:
|
||||||
|
print('record queue empty,')
|
||||||
|
continue
|
||||||
|
except Exception as e:
|
||||||
|
print(e)
|
||||||
|
#break
|
||||||
|
for packet in videostream.encode(None):
|
||||||
|
self.container.mux(packet)
|
||||||
|
for packet in audiostream.encode(None):
|
||||||
|
self.container.mux(packet)
|
||||||
|
self.container.close()
|
||||||
|
self.recordq_video.queue.clear()
|
||||||
|
self.recordq_audio.queue.clear()
|
||||||
|
print('record thread stop')
|
||||||
|
|
||||||
|
def stop_recording(self):
|
||||||
|
"""停止录制视频"""
|
||||||
|
if not self.recording:
|
||||||
|
return
|
||||||
|
self.recording = False
|
||||||
|
|
||||||
|
def mirror_index(self,size, index):
|
||||||
|
#size = len(self.coord_list_cycle)
|
||||||
|
turn = index // size
|
||||||
|
res = index % size
|
||||||
|
if turn % 2 == 0:
|
||||||
|
return res
|
||||||
|
else:
|
||||||
|
return size - res - 1
|
||||||
|
|
||||||
|
def get_audio_stream(self,audiotype):
|
||||||
|
idx = self.custom_audio_index[audiotype]
|
||||||
|
stream = self.custom_audio_cycle[audiotype][idx:idx+self.chunk]
|
||||||
|
self.custom_audio_index[audiotype] += self.chunk
|
||||||
|
if self.custom_audio_index[audiotype]>=self.custom_audio_cycle[audiotype].shape[0]:
|
||||||
|
self.curr_state = 1 #当前视频不循环播放,切换到静音状态
|
||||||
|
return stream
|
||||||
|
|
||||||
|
def set_curr_state(self,audiotype, reinit):
|
||||||
|
print('set_curr_state:',audiotype)
|
||||||
|
self.curr_state = audiotype
|
||||||
|
if reinit:
|
||||||
|
self.custom_audio_index[audiotype] = 0
|
||||||
|
self.custom_index[audiotype] = 0
|
||||||
|
|
||||||
|
# def process_custom(self,audiotype:int,idx:int):
|
||||||
|
# if self.curr_state!=audiotype: #从推理切到口播
|
||||||
|
# if idx in self.switch_pos: #在卡点位置可以切换
|
||||||
|
# self.curr_state=audiotype
|
||||||
|
# self.custom_index=0
|
||||||
|
# else:
|
||||||
|
# self.custom_index+=1
|
|
@ -0,0 +1,7 @@
|
||||||
|
[
|
||||||
|
{
|
||||||
|
"audiotype":2,
|
||||||
|
"imgpath":"data/customvideo/image",
|
||||||
|
"audiopath":"data/customvideo/audio.wav"
|
||||||
|
}
|
||||||
|
]
|
|
@ -13,23 +13,23 @@ def get_encoder(encoding, input_dim=3,
|
||||||
return lambda x, **kwargs: x, input_dim
|
return lambda x, **kwargs: x, input_dim
|
||||||
|
|
||||||
elif encoding == 'frequency':
|
elif encoding == 'frequency':
|
||||||
from freqencoder import FreqEncoder
|
from .freqencoder import FreqEncoder
|
||||||
encoder = FreqEncoder(input_dim=input_dim, degree=multires)
|
encoder = FreqEncoder(input_dim=input_dim, degree=multires)
|
||||||
|
|
||||||
elif encoding == 'spherical_harmonics':
|
elif encoding == 'spherical_harmonics':
|
||||||
from shencoder import SHEncoder
|
from .shencoder import SHEncoder
|
||||||
encoder = SHEncoder(input_dim=input_dim, degree=degree)
|
encoder = SHEncoder(input_dim=input_dim, degree=degree)
|
||||||
|
|
||||||
elif encoding == 'hashgrid':
|
elif encoding == 'hashgrid':
|
||||||
from gridencoder import GridEncoder
|
from .gridencoder import GridEncoder
|
||||||
encoder = GridEncoder(input_dim=input_dim, num_levels=num_levels, level_dim=level_dim, base_resolution=base_resolution, log2_hashmap_size=log2_hashmap_size, desired_resolution=desired_resolution, gridtype='hash', align_corners=align_corners)
|
encoder = GridEncoder(input_dim=input_dim, num_levels=num_levels, level_dim=level_dim, base_resolution=base_resolution, log2_hashmap_size=log2_hashmap_size, desired_resolution=desired_resolution, gridtype='hash', align_corners=align_corners)
|
||||||
|
|
||||||
elif encoding == 'tiledgrid':
|
elif encoding == 'tiledgrid':
|
||||||
from gridencoder import GridEncoder
|
from .gridencoder import GridEncoder
|
||||||
encoder = GridEncoder(input_dim=input_dim, num_levels=num_levels, level_dim=level_dim, base_resolution=base_resolution, log2_hashmap_size=log2_hashmap_size, desired_resolution=desired_resolution, gridtype='tiled', align_corners=align_corners)
|
encoder = GridEncoder(input_dim=input_dim, num_levels=num_levels, level_dim=level_dim, base_resolution=base_resolution, log2_hashmap_size=log2_hashmap_size, desired_resolution=desired_resolution, gridtype='tiled', align_corners=align_corners)
|
||||||
|
|
||||||
elif encoding == 'ash':
|
elif encoding == 'ash':
|
||||||
from ashencoder import AshEncoder
|
from .ashencoder import AshEncoder
|
||||||
encoder = AshEncoder(input_dim=input_dim, output_dim=16, log2_hashmap_size=log2_hashmap_size, resolution=desired_resolution)
|
encoder = AshEncoder(input_dim=input_dim, output_dim=16, log2_hashmap_size=log2_hashmap_size, resolution=desired_resolution)
|
||||||
|
|
||||||
else:
|
else:
|
|
@ -4,13 +4,13 @@ from torch.utils.cpp_extension import load
|
||||||
_src_path = os.path.dirname(os.path.abspath(__file__))
|
_src_path = os.path.dirname(os.path.abspath(__file__))
|
||||||
|
|
||||||
nvcc_flags = [
|
nvcc_flags = [
|
||||||
'-O3', '-std=c++14',
|
'-O3', '-std=c++17',
|
||||||
'-U__CUDA_NO_HALF_OPERATORS__', '-U__CUDA_NO_HALF_CONVERSIONS__', '-U__CUDA_NO_HALF2_OPERATORS__',
|
'-U__CUDA_NO_HALF_OPERATORS__', '-U__CUDA_NO_HALF_CONVERSIONS__', '-U__CUDA_NO_HALF2_OPERATORS__', '-allow-unsupported-compiler',
|
||||||
'-use_fast_math'
|
'-use_fast_math'
|
||||||
]
|
]
|
||||||
|
|
||||||
if os.name == "posix":
|
if os.name == "posix":
|
||||||
c_flags = ['-O3', '-std=c++14']
|
c_flags = ['-O3', '-std=c++17']
|
||||||
elif os.name == "nt":
|
elif os.name == "nt":
|
||||||
c_flags = ['/O2', '/std:c++17']
|
c_flags = ['/O2', '/std:c++17']
|
||||||
|
|
|
@ -5,13 +5,13 @@ from torch.utils.cpp_extension import BuildExtension, CUDAExtension
|
||||||
_src_path = os.path.dirname(os.path.abspath(__file__))
|
_src_path = os.path.dirname(os.path.abspath(__file__))
|
||||||
|
|
||||||
nvcc_flags = [
|
nvcc_flags = [
|
||||||
'-O3', '-std=c++14',
|
'-O3', '-std=c++17',
|
||||||
'-U__CUDA_NO_HALF_OPERATORS__', '-U__CUDA_NO_HALF_CONVERSIONS__', '-U__CUDA_NO_HALF2_OPERATORS__',
|
'-U__CUDA_NO_HALF_OPERATORS__', '-U__CUDA_NO_HALF_CONVERSIONS__', '-U__CUDA_NO_HALF2_OPERATORS__', '-allow-unsupported-compiler',
|
||||||
'-use_fast_math'
|
'-use_fast_math'
|
||||||
]
|
]
|
||||||
|
|
||||||
if os.name == "posix":
|
if os.name == "posix":
|
||||||
c_flags = ['-O3', '-std=c++14']
|
c_flags = ['-O3', '-std=c++17']
|
||||||
elif os.name == "nt":
|
elif os.name == "nt":
|
||||||
c_flags = ['/O2', '/std:c++17']
|
c_flags = ['/O2', '/std:c++17']
|
||||||
|
|
|
@ -4,12 +4,12 @@ from torch.utils.cpp_extension import load
|
||||||
_src_path = os.path.dirname(os.path.abspath(__file__))
|
_src_path = os.path.dirname(os.path.abspath(__file__))
|
||||||
|
|
||||||
nvcc_flags = [
|
nvcc_flags = [
|
||||||
'-O3', '-std=c++14',
|
'-O3', '-std=c++17',
|
||||||
'-U__CUDA_NO_HALF_OPERATORS__', '-U__CUDA_NO_HALF_CONVERSIONS__', '-U__CUDA_NO_HALF2_OPERATORS__',
|
'-U__CUDA_NO_HALF_OPERATORS__', '-U__CUDA_NO_HALF_CONVERSIONS__', '-U__CUDA_NO_HALF2_OPERATORS__',
|
||||||
]
|
]
|
||||||
|
|
||||||
if os.name == "posix":
|
if os.name == "posix":
|
||||||
c_flags = ['-O3', '-std=c++14', '-finput-charset=UTF-8']
|
c_flags = ['-O3', '-std=c++17', '-finput-charset=UTF-8']
|
||||||
elif os.name == "nt":
|
elif os.name == "nt":
|
||||||
c_flags = ['/O2', '/std:c++17', '/finput-charset=UTF-8']
|
c_flags = ['/O2', '/std:c++17', '/finput-charset=UTF-8']
|
||||||
|
|
|
@ -5,12 +5,12 @@ from torch.utils.cpp_extension import BuildExtension, CUDAExtension
|
||||||
_src_path = os.path.dirname(os.path.abspath(__file__))
|
_src_path = os.path.dirname(os.path.abspath(__file__))
|
||||||
|
|
||||||
nvcc_flags = [
|
nvcc_flags = [
|
||||||
'-O3', '-std=c++14',
|
'-O3', '-std=c++17',
|
||||||
'-U__CUDA_NO_HALF_OPERATORS__', '-U__CUDA_NO_HALF_CONVERSIONS__', '-U__CUDA_NO_HALF2_OPERATORS__',
|
'-U__CUDA_NO_HALF_OPERATORS__', '-U__CUDA_NO_HALF_CONVERSIONS__', '-U__CUDA_NO_HALF2_OPERATORS__','-allow-unsupported-compiler',
|
||||||
]
|
]
|
||||||
|
|
||||||
if os.name == "posix":
|
if os.name == "posix":
|
||||||
c_flags = ['-O3', '-std=c++14']
|
c_flags = ['-O3', '-std=c++17']
|
||||||
elif os.name == "nt":
|
elif os.name == "nt":
|
||||||
c_flags = ['/O2', '/std:c++17']
|
c_flags = ['/O2', '/std:c++17']
|
||||||
|
|
|
@ -1,9 +1,9 @@
|
||||||
import torch
|
import torch
|
||||||
import argparse
|
import argparse
|
||||||
|
|
||||||
from nerf_triplane.provider import NeRFDataset,NeRFDataset_Test
|
from .nerf_triplane.provider import NeRFDataset,NeRFDataset_Test
|
||||||
from nerf_triplane.utils import *
|
from .nerf_triplane.utils import *
|
||||||
from nerf_triplane.network import NeRFNetwork
|
from .nerf_triplane.network import NeRFNetwork
|
||||||
|
|
||||||
# torch.autograd.set_detect_anomaly(True)
|
# torch.autograd.set_detect_anomaly(True)
|
||||||
# Close tf32 features. Fix low numerical accuracy on rtx30xx gpu.
|
# Close tf32 features. Fix low numerical accuracy on rtx30xx gpu.
|
|
@ -2,7 +2,7 @@ import torch
|
||||||
import torch.nn as nn
|
import torch.nn as nn
|
||||||
import torch.nn.functional as F
|
import torch.nn.functional as F
|
||||||
|
|
||||||
from encoding import get_encoder
|
from ..encoding import get_encoder
|
||||||
from .renderer import NeRFRenderer
|
from .renderer import NeRFRenderer
|
||||||
|
|
||||||
# Audio feature extractor
|
# Audio feature extractor
|
|
@ -7,7 +7,7 @@ import torch
|
||||||
import torch.nn as nn
|
import torch.nn as nn
|
||||||
import torch.nn.functional as F
|
import torch.nn.functional as F
|
||||||
|
|
||||||
import raymarching
|
from .. import raymarching
|
||||||
from .utils import custom_meshgrid, get_audio_features, euler_angles_to_matrix, convert_poses
|
from .utils import custom_meshgrid, get_audio_features, euler_angles_to_matrix, convert_poses
|
||||||
|
|
||||||
def sample_pdf(bins, weights, n_samples, det=False):
|
def sample_pdf(bins, weights, n_samples, det=False):
|
|
@ -4,12 +4,12 @@ from torch.utils.cpp_extension import load
|
||||||
_src_path = os.path.dirname(os.path.abspath(__file__))
|
_src_path = os.path.dirname(os.path.abspath(__file__))
|
||||||
|
|
||||||
nvcc_flags = [
|
nvcc_flags = [
|
||||||
'-O3', '-std=c++14',
|
'-O3', '-std=c++17',
|
||||||
'-U__CUDA_NO_HALF_OPERATORS__', '-U__CUDA_NO_HALF_CONVERSIONS__', '-U__CUDA_NO_HALF2_OPERATORS__',
|
'-U__CUDA_NO_HALF_OPERATORS__', '-U__CUDA_NO_HALF_CONVERSIONS__', '-U__CUDA_NO_HALF2_OPERATORS__','-allow-unsupported-compiler',
|
||||||
]
|
]
|
||||||
|
|
||||||
if os.name == "posix":
|
if os.name == "posix":
|
||||||
c_flags = ['-O3', '-std=c++14']
|
c_flags = ['-O3', '-std=c++17']
|
||||||
elif os.name == "nt":
|
elif os.name == "nt":
|
||||||
c_flags = ['/O2', '/std:c++17']
|
c_flags = ['/O2', '/std:c++17']
|
||||||
|
|
|
@ -5,13 +5,13 @@ from torch.utils.cpp_extension import BuildExtension, CUDAExtension
|
||||||
_src_path = os.path.dirname(os.path.abspath(__file__))
|
_src_path = os.path.dirname(os.path.abspath(__file__))
|
||||||
|
|
||||||
nvcc_flags = [
|
nvcc_flags = [
|
||||||
'-O3', '-std=c++14',
|
'-O3', '-std=c++17',
|
||||||
# '-lineinfo', # to debug illegal memory access
|
# '-lineinfo', # to debug illegal memory access
|
||||||
'-U__CUDA_NO_HALF_OPERATORS__', '-U__CUDA_NO_HALF_CONVERSIONS__', '-U__CUDA_NO_HALF2_OPERATORS__',
|
'-U__CUDA_NO_HALF_OPERATORS__', '-U__CUDA_NO_HALF_CONVERSIONS__', '-U__CUDA_NO_HALF2_OPERATORS__','-allow-unsupported-compiler',
|
||||||
]
|
]
|
||||||
|
|
||||||
if os.name == "posix":
|
if os.name == "posix":
|
||||||
c_flags = ['-O3', '-std=c++14']
|
c_flags = ['-O3', '-std=c++17']
|
||||||
elif os.name == "nt":
|
elif os.name == "nt":
|
||||||
c_flags = ['/O2', '/std:c++17']
|
c_flags = ['/O2', '/std:c++17']
|
||||||
|
|
|
@ -4,12 +4,12 @@ from torch.utils.cpp_extension import load
|
||||||
_src_path = os.path.dirname(os.path.abspath(__file__))
|
_src_path = os.path.dirname(os.path.abspath(__file__))
|
||||||
|
|
||||||
nvcc_flags = [
|
nvcc_flags = [
|
||||||
'-O3', '-std=c++14',
|
'-O3', '-std=c++17',
|
||||||
'-U__CUDA_NO_HALF_OPERATORS__', '-U__CUDA_NO_HALF_CONVERSIONS__', '-U__CUDA_NO_HALF2_OPERATORS__',
|
'-U__CUDA_NO_HALF_OPERATORS__', '-U__CUDA_NO_HALF_CONVERSIONS__', '-U__CUDA_NO_HALF2_OPERATORS__','-allow-unsupported-compiler',
|
||||||
]
|
]
|
||||||
|
|
||||||
if os.name == "posix":
|
if os.name == "posix":
|
||||||
c_flags = ['-O3', '-std=c++14', '-finput-charset=utf-8']
|
c_flags = ['-O3', '-std=c++17', '-finput-charset=utf-8']
|
||||||
elif os.name == "nt":
|
elif os.name == "nt":
|
||||||
c_flags = ['/O2', '/std:c++17', '/source-charset:utf-8']
|
c_flags = ['/O2', '/std:c++17', '/source-charset:utf-8']
|
||||||
|
|
|
@ -5,12 +5,12 @@ from torch.utils.cpp_extension import BuildExtension, CUDAExtension
|
||||||
_src_path = os.path.dirname(os.path.abspath(__file__))
|
_src_path = os.path.dirname(os.path.abspath(__file__))
|
||||||
|
|
||||||
nvcc_flags = [
|
nvcc_flags = [
|
||||||
'-O3', '-std=c++14',
|
'-O3', '-std=c++17',
|
||||||
'-U__CUDA_NO_HALF_OPERATORS__', '-U__CUDA_NO_HALF_CONVERSIONS__', '-U__CUDA_NO_HALF2_OPERATORS__',
|
'-U__CUDA_NO_HALF_OPERATORS__', '-U__CUDA_NO_HALF_CONVERSIONS__', '-U__CUDA_NO_HALF2_OPERATORS__','-allow-unsupported-compiler',
|
||||||
]
|
]
|
||||||
|
|
||||||
if os.name == "posix":
|
if os.name == "posix":
|
||||||
c_flags = ['-O3', '-std=c++14']
|
c_flags = ['-O3', '-std=c++17']
|
||||||
elif os.name == "nt":
|
elif os.name == "nt":
|
||||||
c_flags = ['/O2', '/std:c++17']
|
c_flags = ['/O2', '/std:c++17']
|
||||||
|
|
|
@ -0,0 +1,14 @@
|
||||||
|
[INFO] Trainer: ngp | 2023-09-23_17-52-22 | cuda | fp16 | workspace
|
||||||
|
[INFO] #parameters: 1789121
|
||||||
|
[INFO] Loading data/pretrained/ngp_kf.pth ...
|
||||||
|
[INFO] Trainer: ngp | 2023-09-23_18-58-25 | cuda | fp16 | workspace
|
||||||
|
[INFO] #parameters: 1789121
|
||||||
|
[INFO] Loading data/pretrained/ngp_kf.pth ...
|
||||||
|
[INFO] Trainer: ngp | 2023-09-23_19-01-54 | cuda | fp16 | workspace
|
||||||
|
[INFO] #parameters: 1787681
|
||||||
|
[INFO] Loading data/pretrained/ngp_kf.pth ...
|
||||||
|
[INFO] loaded model.
|
||||||
|
[INFO] load at epoch 14, global step 51226
|
||||||
|
[WARN] Failed to load optimizer.
|
||||||
|
[INFO] loaded scheduler.
|
||||||
|
[INFO] loaded scaler.
|
|
@ -0,0 +1,47 @@
|
||||||
|
import time
|
||||||
|
import torch
|
||||||
|
import numpy as np
|
||||||
|
|
||||||
|
import queue
|
||||||
|
from queue import Queue
|
||||||
|
import multiprocessing as mp
|
||||||
|
|
||||||
|
from baseasr import BaseASR
|
||||||
|
from wav2lip import audio
|
||||||
|
|
||||||
|
class LipASR(BaseASR):
|
||||||
|
|
||||||
|
def run_step(self):
|
||||||
|
############################################## extract audio feature ##############################################
|
||||||
|
# get a frame of audio
|
||||||
|
for _ in range(self.batch_size*2):
|
||||||
|
frame,type = self.get_audio_frame()
|
||||||
|
self.frames.append(frame)
|
||||||
|
# put to output
|
||||||
|
self.output_queue.put((frame,type))
|
||||||
|
# context not enough, do not run network.
|
||||||
|
if len(self.frames) <= self.stride_left_size + self.stride_right_size:
|
||||||
|
return
|
||||||
|
|
||||||
|
inputs = np.concatenate(self.frames) # [N * chunk]
|
||||||
|
mel = audio.melspectrogram(inputs)
|
||||||
|
#print(mel.shape[0],mel.shape,len(mel[0]),len(self.frames))
|
||||||
|
# cut off stride
|
||||||
|
left = max(0, self.stride_left_size*80/50)
|
||||||
|
right = min(len(mel[0]), len(mel[0]) - self.stride_right_size*80/50)
|
||||||
|
mel_idx_multiplier = 80.*2/self.fps
|
||||||
|
mel_step_size = 16
|
||||||
|
i = 0
|
||||||
|
mel_chunks = []
|
||||||
|
while i < (len(self.frames)-self.stride_left_size-self.stride_right_size)/2:
|
||||||
|
start_idx = int(left + i * mel_idx_multiplier)
|
||||||
|
#print(start_idx)
|
||||||
|
if start_idx + mel_step_size > len(mel[0]):
|
||||||
|
mel_chunks.append(mel[:, len(mel[0]) - mel_step_size:])
|
||||||
|
else:
|
||||||
|
mel_chunks.append(mel[:, start_idx : start_idx + mel_step_size])
|
||||||
|
i += 1
|
||||||
|
self.feat_queue.put(mel_chunks)
|
||||||
|
|
||||||
|
# discard the old part to save memory
|
||||||
|
self.frames = self.frames[-(self.stride_left_size + self.stride_right_size):]
|
|
@ -0,0 +1,281 @@
|
||||||
|
import math
|
||||||
|
import torch
|
||||||
|
import numpy as np
|
||||||
|
|
||||||
|
#from .utils import *
|
||||||
|
import subprocess
|
||||||
|
import os
|
||||||
|
import time
|
||||||
|
import cv2
|
||||||
|
import glob
|
||||||
|
import pickle
|
||||||
|
import copy
|
||||||
|
|
||||||
|
import queue
|
||||||
|
from queue import Queue
|
||||||
|
from threading import Thread, Event
|
||||||
|
from io import BytesIO
|
||||||
|
import multiprocessing as mp
|
||||||
|
|
||||||
|
|
||||||
|
from ttsreal import EdgeTTS,VoitsTTS,XTTS
|
||||||
|
|
||||||
|
from lipasr import LipASR
|
||||||
|
import asyncio
|
||||||
|
from av import AudioFrame, VideoFrame
|
||||||
|
from wav2lip.models import Wav2Lip
|
||||||
|
from basereal import BaseReal
|
||||||
|
|
||||||
|
#from imgcache import ImgCache
|
||||||
|
|
||||||
|
from tqdm import tqdm
|
||||||
|
|
||||||
|
device = 'cuda' if torch.cuda.is_available() else 'cpu'
|
||||||
|
print('Using {} for inference.'.format(device))
|
||||||
|
|
||||||
|
def _load(checkpoint_path):
|
||||||
|
if device == 'cuda':
|
||||||
|
checkpoint = torch.load(checkpoint_path)
|
||||||
|
else:
|
||||||
|
checkpoint = torch.load(checkpoint_path,
|
||||||
|
map_location=lambda storage, loc: storage)
|
||||||
|
return checkpoint
|
||||||
|
|
||||||
|
def load_model(path):
|
||||||
|
model = Wav2Lip()
|
||||||
|
print("Load checkpoint from: {}".format(path))
|
||||||
|
checkpoint = _load(path)
|
||||||
|
s = checkpoint["state_dict"]
|
||||||
|
new_s = {}
|
||||||
|
for k, v in s.items():
|
||||||
|
new_s[k.replace('module.', '')] = v
|
||||||
|
model.load_state_dict(new_s)
|
||||||
|
|
||||||
|
model = model.to(device)
|
||||||
|
return model.eval()
|
||||||
|
|
||||||
|
def read_imgs(img_list):
|
||||||
|
frames = []
|
||||||
|
print('reading images...')
|
||||||
|
for img_path in tqdm(img_list):
|
||||||
|
frame = cv2.imread(img_path)
|
||||||
|
frames.append(frame)
|
||||||
|
return frames
|
||||||
|
|
||||||
|
def __mirror_index(size, index):
|
||||||
|
#size = len(self.coord_list_cycle)
|
||||||
|
turn = index // size
|
||||||
|
res = index % size
|
||||||
|
if turn % 2 == 0:
|
||||||
|
return res
|
||||||
|
else:
|
||||||
|
return size - res - 1
|
||||||
|
|
||||||
|
def inference(render_event,batch_size,face_imgs_path,audio_feat_queue,audio_out_queue,res_frame_queue):
|
||||||
|
|
||||||
|
model = load_model("./models/wav2lip.pth")
|
||||||
|
input_face_list = glob.glob(os.path.join(face_imgs_path, '*.[jpJP][pnPN]*[gG]'))
|
||||||
|
input_face_list = sorted(input_face_list, key=lambda x: int(os.path.splitext(os.path.basename(x))[0]))
|
||||||
|
face_list_cycle = read_imgs(input_face_list)
|
||||||
|
|
||||||
|
#input_latent_list_cycle = torch.load(latents_out_path)
|
||||||
|
length = len(face_list_cycle)
|
||||||
|
index = 0
|
||||||
|
count=0
|
||||||
|
counttime=0
|
||||||
|
print('start inference')
|
||||||
|
while True:
|
||||||
|
if render_event.is_set():
|
||||||
|
starttime=time.perf_counter()
|
||||||
|
mel_batch = []
|
||||||
|
try:
|
||||||
|
mel_batch = audio_feat_queue.get(block=True, timeout=1)
|
||||||
|
except queue.Empty:
|
||||||
|
continue
|
||||||
|
|
||||||
|
is_all_silence=True
|
||||||
|
audio_frames = []
|
||||||
|
for _ in range(batch_size*2):
|
||||||
|
frame,type = audio_out_queue.get()
|
||||||
|
audio_frames.append((frame,type))
|
||||||
|
if type==0:
|
||||||
|
is_all_silence=False
|
||||||
|
|
||||||
|
if is_all_silence:
|
||||||
|
for i in range(batch_size):
|
||||||
|
res_frame_queue.put((None,__mirror_index(length,index),audio_frames[i*2:i*2+2]))
|
||||||
|
index = index + 1
|
||||||
|
else:
|
||||||
|
# print('infer=======')
|
||||||
|
t=time.perf_counter()
|
||||||
|
img_batch = []
|
||||||
|
for i in range(batch_size):
|
||||||
|
idx = __mirror_index(length,index+i)
|
||||||
|
face = face_list_cycle[idx]
|
||||||
|
img_batch.append(face)
|
||||||
|
img_batch, mel_batch = np.asarray(img_batch), np.asarray(mel_batch)
|
||||||
|
|
||||||
|
img_masked = img_batch.copy()
|
||||||
|
img_masked[:, face.shape[0]//2:] = 0
|
||||||
|
|
||||||
|
img_batch = np.concatenate((img_masked, img_batch), axis=3) / 255.
|
||||||
|
mel_batch = np.reshape(mel_batch, [len(mel_batch), mel_batch.shape[1], mel_batch.shape[2], 1])
|
||||||
|
|
||||||
|
img_batch = torch.FloatTensor(np.transpose(img_batch, (0, 3, 1, 2))).to(device)
|
||||||
|
mel_batch = torch.FloatTensor(np.transpose(mel_batch, (0, 3, 1, 2))).to(device)
|
||||||
|
|
||||||
|
with torch.no_grad():
|
||||||
|
pred = model(mel_batch, img_batch)
|
||||||
|
pred = pred.cpu().numpy().transpose(0, 2, 3, 1) * 255.
|
||||||
|
|
||||||
|
counttime += (time.perf_counter() - t)
|
||||||
|
count += batch_size
|
||||||
|
#_totalframe += 1
|
||||||
|
if count>=100:
|
||||||
|
print(f"------actual avg infer fps:{count/counttime:.4f}")
|
||||||
|
count=0
|
||||||
|
counttime=0
|
||||||
|
for i,res_frame in enumerate(pred):
|
||||||
|
#self.__pushmedia(res_frame,loop,audio_track,video_track)
|
||||||
|
res_frame_queue.put((res_frame,__mirror_index(length,index),audio_frames[i*2:i*2+2]))
|
||||||
|
index = index + 1
|
||||||
|
#print('total batch time:',time.perf_counter()-starttime)
|
||||||
|
else:
|
||||||
|
time.sleep(1)
|
||||||
|
print('musereal inference processor stop')
|
||||||
|
|
||||||
|
@torch.no_grad()
|
||||||
|
class LipReal(BaseReal):
|
||||||
|
def __init__(self, opt):
|
||||||
|
super().__init__(opt)
|
||||||
|
#self.opt = opt # shared with the trainer's opt to support in-place modification of rendering parameters.
|
||||||
|
self.W = opt.W
|
||||||
|
self.H = opt.H
|
||||||
|
|
||||||
|
self.fps = opt.fps # 20 ms per frame
|
||||||
|
|
||||||
|
#### musetalk
|
||||||
|
self.avatar_id = opt.avatar_id
|
||||||
|
self.avatar_path = f"./data/avatars/{self.avatar_id}"
|
||||||
|
self.full_imgs_path = f"{self.avatar_path}/full_imgs"
|
||||||
|
self.face_imgs_path = f"{self.avatar_path}/face_imgs"
|
||||||
|
self.coords_path = f"{self.avatar_path}/coords.pkl"
|
||||||
|
self.batch_size = opt.batch_size
|
||||||
|
self.idx = 0
|
||||||
|
self.res_frame_queue = mp.Queue(self.batch_size*2)
|
||||||
|
#self.__loadmodels()
|
||||||
|
self.__loadavatar()
|
||||||
|
|
||||||
|
self.asr = LipASR(opt,self)
|
||||||
|
self.asr.warm_up()
|
||||||
|
#self.__warm_up()
|
||||||
|
|
||||||
|
self.render_event = mp.Event()
|
||||||
|
mp.Process(target=inference, args=(self.render_event,self.batch_size,self.face_imgs_path,
|
||||||
|
self.asr.feat_queue,self.asr.output_queue,self.res_frame_queue,
|
||||||
|
)).start()
|
||||||
|
|
||||||
|
# def __loadmodels(self):
|
||||||
|
# # load model weights
|
||||||
|
# self.audio_processor, self.vae, self.unet, self.pe = load_all_model()
|
||||||
|
# device = torch.device("cuda" if torch.cuda.is_available() else "cpu")
|
||||||
|
# self.timesteps = torch.tensor([0], device=device)
|
||||||
|
# self.pe = self.pe.half()
|
||||||
|
# self.vae.vae = self.vae.vae.half()
|
||||||
|
# self.unet.model = self.unet.model.half()
|
||||||
|
|
||||||
|
def __loadavatar(self):
|
||||||
|
with open(self.coords_path, 'rb') as f:
|
||||||
|
self.coord_list_cycle = pickle.load(f)
|
||||||
|
input_img_list = glob.glob(os.path.join(self.full_imgs_path, '*.[jpJP][pnPN]*[gG]'))
|
||||||
|
input_img_list = sorted(input_img_list, key=lambda x: int(os.path.splitext(os.path.basename(x))[0]))
|
||||||
|
self.frame_list_cycle = read_imgs(input_img_list)
|
||||||
|
#self.imagecache = ImgCache(len(self.coord_list_cycle),self.full_imgs_path,1000)
|
||||||
|
|
||||||
|
|
||||||
|
def process_frames(self,quit_event,loop=None,audio_track=None,video_track=None):
|
||||||
|
|
||||||
|
while not quit_event.is_set():
|
||||||
|
try:
|
||||||
|
res_frame,idx,audio_frames = self.res_frame_queue.get(block=True, timeout=1)
|
||||||
|
except queue.Empty:
|
||||||
|
continue
|
||||||
|
if audio_frames[0][1]!=0 and audio_frames[1][1]!=0: #全为静音数据,只需要取fullimg
|
||||||
|
self.speaking = False
|
||||||
|
audiotype = audio_frames[0][1]
|
||||||
|
if self.custom_index.get(audiotype) is not None: #有自定义视频
|
||||||
|
mirindex = self.mirror_index(len(self.custom_img_cycle[audiotype]),self.custom_index[audiotype])
|
||||||
|
combine_frame = self.custom_img_cycle[audiotype][mirindex]
|
||||||
|
self.custom_index[audiotype] += 1
|
||||||
|
# if not self.custom_opt[audiotype].loop and self.custom_index[audiotype]>=len(self.custom_img_cycle[audiotype]):
|
||||||
|
# self.curr_state = 1 #当前视频不循环播放,切换到静音状态
|
||||||
|
else:
|
||||||
|
combine_frame = self.frame_list_cycle[idx]
|
||||||
|
#combine_frame = self.imagecache.get_img(idx)
|
||||||
|
else:
|
||||||
|
self.speaking = True
|
||||||
|
bbox = self.coord_list_cycle[idx]
|
||||||
|
combine_frame = copy.deepcopy(self.frame_list_cycle[idx])
|
||||||
|
#combine_frame = copy.deepcopy(self.imagecache.get_img(idx))
|
||||||
|
y1, y2, x1, x2 = bbox
|
||||||
|
try:
|
||||||
|
res_frame = cv2.resize(res_frame.astype(np.uint8),(x2-x1,y2-y1))
|
||||||
|
except:
|
||||||
|
continue
|
||||||
|
#combine_frame = get_image(ori_frame,res_frame,bbox)
|
||||||
|
#t=time.perf_counter()
|
||||||
|
combine_frame[y1:y2, x1:x2] = res_frame
|
||||||
|
#print('blending time:',time.perf_counter()-t)
|
||||||
|
|
||||||
|
image = combine_frame #(outputs['image'] * 255).astype(np.uint8)
|
||||||
|
new_frame = VideoFrame.from_ndarray(image, format="bgr24")
|
||||||
|
asyncio.run_coroutine_threadsafe(video_track._queue.put(new_frame), loop)
|
||||||
|
if self.recording:
|
||||||
|
self.recordq_video.put(new_frame)
|
||||||
|
|
||||||
|
for audio_frame in audio_frames:
|
||||||
|
frame,type = audio_frame
|
||||||
|
frame = (frame * 32767).astype(np.int16)
|
||||||
|
new_frame = AudioFrame(format='s16', layout='mono', samples=frame.shape[0])
|
||||||
|
new_frame.planes[0].update(frame.tobytes())
|
||||||
|
new_frame.sample_rate=16000
|
||||||
|
# if audio_track._queue.qsize()>10:
|
||||||
|
# time.sleep(0.1)
|
||||||
|
asyncio.run_coroutine_threadsafe(audio_track._queue.put(new_frame), loop)
|
||||||
|
if self.recording:
|
||||||
|
self.recordq_audio.put(new_frame)
|
||||||
|
print('musereal process_frames thread stop')
|
||||||
|
|
||||||
|
def render(self,quit_event,loop=None,audio_track=None,video_track=None):
|
||||||
|
#if self.opt.asr:
|
||||||
|
# self.asr.warm_up()
|
||||||
|
|
||||||
|
self.tts.render(quit_event)
|
||||||
|
self.init_customindex()
|
||||||
|
process_thread = Thread(target=self.process_frames, args=(quit_event,loop,audio_track,video_track))
|
||||||
|
process_thread.start()
|
||||||
|
|
||||||
|
self.render_event.set() #start infer process render
|
||||||
|
count=0
|
||||||
|
totaltime=0
|
||||||
|
_starttime=time.perf_counter()
|
||||||
|
#_totalframe=0
|
||||||
|
while not quit_event.is_set():
|
||||||
|
# update texture every frame
|
||||||
|
# audio stream thread...
|
||||||
|
t = time.perf_counter()
|
||||||
|
self.asr.run_step()
|
||||||
|
|
||||||
|
# if video_track._queue.qsize()>=2*self.opt.batch_size:
|
||||||
|
# print('sleep qsize=',video_track._queue.qsize())
|
||||||
|
# time.sleep(0.04*video_track._queue.qsize()*0.8)
|
||||||
|
if video_track._queue.qsize()>=5:
|
||||||
|
print('sleep qsize=',video_track._queue.qsize())
|
||||||
|
time.sleep(0.04*video_track._queue.qsize()*0.8)
|
||||||
|
|
||||||
|
# delay = _starttime+_totalframe*0.04-time.perf_counter() #40ms
|
||||||
|
# if delay > 0:
|
||||||
|
# time.sleep(delay)
|
||||||
|
self.render_event.clear() #end infer process render
|
||||||
|
print('musereal thread stop')
|
||||||
|
|
|
@ -0,0 +1,56 @@
|
||||||
|
# 使用NVIDIA的CUDA基础镜像
|
||||||
|
#FROM nvidia/cuda:11.3.0-cudnn8-runtime-ubuntu18.04
|
||||||
|
#FROM m11007322/cuda11.3.0-cudnn8-devel-ubuntu20.04-jupyterlab
|
||||||
|
FROM nvidia/cuda:11.3.1-cudnn8-devel-ubuntu20.04
|
||||||
|
# 安装Python和pip
|
||||||
|
RUN apt-get update && apt-get install -y --no-install-recommends \
|
||||||
|
python3 \
|
||||||
|
python3-pip \
|
||||||
|
&& rm -rf /var/lib/apt/lists/*
|
||||||
|
|
||||||
|
# 安装Jupyter
|
||||||
|
RUN pip3 install --no-cache-dir jupyter
|
||||||
|
|
||||||
|
# 安装基础工具
|
||||||
|
RUN apt-get update -yq --fix-missing \
|
||||||
|
&& DEBIAN_FRONTEND=noninteractive apt-get install -yq --no-install-recommends \
|
||||||
|
pkg-config \
|
||||||
|
wget \
|
||||||
|
cmake \
|
||||||
|
curl \
|
||||||
|
git \
|
||||||
|
vim
|
||||||
|
|
||||||
|
# 创建一个新的Conda环境
|
||||||
|
RUN apt-get update && apt-get install -y wget \
|
||||||
|
&& wget https://repo.anaconda.com/miniconda/Miniconda3-latest-Linux-x86_64.sh \
|
||||||
|
&& /bin/bash Miniconda3-latest-Linux-x86_64.sh -b -p /opt/conda \
|
||||||
|
&& rm Miniconda3-latest-Linux-x86_64.sh \
|
||||||
|
&& apt-get remove --purge --auto-remove -y wget \
|
||||||
|
&& apt-get clean \
|
||||||
|
&& ln -s /opt/conda/bin/conda /usr/bin/conda \
|
||||||
|
&& conda update -n base -c defaults conda
|
||||||
|
|
||||||
|
SHELL ["/bin/bash","-ic"]
|
||||||
|
# 增加cuda全局变量
|
||||||
|
RUN echo "export CUDA_HOME=/usr/local/cuda" >> ~/.bashrc \
|
||||||
|
&& echo "export PATH=${CUDA_HOME}/bin:$PATH" >> ~/.bashrc \
|
||||||
|
&& echo "export LD_LIBRARY_PATH=${CUDA_HOME}/lib64:$LD_LIBRARY_PATH" >> ~/.bashrc \
|
||||||
|
&& source ~/.bashrc
|
||||||
|
|
||||||
|
#&& echo "nameserver 8.8.8.8" >> /etc/resolv.conf
|
||||||
|
|
||||||
|
# 安装cv2依赖,修复libGL.so.1错误
|
||||||
|
RUN apt-get update
|
||||||
|
RUN apt-get install ffmpeg libsm6 libxext6 -y
|
||||||
|
|
||||||
|
# 配置Jupyter
|
||||||
|
ENV JUPYTER_ENABLE_LAB=yes
|
||||||
|
ENV USER=root
|
||||||
|
ENV HOME=/home/$USER
|
||||||
|
|
||||||
|
# 设置工作目录
|
||||||
|
WORKDIR /root
|
||||||
|
|
||||||
|
# 设置启动命令
|
||||||
|
CMD ["jupyter", "lab", "--ip='*'", "--port=8888", "--no-browser", "--allow-root"]
|
|
@ -0,0 +1,22 @@
|
||||||
|
import openai
|
||||||
|
|
||||||
|
|
||||||
|
class GPT():
|
||||||
|
def __init__(self, model_path = 'gpt-3.5-turbo', api_key = None, base_url = None):
|
||||||
|
openai.api_key = api_key
|
||||||
|
self.model_path = model_path
|
||||||
|
if base_url != None:
|
||||||
|
openai.base_url = base_url
|
||||||
|
|
||||||
|
def chat(self, message):
|
||||||
|
response = openai.ChatCompletion.create(
|
||||||
|
model=self.model_path,
|
||||||
|
messages=[
|
||||||
|
{"role": "user", "content": message}
|
||||||
|
]
|
||||||
|
)
|
||||||
|
return response['choices'][0]['message']['content']
|
||||||
|
|
||||||
|
if __name__ == '__main__':
|
||||||
|
llm = GPT('gpt-3.5-turbo', '你的API Key','https://openai.api2d.net/v1')
|
||||||
|
response = llm.chat("如何应对压力?")
|
32
llm/LLM.py
32
llm/LLM.py
|
@ -1,38 +1,40 @@
|
||||||
from llm.Qwen import Qwen
|
from llm.Qwen import Qwen
|
||||||
from llm.Gemini import Gemini
|
from llm.Gemini import Gemini
|
||||||
from llm.ChatGPT import ChatGPT
|
from llm.ChatGPT import ChatGPT
|
||||||
|
from llm.VllmGPT import VllmGPT
|
||||||
|
|
||||||
|
|
||||||
def test_Qwen(question = "如何应对压力?", mode='offline', model_path="Qwen/Qwen-1_8B-Chat"):
|
def test_Qwen(question = "如何应对压力?", mode='offline', model_path="Qwen/Qwen-1_8B-Chat"):
|
||||||
llm = Qwen(mode, model_path)
|
llm = Qwen(mode, model_path)
|
||||||
answer = llm.generate(question)
|
answer = llm.generate(question)
|
||||||
print(answer)
|
print(answer)
|
||||||
|
|
||||||
def test_Gemini(question = "如何应对压力?", model_path='gemini-pro', api_key=None, proxy_url=None):
|
def test_Gemini(question = "如何应对压力?", model_path='gemini-pro', api_key=None, proxy_url=None):
|
||||||
llm = Gemini(model_path, api_key, proxy_url)
|
llm = Gemini(model_path, api_key, proxy_url)
|
||||||
answer = llm.generate(question)
|
answer = llm.generate(question)
|
||||||
print(answer)
|
print(answer)
|
||||||
|
|
||||||
class LLM:
|
class LLM:
|
||||||
def __init__(self, mode='offline'):
|
def __init__(self, mode='offline'):
|
||||||
self.mode = mode
|
self.mode = mode
|
||||||
|
|
||||||
def init_model(self, model_name, model_path, api_key=None, proxy_url=None):
|
def init_model(self, model_name, model_path, api_key=None, proxy_url=None):
|
||||||
if model_name not in ['Qwen', 'Gemini', 'ChatGPT']:
|
if model_name not in ['Qwen', 'Gemini', 'ChatGPT', 'VllmGPT']:
|
||||||
raise ValueError("model_name must be 'ChatGPT', 'Qwen', or 'Gemini'(其他模型还未集成)")
|
raise ValueError("model_name must be 'ChatGPT', 'VllmGPT', 'Qwen', or 'Gemini'(其他模型还未集成)")
|
||||||
|
|
||||||
if model_name == 'Gemini':
|
if model_name == 'Gemini':
|
||||||
llm = Gemini(model_path, api_key, proxy_url)
|
llm = Gemini(model_path, api_key, proxy_url)
|
||||||
elif model_name == 'ChatGPT':
|
elif model_name == 'ChatGPT':
|
||||||
llm = ChatGPT(model_path, api_key=api_key)
|
llm = ChatGPT(model_path, api_key=api_key)
|
||||||
elif model_name == 'Qwen':
|
elif model_name == 'Qwen':
|
||||||
llm = Qwen(self.mode, model_path)
|
llm = Qwen(model_path=model_path, api_key=api_key, api_base=proxy_url)
|
||||||
|
elif model_name == 'VllmGPT':
|
||||||
|
llm = VllmGPT()
|
||||||
return llm
|
return llm
|
||||||
|
|
||||||
|
|
||||||
def test_Qwen(self, question="如何应对压力?", model_path="Qwen/Qwen-1_8B-Chat"):
|
def test_Qwen(self, question="如何应对压力?", model_path="Qwen/Qwen-1_8B-Chat", api_key=None, proxy_url=None):
|
||||||
llm = Qwen(self.mode, model_path)
|
llm = Qwen(model_path=model_path, api_key=api_key, api_base=proxy_url)
|
||||||
answer = llm.generate(question)
|
answer = llm.chat(question)
|
||||||
print(answer)
|
print(answer)
|
||||||
|
|
||||||
def test_Gemini(self, question="如何应对压力?", model_path='gemini-pro', api_key=None, proxy_url=None):
|
def test_Gemini(self, question="如何应对压力?", model_path='gemini-pro', api_key=None, proxy_url=None):
|
||||||
|
@ -42,7 +44,11 @@ class LLM:
|
||||||
|
|
||||||
if __name__ == '__main__':
|
if __name__ == '__main__':
|
||||||
llm = LLM()
|
llm = LLM()
|
||||||
llm.test_Gemini(api_key='你的API Key', proxy_url=None)
|
# llm.test_Gemini(api_key='你的API Key', proxy_url=None)
|
||||||
# llm = LLM().init_model('Gemini', model_path= 'gemini-pro',api_key='AIzaSyBWAWfT8zsyAZcRIXLS5Vzlw8KKCN9qsAg', proxy_url='http://172.31.71.58:7890')
|
# llm = LLM().init_model('Gemini', model_path= 'gemini-pro',api_key='AIzaSyBWAWfT8zsyAZcRIXLS5Vzlw8KKCN9qsAg', proxy_url='http://172.31.71.58:7890')
|
||||||
# response = llm.chat("如何应对压力?")
|
# response = llm.chat("如何应对压力?")
|
||||||
# print(response)
|
# llm = LLM().init_model('VllmGPT', model_path= 'THUDM/chatglm3-6b')
|
||||||
|
# response = llm.chat("如何应对压力?")
|
||||||
|
# print(response)
|
||||||
|
|
||||||
|
llm.test_Qwen(api_key="none", proxy_url="http://10.1.1.113:18000/v1")
|
||||||
|
|
60
llm/Qwen.py
60
llm/Qwen.py
|
@ -1,25 +1,56 @@
|
||||||
import os
|
import os
|
||||||
import torch
|
import openai
|
||||||
import requests
|
|
||||||
from transformers import AutoModelForCausalLM, AutoTokenizer
|
'''
|
||||||
|
`huggingface`连接不上可以使用 `modelscope`
|
||||||
|
`pip install modelscope`
|
||||||
|
'''
|
||||||
|
from modelscope import AutoModelForCausalLM, AutoTokenizer
|
||||||
|
#from transformers import AutoModelForCausalLM, AutoTokenizer
|
||||||
|
|
||||||
os.environ['CUDA_LAUNCH_BLOCKING'] = '1'
|
os.environ['CUDA_LAUNCH_BLOCKING'] = '1'
|
||||||
|
|
||||||
|
|
||||||
class Qwen:
|
class Qwen:
|
||||||
def __init__(self, model_path="Qwen/Qwen-1_8B-Chat") -> None:
|
def __init__(self, model_path="Qwen/Qwen-1_8B-Chat", api_base=None, api_key=None) -> None:
|
||||||
'''暂时不写api版本,与Linly-api相类似,感兴趣可以实现一下'''
|
'''暂时不写api版本,与Linly-api相类似,感兴趣可以实现一下'''
|
||||||
|
# 默认本地推理
|
||||||
|
self.local = True
|
||||||
|
|
||||||
|
# api_base和api_key不为空时使用openapi的方式
|
||||||
|
if api_key is not None and api_base is not None:
|
||||||
|
openai.api_base = api_base
|
||||||
|
openai.api_key = api_key
|
||||||
|
self.local = False
|
||||||
|
return
|
||||||
|
|
||||||
self.model, self.tokenizer = self.init_model(model_path)
|
self.model, self.tokenizer = self.init_model(model_path)
|
||||||
|
self.data = {}
|
||||||
def init_model(self, path = "Qwen/Qwen-1_8B-Chat"):
|
|
||||||
model = AutoModelForCausalLM.from_pretrained("Qwen/Qwen-1_8B-Chat",
|
def init_model(self, path="Qwen/Qwen-1_8B-Chat"):
|
||||||
device_map="auto",
|
model = AutoModelForCausalLM.from_pretrained("Qwen/Qwen-1_8B-Chat",
|
||||||
|
device_map="auto",
|
||||||
trust_remote_code=True).eval()
|
trust_remote_code=True).eval()
|
||||||
tokenizer = AutoTokenizer.from_pretrained(path, trust_remote_code=True)
|
tokenizer = AutoTokenizer.from_pretrained(path, trust_remote_code=True)
|
||||||
|
|
||||||
return model, tokenizer
|
return model, tokenizer
|
||||||
|
|
||||||
def chat(self, question):
|
|
||||||
|
|
||||||
self.data["question"] = f"{self.prompt} ### Instruction:{question} ### Response:"
|
def chat(self, question):
|
||||||
|
# 优先调用qwen openapi的方式
|
||||||
|
if not self.local:
|
||||||
|
# 不使用流式回复的请求
|
||||||
|
response = openai.ChatCompletion.create(
|
||||||
|
model="Qwen",
|
||||||
|
messages=[
|
||||||
|
{"role": "user", "content": question}
|
||||||
|
],
|
||||||
|
stream=False,
|
||||||
|
stop=[]
|
||||||
|
)
|
||||||
|
return response.choices[0].message.content
|
||||||
|
|
||||||
|
# 默认本地推理
|
||||||
|
self.data["question"] = f"{question} ### Instruction:{question} ### Response:"
|
||||||
try:
|
try:
|
||||||
response, history = self.model.chat(self.tokenizer, self.data["question"], history=None)
|
response, history = self.model.chat(self.tokenizer, self.data["question"], history=None)
|
||||||
print(history)
|
print(history)
|
||||||
|
@ -27,11 +58,12 @@ class Qwen:
|
||||||
except:
|
except:
|
||||||
return "对不起,你的请求出错了,请再次尝试。\nSorry, your request has encountered an error. Please try again.\n"
|
return "对不起,你的请求出错了,请再次尝试。\nSorry, your request has encountered an error. Please try again.\n"
|
||||||
|
|
||||||
|
|
||||||
def test():
|
def test():
|
||||||
llm = Qwen(model_path="Qwen/Qwen-1_8B-Chat")
|
llm = Qwen(model_path="Qwen/Qwen-1_8B-Chat")
|
||||||
answer = llm.generate("如何应对压力?")
|
answer = llm.chat(question="如何应对压力?")
|
||||||
print(answer)
|
print(answer)
|
||||||
|
|
||||||
|
|
||||||
if __name__ == '__main__':
|
if __name__ == '__main__':
|
||||||
test()
|
test()
|
||||||
|
|
|
@ -0,0 +1,54 @@
|
||||||
|
1、利用vllm可以显著推理加速大模型
|
||||||
|
|
||||||
|
conda create -n vllm python=3.10
|
||||||
|
conda activate vllm
|
||||||
|
conda install pytorch==2.1.0 torchvision==0.16.0 torchaudio==2.1.0 pytorch-cuda=12.1 -c pytorch -c nvidia
|
||||||
|
|
||||||
|
2、启动推理
|
||||||
|
python -m vllm.entrypoints.openai.api_server --tensor-parallel-size=1 --trust-remote-code --max-model-len 1024 --model THUDM/chatglm3-6b
|
||||||
|
指定ip和端口:--host 127.0.0.1 --port 8101
|
||||||
|
|
||||||
|
python -m vllm.entrypoints.openai.api_server --port 8101 --tensor-parallel-size=1 --trust-remote-code --max-model-len 1024 --model THUDM/chatglm3-6b
|
||||||
|
|
||||||
|
CUDA_VISIBLE_DEVICES=6,7 python -m vllm.entrypoints.openai.api_server \
|
||||||
|
--model="/data/mnt/ShareFolder/common_models/Ziya-Reader-13B-v1.0" \
|
||||||
|
--max-model-len=8192 \
|
||||||
|
--tensor-parallel-size=2 \
|
||||||
|
--trust-remote-code \
|
||||||
|
--port=8101
|
||||||
|
|
||||||
|
|
||||||
|
3、测试
|
||||||
|
curl http://127.0.0.1:8101/v1/completions \
|
||||||
|
-H "Content-Type: application/json" \
|
||||||
|
-d '{
|
||||||
|
"model": "THUDM/chatglm3-6b",
|
||||||
|
"prompt": "请用20字内回复我,你今年多大了",
|
||||||
|
"max_tokens": 20,
|
||||||
|
"temperature": 0
|
||||||
|
}'
|
||||||
|
|
||||||
|
多轮对话
|
||||||
|
curl -X POST "http://127.0.0.1:8101/v1/completions" \
|
||||||
|
-H "Content-Type: application/json" \
|
||||||
|
-d "{\"model\": \"THUDM/chatglm3-6b\",\"prompt\": \"你叫什么名字\", \"history\": [{\"role\": \"user\", \"content\": \"你出生在哪里.\"}, {\"role\": \"assistant\", \"content\": \"出生在北京\"}]}"
|
||||||
|
|
||||||
|
多轮对话
|
||||||
|
curl -X POST "http://127.0.0.1:8101/v1/chat/completions" \
|
||||||
|
-H "Content-Type: application/json" \
|
||||||
|
-d "{\"model\": \"THUDM/chatglm3-6b\", \"messages\": [{\"role\": \"system\", \"content\": \"You are ChatGLM3, a large language model trained by Zhipu.AI. Follow the user's instructions carefully. Respond using markdown.\"}, {\"role\": \"user\", \"content\": \"你好,给我讲一个故事,大概100字\"}], \"stream\": false, \"max_tokens\": 100, \"temperature\": 0.8, \"top_p\": 0.8}"
|
||||||
|
|
||||||
|
|
||||||
|
4、启动前端访问
|
||||||
|
docker run -d \
|
||||||
|
--network=host \
|
||||||
|
--name nginx2 --restart=always \
|
||||||
|
-v $PWD/nginx/conf/nginx.conf:/etc/nginx/nginx.conf \
|
||||||
|
-v $PWD/nginx/html:/usr/share/nginx/html \
|
||||||
|
-v $PWD/nginx/logs:/var/log/nginx \
|
||||||
|
--privileged=true \
|
||||||
|
--restart=always \
|
||||||
|
nginx
|
||||||
|
|
||||||
|
|
||||||
|
参考文档:https://docs.vllm.ai/en/latest/
|
|
@ -0,0 +1,74 @@
|
||||||
|
一、cuda11.3容器启动过程
|
||||||
|
1、拷贝Dockerfile文件到任意磁盘目录,然后执行下面的命令
|
||||||
|
docker build -t nvidia/cuda:11.3.1-cudnn8-devel-ubuntu20.04-jupyter-conda .
|
||||||
|
|
||||||
|
docker images
|
||||||
|
|
||||||
|
2、启动容器
|
||||||
|
打开镜像(常规模式--支持使用GPU)
|
||||||
|
docker run -i -t --gpus all nvidia/cuda:11.3.1-cudnn8-devel-ubuntu20.04-jupyter-conda /bin/bash
|
||||||
|
|
||||||
|
|
||||||
|
打开镜像(增强模式--支持使用GPU、映射目录、设置内存)
|
||||||
|
docker run -i -t -v /home/liguopu/:/guopu:rw --gpus all --shm-size 16G nvidia/cuda:11.3.1-cudnn8-devel-ubuntu20.04 /bin/bash
|
||||||
|
|
||||||
|
测试环境(使用端口映射,把服务映射出去)
|
||||||
|
docker run -i -td --name metehuman --gpus -p 8000:8000 all nvidia/cuda:11.3.1-cudnn8-devel-ubuntu20.04-jupyter-conda /bin/bash
|
||||||
|
|
||||||
|
正式使用(8000端口为业务对外的服务端口,根据情况可以自行增加)
|
||||||
|
docker run -it --rm -p 8886:8888 -p 8000:8000 --gpus all nvidia/cuda:11.3.1-cudnn8-devel-ubuntu20.04-jupyter-conda
|
||||||
|
docker run -itd -p 8886:8888 -p 8000:8000 --gpus all nvidia/cuda:11.3.1-cudnn8-devel-ubuntu20.04-jupyter-conda
|
||||||
|
docker run -itd --name metehuman -p 8886:8888 -p 8000:8000 --gpus all nvidia/cuda:11.3.1-cudnn8-devel-ubuntu20.04-jupyter-conda
|
||||||
|
|
||||||
|
docker run --gpus '"device=vgpu,id=0"' -it --rm nvidia/cuda:11.0-base nvidia-smi
|
||||||
|
|
||||||
|
docker run -itd --name metehuman \
|
||||||
|
-p 8885:8888 -p 8001:8000 \
|
||||||
|
-e GRANT_SUDO=yes \
|
||||||
|
-e JUPYTER_ENABLE_LAB=yes \
|
||||||
|
--user root \
|
||||||
|
--gpus all \
|
||||||
|
nvidia/cuda:11.3.1-cudnn8-devel-ubuntu20.04-jupyter-conda
|
||||||
|
|
||||||
|
3、查看token
|
||||||
|
token=$(docker exec -it metehuman jupyter server list | grep -oP '(?<=token=)[a-zA-Z0-9]+')
|
||||||
|
echo $token
|
||||||
|
|
||||||
|
二、启动默认测试镜像
|
||||||
|
docker pull m11007322/cuda11.3.0-cudnn8-devel-ubuntu20.04-jupyterlab
|
||||||
|
docker run -it \
|
||||||
|
-d \
|
||||||
|
--gpus all \
|
||||||
|
-p 8887:8888 \
|
||||||
|
-p 8001:8000 \
|
||||||
|
--name metehuman2 \
|
||||||
|
--user root \
|
||||||
|
-e NB_USER="ubuntu" \
|
||||||
|
-e CHOWN_HOME=yes \
|
||||||
|
-e GRANT_SUDO=yes \
|
||||||
|
-w "/home/${NB_USER}" \
|
||||||
|
-v "$PWD":"/home/$USER/work" \
|
||||||
|
m11007322/cuda11.3.0-cudnn8-devel-ubuntu20.04-jupyterlab
|
||||||
|
|
||||||
|
三、启动jupter镜像测试
|
||||||
|
docker run -itd --name test \
|
||||||
|
-p 8886:8888 -p 8000:8000 \
|
||||||
|
-e GRANT_SUDO=yes \
|
||||||
|
-e JUPYTER_ENABLE_LAB=yes \
|
||||||
|
--user root \
|
||||||
|
--gpus '"device=vgpu,id=0"' \
|
||||||
|
nvidia/cuda:11.3.1-cudnn8-devel-ubuntu20.04-jupyter-conda
|
||||||
|
|
||||||
|
docker run -it --name test --network=host --dns 8.8.8.8 --dns 8.8.4.4 --rm ubuntu
|
||||||
|
docker run -it --gpus all --network=host --rm registry.cn-hangzhou.aliyuncs.com/lipku/nerfstream:v1.3
|
||||||
|
|
||||||
|
四、查看容器IP
|
||||||
|
docker inspect bceda087524e | grep IPAddress
|
||||||
|
|
||||||
|
curl https://openai.api2d.net/v1/chat/completions \
|
||||||
|
-H 'Content-Type: application/json' \
|
||||||
|
-H 'Authorization: Bearer fk193752-RlcPi2mBQqPOU5u1F8SFkG2z0gtxD0HS' \
|
||||||
|
-d '{
|
||||||
|
"model": "gpt-3.5-turbo",
|
||||||
|
"messages": [{"role": "user", "content": "你好!给我讲个笑话。"}]
|
||||||
|
}'
|
|
@ -0,0 +1,81 @@
|
||||||
|
import json
|
||||||
|
import requests
|
||||||
|
# from core import content_db
|
||||||
|
|
||||||
|
class VllmGPT:
|
||||||
|
|
||||||
|
def __init__(self, host="192.168.1.3",
|
||||||
|
port="8101",
|
||||||
|
model="THUDM/chatglm3-6b",
|
||||||
|
max_tokens="1024"):
|
||||||
|
self.host = host
|
||||||
|
self.port = port
|
||||||
|
self.model=model
|
||||||
|
self.max_tokens=max_tokens
|
||||||
|
self.__URL = "http://{}:{}/v1/completions".format(self.host, self.port)
|
||||||
|
self.__URL2 = "http://{}:{}/v1/chat/completions".format(self.host, self.port)
|
||||||
|
|
||||||
|
def chat(self,cont):
|
||||||
|
chat_list = []
|
||||||
|
# contentdb = content_db.new_instance()
|
||||||
|
# list = contentdb.get_list('all','desc',11)
|
||||||
|
# answer_info = dict()
|
||||||
|
# chat_list = []
|
||||||
|
# i = len(list)-1
|
||||||
|
# while i >= 0:
|
||||||
|
# answer_info = dict()
|
||||||
|
# if list[i][0] == "member":
|
||||||
|
# answer_info["role"] = "user"
|
||||||
|
# answer_info["content"] = list[i][2]
|
||||||
|
# elif list[i][0] == "fay":
|
||||||
|
# answer_info["role"] = "bot"
|
||||||
|
# answer_info["content"] = list[i][2]
|
||||||
|
# chat_list.append(answer_info)
|
||||||
|
# i -= 1
|
||||||
|
content = {
|
||||||
|
"model": self.model,
|
||||||
|
"prompt":"请简单回复我。" + cont,
|
||||||
|
"history":chat_list}
|
||||||
|
url = self.__URL
|
||||||
|
req = json.dumps(content)
|
||||||
|
|
||||||
|
headers = {'content-type': 'application/json'}
|
||||||
|
r = requests.post(url, headers=headers, data=req)
|
||||||
|
res = json.loads(r.text)
|
||||||
|
|
||||||
|
return res['choices'][0]['text']
|
||||||
|
|
||||||
|
def question2(self,cont):
|
||||||
|
chat_list = []
|
||||||
|
# contentdb = content_db.new_instance()
|
||||||
|
# list = contentdb.get_list('all','desc',11)
|
||||||
|
# answer_info = dict()
|
||||||
|
# chat_list = []
|
||||||
|
# i = len(list)-1
|
||||||
|
# while i >= 0:
|
||||||
|
# answer_info = dict()
|
||||||
|
# if list[i][0] == "member":
|
||||||
|
# answer_info["role"] = "user"
|
||||||
|
# answer_info["content"] = list[i][2]
|
||||||
|
# elif list[i][0] == "fay":
|
||||||
|
# answer_info["role"] = "bot"
|
||||||
|
# answer_info["content"] = list[i][2]
|
||||||
|
# chat_list.append(answer_info)
|
||||||
|
# i -= 1
|
||||||
|
content = {
|
||||||
|
"model": self.model,
|
||||||
|
"prompt":"请简单回复我。" + cont,
|
||||||
|
"history":chat_list}
|
||||||
|
url = self.__URL2
|
||||||
|
req = json.dumps(content)
|
||||||
|
|
||||||
|
headers = {'content-type': 'application/json'}
|
||||||
|
r = requests.post(url, headers=headers, data=req)
|
||||||
|
res = json.loads(r.text)
|
||||||
|
|
||||||
|
return res['choices'][0]['message']['content']
|
||||||
|
|
||||||
|
if __name__ == "__main__":
|
||||||
|
vllm = VllmGPT('192.168.1.3','8101')
|
||||||
|
req = vllm.chat("你叫什么名字啊今年多大了")
|
||||||
|
print(req)
|
|
@ -0,0 +1,36 @@
|
||||||
|
import time
|
||||||
|
import numpy as np
|
||||||
|
|
||||||
|
import queue
|
||||||
|
from queue import Queue
|
||||||
|
import multiprocessing as mp
|
||||||
|
from baseasr import BaseASR
|
||||||
|
from musetalk.whisper.audio2feature import Audio2Feature
|
||||||
|
|
||||||
|
class MuseASR(BaseASR):
|
||||||
|
def __init__(self, opt, parent,audio_processor:Audio2Feature):
|
||||||
|
super().__init__(opt,parent)
|
||||||
|
self.audio_processor = audio_processor
|
||||||
|
|
||||||
|
def run_step(self):
|
||||||
|
############################################## extract audio feature ##############################################
|
||||||
|
start_time = time.time()
|
||||||
|
for _ in range(self.batch_size*2):
|
||||||
|
audio_frame,type=self.get_audio_frame()
|
||||||
|
self.frames.append(audio_frame)
|
||||||
|
self.output_queue.put((audio_frame,type))
|
||||||
|
|
||||||
|
if len(self.frames) <= self.stride_left_size + self.stride_right_size:
|
||||||
|
return
|
||||||
|
|
||||||
|
inputs = np.concatenate(self.frames) # [N * chunk]
|
||||||
|
whisper_feature = self.audio_processor.audio2feat(inputs)
|
||||||
|
# for feature in whisper_feature:
|
||||||
|
# self.audio_feats.append(feature)
|
||||||
|
#print(f"processing audio costs {(time.time() - start_time) * 1000}ms, inputs shape:{inputs.shape} whisper_feature len:{len(whisper_feature)}")
|
||||||
|
whisper_chunks = self.audio_processor.feature2chunks(feature_array=whisper_feature,fps=self.fps/2,batch_size=self.batch_size,start=self.stride_left_size/2 )
|
||||||
|
#print(f"whisper_chunks len:{len(whisper_chunks)},self.audio_feats len:{len(self.audio_feats)},self.output_queue len:{self.output_queue.qsize()}")
|
||||||
|
#self.audio_feats = self.audio_feats[-(self.stride_left_size + self.stride_right_size):]
|
||||||
|
self.feat_queue.put(whisper_chunks)
|
||||||
|
# discard the old part to save memory
|
||||||
|
self.frames = self.frames[-(self.stride_left_size + self.stride_right_size):]
|
|
@ -0,0 +1,318 @@
|
||||||
|
import math
|
||||||
|
import torch
|
||||||
|
import numpy as np
|
||||||
|
|
||||||
|
#from .utils import *
|
||||||
|
import subprocess
|
||||||
|
import os
|
||||||
|
import time
|
||||||
|
import torch.nn.functional as F
|
||||||
|
import cv2
|
||||||
|
import glob
|
||||||
|
import pickle
|
||||||
|
import copy
|
||||||
|
|
||||||
|
import queue
|
||||||
|
from queue import Queue
|
||||||
|
from threading import Thread, Event
|
||||||
|
from io import BytesIO
|
||||||
|
import multiprocessing as mp
|
||||||
|
|
||||||
|
from musetalk.utils.utils import get_file_type,get_video_fps,datagen
|
||||||
|
#from musetalk.utils.preprocessing import get_landmark_and_bbox,read_imgs,coord_placeholder
|
||||||
|
from musetalk.utils.blending import get_image,get_image_prepare_material,get_image_blending
|
||||||
|
from musetalk.utils.utils import load_all_model,load_diffusion_model,load_audio_model
|
||||||
|
from ttsreal import EdgeTTS,VoitsTTS,XTTS
|
||||||
|
|
||||||
|
from museasr import MuseASR
|
||||||
|
import asyncio
|
||||||
|
from av import AudioFrame, VideoFrame
|
||||||
|
from basereal import BaseReal
|
||||||
|
|
||||||
|
from tqdm import tqdm
|
||||||
|
def read_imgs(img_list):
|
||||||
|
frames = []
|
||||||
|
print('reading images...')
|
||||||
|
for img_path in tqdm(img_list):
|
||||||
|
frame = cv2.imread(img_path)
|
||||||
|
frames.append(frame)
|
||||||
|
return frames
|
||||||
|
|
||||||
|
def __mirror_index(size, index):
|
||||||
|
#size = len(self.coord_list_cycle)
|
||||||
|
turn = index // size
|
||||||
|
res = index % size
|
||||||
|
if turn % 2 == 0:
|
||||||
|
return res
|
||||||
|
else:
|
||||||
|
return size - res - 1
|
||||||
|
@torch.no_grad()
|
||||||
|
def inference(render_event,batch_size,latents_out_path,audio_feat_queue,audio_out_queue,res_frame_queue,
|
||||||
|
): #vae, unet, pe,timesteps
|
||||||
|
|
||||||
|
vae, unet, pe = load_diffusion_model()
|
||||||
|
device = torch.device("cuda" if torch.cuda.is_available() else "cpu")
|
||||||
|
timesteps = torch.tensor([0], device=device)
|
||||||
|
pe = pe.half()
|
||||||
|
vae.vae = vae.vae.half()
|
||||||
|
unet.model = unet.model.half()
|
||||||
|
|
||||||
|
input_latent_list_cycle = torch.load(latents_out_path)
|
||||||
|
length = len(input_latent_list_cycle)
|
||||||
|
index = 0
|
||||||
|
count=0
|
||||||
|
counttime=0
|
||||||
|
print('start inference')
|
||||||
|
while True:
|
||||||
|
if render_event.is_set():
|
||||||
|
starttime=time.perf_counter()
|
||||||
|
try:
|
||||||
|
whisper_chunks = audio_feat_queue.get(block=True, timeout=1)
|
||||||
|
except queue.Empty:
|
||||||
|
continue
|
||||||
|
is_all_silence=True
|
||||||
|
audio_frames = []
|
||||||
|
for _ in range(batch_size*2):
|
||||||
|
frame,type = audio_out_queue.get()
|
||||||
|
audio_frames.append((frame,type))
|
||||||
|
if type==0:
|
||||||
|
is_all_silence=False
|
||||||
|
if is_all_silence:
|
||||||
|
for i in range(batch_size):
|
||||||
|
res_frame_queue.put((None,__mirror_index(length,index),audio_frames[i*2:i*2+2]))
|
||||||
|
index = index + 1
|
||||||
|
else:
|
||||||
|
# print('infer=======')
|
||||||
|
t=time.perf_counter()
|
||||||
|
whisper_batch = np.stack(whisper_chunks)
|
||||||
|
latent_batch = []
|
||||||
|
for i in range(batch_size):
|
||||||
|
idx = __mirror_index(length,index+i)
|
||||||
|
latent = input_latent_list_cycle[idx]
|
||||||
|
latent_batch.append(latent)
|
||||||
|
latent_batch = torch.cat(latent_batch, dim=0)
|
||||||
|
|
||||||
|
# for i, (whisper_batch,latent_batch) in enumerate(gen):
|
||||||
|
audio_feature_batch = torch.from_numpy(whisper_batch)
|
||||||
|
audio_feature_batch = audio_feature_batch.to(device=unet.device,
|
||||||
|
dtype=unet.model.dtype)
|
||||||
|
audio_feature_batch = pe(audio_feature_batch)
|
||||||
|
latent_batch = latent_batch.to(dtype=unet.model.dtype)
|
||||||
|
# print('prepare time:',time.perf_counter()-t)
|
||||||
|
# t=time.perf_counter()
|
||||||
|
|
||||||
|
pred_latents = unet.model(latent_batch,
|
||||||
|
timesteps,
|
||||||
|
encoder_hidden_states=audio_feature_batch).sample
|
||||||
|
# print('unet time:',time.perf_counter()-t)
|
||||||
|
# t=time.perf_counter()
|
||||||
|
recon = vae.decode_latents(pred_latents)
|
||||||
|
# print('vae time:',time.perf_counter()-t)
|
||||||
|
#print('diffusion len=',len(recon))
|
||||||
|
counttime += (time.perf_counter() - t)
|
||||||
|
count += batch_size
|
||||||
|
#_totalframe += 1
|
||||||
|
if count>=100:
|
||||||
|
print(f"------actual avg infer fps:{count/counttime:.4f}")
|
||||||
|
count=0
|
||||||
|
counttime=0
|
||||||
|
for i,res_frame in enumerate(recon):
|
||||||
|
#self.__pushmedia(res_frame,loop,audio_track,video_track)
|
||||||
|
res_frame_queue.put((res_frame,__mirror_index(length,index),audio_frames[i*2:i*2+2]))
|
||||||
|
index = index + 1
|
||||||
|
#print('total batch time:',time.perf_counter()-starttime)
|
||||||
|
else:
|
||||||
|
time.sleep(1)
|
||||||
|
print('musereal inference processor stop')
|
||||||
|
|
||||||
|
@torch.no_grad()
|
||||||
|
class MuseReal(BaseReal):
|
||||||
|
def __init__(self, opt):
|
||||||
|
super().__init__(opt)
|
||||||
|
#self.opt = opt # shared with the trainer's opt to support in-place modification of rendering parameters.
|
||||||
|
self.W = opt.W
|
||||||
|
self.H = opt.H
|
||||||
|
|
||||||
|
self.fps = opt.fps # 20 ms per frame
|
||||||
|
|
||||||
|
#### musetalk
|
||||||
|
self.avatar_id = opt.avatar_id
|
||||||
|
self.video_path = '' #video_path
|
||||||
|
self.bbox_shift = opt.bbox_shift
|
||||||
|
self.avatar_path = f"./data/avatars/{self.avatar_id}"
|
||||||
|
self.full_imgs_path = f"{self.avatar_path}/full_imgs"
|
||||||
|
self.coords_path = f"{self.avatar_path}/coords.pkl"
|
||||||
|
self.latents_out_path= f"{self.avatar_path}/latents.pt"
|
||||||
|
self.video_out_path = f"{self.avatar_path}/vid_output/"
|
||||||
|
self.mask_out_path =f"{self.avatar_path}/mask"
|
||||||
|
self.mask_coords_path =f"{self.avatar_path}/mask_coords.pkl"
|
||||||
|
self.avatar_info_path = f"{self.avatar_path}/avator_info.json"
|
||||||
|
self.avatar_info = {
|
||||||
|
"avatar_id":self.avatar_id,
|
||||||
|
"video_path":self.video_path,
|
||||||
|
"bbox_shift":self.bbox_shift
|
||||||
|
}
|
||||||
|
self.batch_size = opt.batch_size
|
||||||
|
self.idx = 0
|
||||||
|
self.res_frame_queue = mp.Queue(self.batch_size*2)
|
||||||
|
self.__loadmodels()
|
||||||
|
self.__loadavatar()
|
||||||
|
|
||||||
|
self.asr = MuseASR(opt,self,self.audio_processor)
|
||||||
|
self.asr.warm_up()
|
||||||
|
#self.__warm_up()
|
||||||
|
|
||||||
|
self.render_event = mp.Event()
|
||||||
|
mp.Process(target=inference, args=(self.render_event,self.batch_size,self.latents_out_path,
|
||||||
|
self.asr.feat_queue,self.asr.output_queue,self.res_frame_queue,
|
||||||
|
)).start() #self.vae, self.unet, self.pe,self.timesteps
|
||||||
|
|
||||||
|
def __loadmodels(self):
|
||||||
|
# load model weights
|
||||||
|
self.audio_processor= load_audio_model()
|
||||||
|
# self.audio_processor, self.vae, self.unet, self.pe = load_all_model()
|
||||||
|
# device = torch.device("cuda" if torch.cuda.is_available() else "cpu")
|
||||||
|
# self.timesteps = torch.tensor([0], device=device)
|
||||||
|
# self.pe = self.pe.half()
|
||||||
|
# self.vae.vae = self.vae.vae.half()
|
||||||
|
# self.unet.model = self.unet.model.half()
|
||||||
|
|
||||||
|
def __loadavatar(self):
|
||||||
|
#self.input_latent_list_cycle = torch.load(self.latents_out_path)
|
||||||
|
with open(self.coords_path, 'rb') as f:
|
||||||
|
self.coord_list_cycle = pickle.load(f)
|
||||||
|
input_img_list = glob.glob(os.path.join(self.full_imgs_path, '*.[jpJP][pnPN]*[gG]'))
|
||||||
|
input_img_list = sorted(input_img_list, key=lambda x: int(os.path.splitext(os.path.basename(x))[0]))
|
||||||
|
self.frame_list_cycle = read_imgs(input_img_list)
|
||||||
|
with open(self.mask_coords_path, 'rb') as f:
|
||||||
|
self.mask_coords_list_cycle = pickle.load(f)
|
||||||
|
input_mask_list = glob.glob(os.path.join(self.mask_out_path, '*.[jpJP][pnPN]*[gG]'))
|
||||||
|
input_mask_list = sorted(input_mask_list, key=lambda x: int(os.path.splitext(os.path.basename(x))[0]))
|
||||||
|
self.mask_list_cycle = read_imgs(input_mask_list)
|
||||||
|
|
||||||
|
|
||||||
|
def __mirror_index(self, index):
|
||||||
|
size = len(self.coord_list_cycle)
|
||||||
|
turn = index // size
|
||||||
|
res = index % size
|
||||||
|
if turn % 2 == 0:
|
||||||
|
return res
|
||||||
|
else:
|
||||||
|
return size - res - 1
|
||||||
|
|
||||||
|
def __warm_up(self):
|
||||||
|
self.asr.run_step()
|
||||||
|
whisper_chunks = self.asr.get_next_feat()
|
||||||
|
whisper_batch = np.stack(whisper_chunks)
|
||||||
|
latent_batch = []
|
||||||
|
for i in range(self.batch_size):
|
||||||
|
idx = self.__mirror_index(self.idx+i)
|
||||||
|
latent = self.input_latent_list_cycle[idx]
|
||||||
|
latent_batch.append(latent)
|
||||||
|
latent_batch = torch.cat(latent_batch, dim=0)
|
||||||
|
print('infer=======')
|
||||||
|
# for i, (whisper_batch,latent_batch) in enumerate(gen):
|
||||||
|
audio_feature_batch = torch.from_numpy(whisper_batch)
|
||||||
|
audio_feature_batch = audio_feature_batch.to(device=self.unet.device,
|
||||||
|
dtype=self.unet.model.dtype)
|
||||||
|
audio_feature_batch = self.pe(audio_feature_batch)
|
||||||
|
latent_batch = latent_batch.to(dtype=self.unet.model.dtype)
|
||||||
|
|
||||||
|
pred_latents = self.unet.model(latent_batch,
|
||||||
|
self.timesteps,
|
||||||
|
encoder_hidden_states=audio_feature_batch).sample
|
||||||
|
recon = self.vae.decode_latents(pred_latents)
|
||||||
|
|
||||||
|
|
||||||
|
def process_frames(self,quit_event,loop=None,audio_track=None,video_track=None):
|
||||||
|
|
||||||
|
while not quit_event.is_set():
|
||||||
|
try:
|
||||||
|
res_frame,idx,audio_frames = self.res_frame_queue.get(block=True, timeout=1)
|
||||||
|
except queue.Empty:
|
||||||
|
continue
|
||||||
|
if audio_frames[0][1]!=0 and audio_frames[1][1]!=0: #全为静音数据,只需要取fullimg
|
||||||
|
self.speaking = False
|
||||||
|
audiotype = audio_frames[0][1]
|
||||||
|
if self.custom_index.get(audiotype) is not None: #有自定义视频
|
||||||
|
mirindex = self.mirror_index(len(self.custom_img_cycle[audiotype]),self.custom_index[audiotype])
|
||||||
|
combine_frame = self.custom_img_cycle[audiotype][mirindex]
|
||||||
|
self.custom_index[audiotype] += 1
|
||||||
|
# if not self.custom_opt[audiotype].loop and self.custom_index[audiotype]>=len(self.custom_img_cycle[audiotype]):
|
||||||
|
# self.curr_state = 1 #当前视频不循环播放,切换到静音状态
|
||||||
|
else:
|
||||||
|
combine_frame = self.frame_list_cycle[idx]
|
||||||
|
else:
|
||||||
|
self.speaking = True
|
||||||
|
bbox = self.coord_list_cycle[idx]
|
||||||
|
ori_frame = copy.deepcopy(self.frame_list_cycle[idx])
|
||||||
|
x1, y1, x2, y2 = bbox
|
||||||
|
try:
|
||||||
|
res_frame = cv2.resize(res_frame.astype(np.uint8),(x2-x1,y2-y1))
|
||||||
|
except:
|
||||||
|
continue
|
||||||
|
mask = self.mask_list_cycle[idx]
|
||||||
|
mask_crop_box = self.mask_coords_list_cycle[idx]
|
||||||
|
#combine_frame = get_image(ori_frame,res_frame,bbox)
|
||||||
|
#t=time.perf_counter()
|
||||||
|
combine_frame = get_image_blending(ori_frame,res_frame,bbox,mask,mask_crop_box)
|
||||||
|
#print('blending time:',time.perf_counter()-t)
|
||||||
|
|
||||||
|
image = combine_frame #(outputs['image'] * 255).astype(np.uint8)
|
||||||
|
new_frame = VideoFrame.from_ndarray(image, format="bgr24")
|
||||||
|
asyncio.run_coroutine_threadsafe(video_track._queue.put(new_frame), loop)
|
||||||
|
if self.recording:
|
||||||
|
self.recordq_video.put(new_frame)
|
||||||
|
|
||||||
|
for audio_frame in audio_frames:
|
||||||
|
frame,type = audio_frame
|
||||||
|
frame = (frame * 32767).astype(np.int16)
|
||||||
|
new_frame = AudioFrame(format='s16', layout='mono', samples=frame.shape[0])
|
||||||
|
new_frame.planes[0].update(frame.tobytes())
|
||||||
|
new_frame.sample_rate=16000
|
||||||
|
# if audio_track._queue.qsize()>10:
|
||||||
|
# time.sleep(0.1)
|
||||||
|
asyncio.run_coroutine_threadsafe(audio_track._queue.put(new_frame), loop)
|
||||||
|
if self.recording:
|
||||||
|
self.recordq_audio.put(new_frame)
|
||||||
|
print('musereal process_frames thread stop')
|
||||||
|
|
||||||
|
def render(self,quit_event,loop=None,audio_track=None,video_track=None):
|
||||||
|
#if self.opt.asr:
|
||||||
|
# self.asr.warm_up()
|
||||||
|
|
||||||
|
self.tts.render(quit_event)
|
||||||
|
self.init_customindex()
|
||||||
|
process_thread = Thread(target=self.process_frames, args=(quit_event,loop,audio_track,video_track))
|
||||||
|
process_thread.start()
|
||||||
|
|
||||||
|
self.render_event.set() #start infer process render
|
||||||
|
count=0
|
||||||
|
totaltime=0
|
||||||
|
_starttime=time.perf_counter()
|
||||||
|
#_totalframe=0
|
||||||
|
while not quit_event.is_set(): #todo
|
||||||
|
# update texture every frame
|
||||||
|
# audio stream thread...
|
||||||
|
t = time.perf_counter()
|
||||||
|
self.asr.run_step()
|
||||||
|
#self.test_step(loop,audio_track,video_track)
|
||||||
|
# totaltime += (time.perf_counter() - t)
|
||||||
|
# count += self.opt.batch_size
|
||||||
|
# if count>=100:
|
||||||
|
# print(f"------actual avg infer fps:{count/totaltime:.4f}")
|
||||||
|
# count=0
|
||||||
|
# totaltime=0
|
||||||
|
if video_track._queue.qsize()>=1.5*self.opt.batch_size:
|
||||||
|
print('sleep qsize=',video_track._queue.qsize())
|
||||||
|
time.sleep(0.04*video_track._queue.qsize()*0.8)
|
||||||
|
# if video_track._queue.qsize()>=5:
|
||||||
|
# print('sleep qsize=',video_track._queue.qsize())
|
||||||
|
# time.sleep(0.04*video_track._queue.qsize()*0.8)
|
||||||
|
|
||||||
|
# delay = _starttime+_totalframe*0.04-time.perf_counter() #40ms
|
||||||
|
# if delay > 0:
|
||||||
|
# time.sleep(delay)
|
||||||
|
self.render_event.clear() #end infer process render
|
||||||
|
print('musereal thread stop')
|
||||||
|
|
|
@ -0,0 +1,47 @@
|
||||||
|
import torch
|
||||||
|
import torch.nn as nn
|
||||||
|
import math
|
||||||
|
import json
|
||||||
|
|
||||||
|
from diffusers import UNet2DConditionModel
|
||||||
|
import sys
|
||||||
|
import time
|
||||||
|
import numpy as np
|
||||||
|
import os
|
||||||
|
|
||||||
|
class PositionalEncoding(nn.Module):
|
||||||
|
def __init__(self, d_model=384, max_len=5000):
|
||||||
|
super(PositionalEncoding, self).__init__()
|
||||||
|
pe = torch.zeros(max_len, d_model)
|
||||||
|
position = torch.arange(0, max_len, dtype=torch.float).unsqueeze(1)
|
||||||
|
div_term = torch.exp(torch.arange(0, d_model, 2).float() * (-math.log(10000.0) / d_model))
|
||||||
|
pe[:, 0::2] = torch.sin(position * div_term)
|
||||||
|
pe[:, 1::2] = torch.cos(position * div_term)
|
||||||
|
pe = pe.unsqueeze(0)
|
||||||
|
self.register_buffer('pe', pe)
|
||||||
|
|
||||||
|
def forward(self, x):
|
||||||
|
b, seq_len, d_model = x.size()
|
||||||
|
pe = self.pe[:, :seq_len, :]
|
||||||
|
x = x + pe.to(x.device)
|
||||||
|
return x
|
||||||
|
|
||||||
|
class UNet():
|
||||||
|
def __init__(self,
|
||||||
|
unet_config,
|
||||||
|
model_path,
|
||||||
|
use_float16=False,
|
||||||
|
):
|
||||||
|
with open(unet_config, 'r') as f:
|
||||||
|
unet_config = json.load(f)
|
||||||
|
self.model = UNet2DConditionModel(**unet_config)
|
||||||
|
self.pe = PositionalEncoding(d_model=384)
|
||||||
|
self.device = torch.device("cuda" if torch.cuda.is_available() else "cpu")
|
||||||
|
weights = torch.load(model_path) if torch.cuda.is_available() else torch.load(model_path, map_location=self.device)
|
||||||
|
self.model.load_state_dict(weights)
|
||||||
|
if use_float16:
|
||||||
|
self.model = self.model.half()
|
||||||
|
self.model.to(self.device)
|
||||||
|
|
||||||
|
if __name__ == "__main__":
|
||||||
|
unet = UNet()
|
|
@ -0,0 +1,148 @@
|
||||||
|
from diffusers import AutoencoderKL
|
||||||
|
import torch
|
||||||
|
import torchvision.transforms as transforms
|
||||||
|
import torch.nn.functional as F
|
||||||
|
import cv2
|
||||||
|
import numpy as np
|
||||||
|
from PIL import Image
|
||||||
|
import os
|
||||||
|
|
||||||
|
class VAE():
|
||||||
|
"""
|
||||||
|
VAE (Variational Autoencoder) class for image processing.
|
||||||
|
"""
|
||||||
|
|
||||||
|
def __init__(self, model_path="./models/sd-vae-ft-mse/", resized_img=256, use_float16=False):
|
||||||
|
"""
|
||||||
|
Initialize the VAE instance.
|
||||||
|
|
||||||
|
:param model_path: Path to the trained model.
|
||||||
|
:param resized_img: The size to which images are resized.
|
||||||
|
:param use_float16: Whether to use float16 precision.
|
||||||
|
"""
|
||||||
|
self.model_path = model_path
|
||||||
|
self.vae = AutoencoderKL.from_pretrained(self.model_path)
|
||||||
|
|
||||||
|
self.device = torch.device("cuda" if torch.cuda.is_available() else "cpu")
|
||||||
|
self.vae.to(self.device)
|
||||||
|
|
||||||
|
if use_float16:
|
||||||
|
self.vae = self.vae.half()
|
||||||
|
self._use_float16 = True
|
||||||
|
else:
|
||||||
|
self._use_float16 = False
|
||||||
|
|
||||||
|
self.scaling_factor = self.vae.config.scaling_factor
|
||||||
|
self.transform = transforms.Normalize(mean=[0.5, 0.5, 0.5], std=[0.5, 0.5, 0.5])
|
||||||
|
self._resized_img = resized_img
|
||||||
|
self._mask_tensor = self.get_mask_tensor()
|
||||||
|
|
||||||
|
def get_mask_tensor(self):
|
||||||
|
"""
|
||||||
|
Creates a mask tensor for image processing.
|
||||||
|
:return: A mask tensor.
|
||||||
|
"""
|
||||||
|
mask_tensor = torch.zeros((self._resized_img,self._resized_img))
|
||||||
|
mask_tensor[:self._resized_img//2,:] = 1
|
||||||
|
mask_tensor[mask_tensor< 0.5] = 0
|
||||||
|
mask_tensor[mask_tensor>= 0.5] = 1
|
||||||
|
return mask_tensor
|
||||||
|
|
||||||
|
def preprocess_img(self,img_name,half_mask=False):
|
||||||
|
"""
|
||||||
|
Preprocess an image for the VAE.
|
||||||
|
|
||||||
|
:param img_name: The image file path or a list of image file paths.
|
||||||
|
:param half_mask: Whether to apply a half mask to the image.
|
||||||
|
:return: A preprocessed image tensor.
|
||||||
|
"""
|
||||||
|
window = []
|
||||||
|
if isinstance(img_name, str):
|
||||||
|
window_fnames = [img_name]
|
||||||
|
for fname in window_fnames:
|
||||||
|
img = cv2.imread(fname)
|
||||||
|
img = cv2.cvtColor(img, cv2.COLOR_BGR2RGB)
|
||||||
|
img = cv2.resize(img, (self._resized_img, self._resized_img),
|
||||||
|
interpolation=cv2.INTER_LANCZOS4)
|
||||||
|
window.append(img)
|
||||||
|
else:
|
||||||
|
img = cv2.cvtColor(img_name, cv2.COLOR_BGR2RGB)
|
||||||
|
window.append(img)
|
||||||
|
|
||||||
|
x = np.asarray(window) / 255.
|
||||||
|
x = np.transpose(x, (3, 0, 1, 2))
|
||||||
|
x = torch.squeeze(torch.FloatTensor(x))
|
||||||
|
if half_mask:
|
||||||
|
x = x * (self._mask_tensor>0.5)
|
||||||
|
x = self.transform(x)
|
||||||
|
|
||||||
|
x = x.unsqueeze(0) # [1, 3, 256, 256] torch tensor
|
||||||
|
x = x.to(self.vae.device)
|
||||||
|
|
||||||
|
return x
|
||||||
|
|
||||||
|
def encode_latents(self,image):
|
||||||
|
"""
|
||||||
|
Encode an image into latent variables.
|
||||||
|
|
||||||
|
:param image: The image tensor to encode.
|
||||||
|
:return: The encoded latent variables.
|
||||||
|
"""
|
||||||
|
with torch.no_grad():
|
||||||
|
init_latent_dist = self.vae.encode(image.to(self.vae.dtype)).latent_dist
|
||||||
|
init_latents = self.scaling_factor * init_latent_dist.sample()
|
||||||
|
return init_latents
|
||||||
|
|
||||||
|
def decode_latents(self, latents):
|
||||||
|
"""
|
||||||
|
Decode latent variables back into an image.
|
||||||
|
:param latents: The latent variables to decode.
|
||||||
|
:return: A NumPy array representing the decoded image.
|
||||||
|
"""
|
||||||
|
latents = (1/ self.scaling_factor) * latents
|
||||||
|
image = self.vae.decode(latents.to(self.vae.dtype)).sample
|
||||||
|
image = (image / 2 + 0.5).clamp(0, 1)
|
||||||
|
image = image.detach().cpu().permute(0, 2, 3, 1).float().numpy()
|
||||||
|
image = (image * 255).round().astype("uint8")
|
||||||
|
image = image[...,::-1] # RGB to BGR
|
||||||
|
return image
|
||||||
|
|
||||||
|
def get_latents_for_unet(self,img):
|
||||||
|
"""
|
||||||
|
Prepare latent variables for a U-Net model.
|
||||||
|
:param img: The image to process.
|
||||||
|
:return: A concatenated tensor of latents for U-Net input.
|
||||||
|
"""
|
||||||
|
|
||||||
|
ref_image = self.preprocess_img(img,half_mask=True) # [1, 3, 256, 256] RGB, torch tensor
|
||||||
|
masked_latents = self.encode_latents(ref_image) # [1, 4, 32, 32], torch tensor
|
||||||
|
ref_image = self.preprocess_img(img,half_mask=False) # [1, 3, 256, 256] RGB, torch tensor
|
||||||
|
ref_latents = self.encode_latents(ref_image) # [1, 4, 32, 32], torch tensor
|
||||||
|
latent_model_input = torch.cat([masked_latents, ref_latents], dim=1)
|
||||||
|
return latent_model_input
|
||||||
|
|
||||||
|
if __name__ == "__main__":
|
||||||
|
vae_mode_path = "./models/sd-vae-ft-mse/"
|
||||||
|
vae = VAE(model_path = vae_mode_path,use_float16=False)
|
||||||
|
img_path = "./results/sun001_crop/00000.png"
|
||||||
|
|
||||||
|
crop_imgs_path = "./results/sun001_crop/"
|
||||||
|
latents_out_path = "./results/latents/"
|
||||||
|
if not os.path.exists(latents_out_path):
|
||||||
|
os.mkdir(latents_out_path)
|
||||||
|
|
||||||
|
files = os.listdir(crop_imgs_path)
|
||||||
|
files.sort()
|
||||||
|
files = [file for file in files if file.split(".")[-1] == "png"]
|
||||||
|
|
||||||
|
for file in files:
|
||||||
|
index = file.split(".")[0]
|
||||||
|
img_path = crop_imgs_path + file
|
||||||
|
latents = vae.get_latents_for_unet(img_path)
|
||||||
|
print(img_path,"latents",latents.size())
|
||||||
|
#torch.save(latents,os.path.join(latents_out_path,index+".pt"))
|
||||||
|
#reload_tensor = torch.load('tensor.pt')
|
||||||
|
#print(reload_tensor.size())
|
||||||
|
|
||||||
|
|
||||||
|
|
|
@ -0,0 +1,348 @@
|
||||||
|
import argparse
|
||||||
|
import glob
|
||||||
|
import json
|
||||||
|
import os
|
||||||
|
import pickle
|
||||||
|
import shutil
|
||||||
|
|
||||||
|
import cv2
|
||||||
|
import numpy as np
|
||||||
|
import torch
|
||||||
|
import torchvision.transforms as transforms
|
||||||
|
from PIL import Image
|
||||||
|
from diffusers import AutoencoderKL
|
||||||
|
from face_alignment import NetworkSize
|
||||||
|
from mmpose.apis import inference_topdown, init_model
|
||||||
|
from mmpose.structures import merge_data_samples
|
||||||
|
from tqdm import tqdm
|
||||||
|
|
||||||
|
try:
|
||||||
|
from utils.face_parsing import FaceParsing
|
||||||
|
except ModuleNotFoundError:
|
||||||
|
from musetalk.utils.face_parsing import FaceParsing
|
||||||
|
|
||||||
|
|
||||||
|
def video2imgs(vid_path, save_path, ext='.png', cut_frame=10000000):
|
||||||
|
cap = cv2.VideoCapture(vid_path)
|
||||||
|
count = 0
|
||||||
|
while True:
|
||||||
|
if count > cut_frame:
|
||||||
|
break
|
||||||
|
ret, frame = cap.read()
|
||||||
|
if ret:
|
||||||
|
cv2.imwrite(f"{save_path}/{count:08d}.png", frame)
|
||||||
|
count += 1
|
||||||
|
else:
|
||||||
|
break
|
||||||
|
|
||||||
|
|
||||||
|
def read_imgs(img_list):
|
||||||
|
frames = []
|
||||||
|
print('reading images...')
|
||||||
|
for img_path in tqdm(img_list):
|
||||||
|
frame = cv2.imread(img_path)
|
||||||
|
frames.append(frame)
|
||||||
|
return frames
|
||||||
|
|
||||||
|
|
||||||
|
def get_landmark_and_bbox(img_list, upperbondrange=0):
|
||||||
|
frames = read_imgs(img_list)
|
||||||
|
batch_size_fa = 1
|
||||||
|
batches = [frames[i:i + batch_size_fa] for i in range(0, len(frames), batch_size_fa)]
|
||||||
|
coords_list = []
|
||||||
|
landmarks = []
|
||||||
|
if upperbondrange != 0:
|
||||||
|
print('get key_landmark and face bounding boxes with the bbox_shift:', upperbondrange)
|
||||||
|
else:
|
||||||
|
print('get key_landmark and face bounding boxes with the default value')
|
||||||
|
average_range_minus = []
|
||||||
|
average_range_plus = []
|
||||||
|
coord_placeholder = (0.0, 0.0, 0.0, 0.0)
|
||||||
|
for fb in tqdm(batches):
|
||||||
|
results = inference_topdown(model, np.asarray(fb)[0])
|
||||||
|
results = merge_data_samples(results)
|
||||||
|
keypoints = results.pred_instances.keypoints
|
||||||
|
face_land_mark = keypoints[0][23:91]
|
||||||
|
face_land_mark = face_land_mark.astype(np.int32)
|
||||||
|
|
||||||
|
# get bounding boxes by face detetion
|
||||||
|
bbox = fa.get_detections_for_batch(np.asarray(fb))
|
||||||
|
|
||||||
|
# adjust the bounding box refer to landmark
|
||||||
|
# Add the bounding box to a tuple and append it to the coordinates list
|
||||||
|
for j, f in enumerate(bbox):
|
||||||
|
if f is None: # no face in the image
|
||||||
|
coords_list += [coord_placeholder]
|
||||||
|
continue
|
||||||
|
|
||||||
|
half_face_coord = face_land_mark[29] # np.mean([face_land_mark[28], face_land_mark[29]], axis=0)
|
||||||
|
range_minus = (face_land_mark[30] - face_land_mark[29])[1]
|
||||||
|
range_plus = (face_land_mark[29] - face_land_mark[28])[1]
|
||||||
|
average_range_minus.append(range_minus)
|
||||||
|
average_range_plus.append(range_plus)
|
||||||
|
if upperbondrange != 0:
|
||||||
|
half_face_coord[1] = upperbondrange + half_face_coord[1] # 手动调整 + 向下(偏29) - 向上(偏28)
|
||||||
|
half_face_dist = np.max(face_land_mark[:, 1]) - half_face_coord[1]
|
||||||
|
upper_bond = half_face_coord[1] - half_face_dist
|
||||||
|
|
||||||
|
f_landmark = (
|
||||||
|
np.min(face_land_mark[:, 0]), int(upper_bond), np.max(face_land_mark[:, 0]),
|
||||||
|
np.max(face_land_mark[:, 1]))
|
||||||
|
x1, y1, x2, y2 = f_landmark
|
||||||
|
|
||||||
|
if y2 - y1 <= 0 or x2 - x1 <= 0 or x1 < 0: # if the landmark bbox is not suitable, reuse the bbox
|
||||||
|
coords_list += [f]
|
||||||
|
w, h = f[2] - f[0], f[3] - f[1]
|
||||||
|
print("error bbox:", f)
|
||||||
|
else:
|
||||||
|
coords_list += [f_landmark]
|
||||||
|
return coords_list, frames
|
||||||
|
|
||||||
|
|
||||||
|
class FaceAlignment:
|
||||||
|
def __init__(self, landmarks_type, network_size=NetworkSize.LARGE,
|
||||||
|
device='cuda', flip_input=False, face_detector='sfd', verbose=False):
|
||||||
|
self.device = device
|
||||||
|
self.flip_input = flip_input
|
||||||
|
self.landmarks_type = landmarks_type
|
||||||
|
self.verbose = verbose
|
||||||
|
|
||||||
|
network_size = int(network_size)
|
||||||
|
if 'cuda' in device:
|
||||||
|
torch.backends.cudnn.benchmark = True
|
||||||
|
# torch.backends.cuda.matmul.allow_tf32 = False
|
||||||
|
# torch.backends.cudnn.benchmark = True
|
||||||
|
# torch.backends.cudnn.deterministic = False
|
||||||
|
# torch.backends.cudnn.allow_tf32 = True
|
||||||
|
print('cuda start')
|
||||||
|
|
||||||
|
# Get the face detector
|
||||||
|
face_detector_module = __import__('face_detection.detection.' + face_detector,
|
||||||
|
globals(), locals(), [face_detector], 0)
|
||||||
|
|
||||||
|
self.face_detector = face_detector_module.FaceDetector(device=device, verbose=verbose)
|
||||||
|
|
||||||
|
def get_detections_for_batch(self, images):
|
||||||
|
images = images[..., ::-1]
|
||||||
|
detected_faces = self.face_detector.detect_from_batch(images.copy())
|
||||||
|
results = []
|
||||||
|
|
||||||
|
for i, d in enumerate(detected_faces):
|
||||||
|
if len(d) == 0:
|
||||||
|
results.append(None)
|
||||||
|
continue
|
||||||
|
d = d[0]
|
||||||
|
d = np.clip(d, 0, None)
|
||||||
|
|
||||||
|
x1, y1, x2, y2 = map(int, d[:-1])
|
||||||
|
results.append((x1, y1, x2, y2))
|
||||||
|
return results
|
||||||
|
|
||||||
|
|
||||||
|
def get_mask_tensor():
|
||||||
|
"""
|
||||||
|
Creates a mask tensor for image processing.
|
||||||
|
:return: A mask tensor.
|
||||||
|
"""
|
||||||
|
mask_tensor = torch.zeros((256, 256))
|
||||||
|
mask_tensor[:256 // 2, :] = 1
|
||||||
|
mask_tensor[mask_tensor < 0.5] = 0
|
||||||
|
mask_tensor[mask_tensor >= 0.5] = 1
|
||||||
|
return mask_tensor
|
||||||
|
|
||||||
|
|
||||||
|
def preprocess_img(img_name, half_mask=False):
|
||||||
|
window = []
|
||||||
|
if isinstance(img_name, str):
|
||||||
|
window_fnames = [img_name]
|
||||||
|
for fname in window_fnames:
|
||||||
|
img = cv2.imread(fname)
|
||||||
|
img = cv2.cvtColor(img, cv2.COLOR_BGR2RGB)
|
||||||
|
img = cv2.resize(img, (256, 256),
|
||||||
|
interpolation=cv2.INTER_LANCZOS4)
|
||||||
|
window.append(img)
|
||||||
|
else:
|
||||||
|
img = cv2.cvtColor(img_name, cv2.COLOR_BGR2RGB)
|
||||||
|
window.append(img)
|
||||||
|
x = np.asarray(window) / 255.
|
||||||
|
x = np.transpose(x, (3, 0, 1, 2))
|
||||||
|
x = torch.squeeze(torch.FloatTensor(x))
|
||||||
|
if half_mask:
|
||||||
|
x = x * (get_mask_tensor() > 0.5)
|
||||||
|
normalize = transforms.Normalize(mean=[0.5, 0.5, 0.5], std=[0.5, 0.5, 0.5])
|
||||||
|
x = normalize(x)
|
||||||
|
x = x.unsqueeze(0) # [1, 3, 256, 256] torch tensor
|
||||||
|
x = x.to(device)
|
||||||
|
return x
|
||||||
|
|
||||||
|
|
||||||
|
def encode_latents(image):
|
||||||
|
with torch.no_grad():
|
||||||
|
init_latent_dist = vae.encode(image.to(vae.dtype)).latent_dist
|
||||||
|
init_latents = vae.config.scaling_factor * init_latent_dist.sample()
|
||||||
|
return init_latents
|
||||||
|
|
||||||
|
|
||||||
|
def get_latents_for_unet(img):
|
||||||
|
ref_image = preprocess_img(img, half_mask=True) # [1, 3, 256, 256] RGB, torch tensor
|
||||||
|
masked_latents = encode_latents(ref_image) # [1, 4, 32, 32], torch tensor
|
||||||
|
ref_image = preprocess_img(img, half_mask=False) # [1, 3, 256, 256] RGB, torch tensor
|
||||||
|
ref_latents = encode_latents(ref_image) # [1, 4, 32, 32], torch tensor
|
||||||
|
latent_model_input = torch.cat([masked_latents, ref_latents], dim=1)
|
||||||
|
return latent_model_input
|
||||||
|
|
||||||
|
|
||||||
|
def get_crop_box(box, expand):
|
||||||
|
x, y, x1, y1 = box
|
||||||
|
x_c, y_c = (x + x1) // 2, (y + y1) // 2
|
||||||
|
w, h = x1 - x, y1 - y
|
||||||
|
s = int(max(w, h) // 2 * expand)
|
||||||
|
crop_box = [x_c - s, y_c - s, x_c + s, y_c + s]
|
||||||
|
return crop_box, s
|
||||||
|
|
||||||
|
|
||||||
|
def face_seg(image):
|
||||||
|
seg_image = fp(image)
|
||||||
|
if seg_image is None:
|
||||||
|
print("error, no person_segment")
|
||||||
|
return None
|
||||||
|
|
||||||
|
seg_image = seg_image.resize(image.size)
|
||||||
|
return seg_image
|
||||||
|
|
||||||
|
|
||||||
|
def get_image_prepare_material(image, face_box, upper_boundary_ratio=0.5, expand=1.2):
|
||||||
|
body = Image.fromarray(image[:, :, ::-1])
|
||||||
|
|
||||||
|
x, y, x1, y1 = face_box
|
||||||
|
# print(x1-x,y1-y)
|
||||||
|
crop_box, s = get_crop_box(face_box, expand)
|
||||||
|
x_s, y_s, x_e, y_e = crop_box
|
||||||
|
|
||||||
|
face_large = body.crop(crop_box)
|
||||||
|
ori_shape = face_large.size
|
||||||
|
|
||||||
|
mask_image = face_seg(face_large)
|
||||||
|
mask_small = mask_image.crop((x - x_s, y - y_s, x1 - x_s, y1 - y_s))
|
||||||
|
mask_image = Image.new('L', ori_shape, 0)
|
||||||
|
mask_image.paste(mask_small, (x - x_s, y - y_s, x1 - x_s, y1 - y_s))
|
||||||
|
|
||||||
|
# keep upper_boundary_ratio of talking area
|
||||||
|
width, height = mask_image.size
|
||||||
|
top_boundary = int(height * upper_boundary_ratio)
|
||||||
|
modified_mask_image = Image.new('L', ori_shape, 0)
|
||||||
|
modified_mask_image.paste(mask_image.crop((0, top_boundary, width, height)), (0, top_boundary))
|
||||||
|
|
||||||
|
blur_kernel_size = int(0.1 * ori_shape[0] // 2 * 2) + 1
|
||||||
|
mask_array = cv2.GaussianBlur(np.array(modified_mask_image), (blur_kernel_size, blur_kernel_size), 0)
|
||||||
|
return mask_array, crop_box
|
||||||
|
|
||||||
|
|
||||||
|
##todo 简单根据文件后缀判断 要更精确的可以自己修改 使用 magic
|
||||||
|
def is_video_file(file_path):
|
||||||
|
video_exts = ['.mp4', '.mkv', '.flv', '.avi', '.mov'] # 这里列出了一些常见的视频文件扩展名,可以根据需要添加更多
|
||||||
|
file_ext = os.path.splitext(file_path)[1].lower() # 获取文件扩展名并转换为小写
|
||||||
|
return file_ext in video_exts
|
||||||
|
|
||||||
|
|
||||||
|
def create_dir(dir_path):
|
||||||
|
if not os.path.exists(dir_path):
|
||||||
|
os.makedirs(dir_path)
|
||||||
|
|
||||||
|
|
||||||
|
current_dir = os.path.dirname(os.path.abspath(__file__))
|
||||||
|
|
||||||
|
|
||||||
|
def create_musetalk_human(file, avatar_id):
|
||||||
|
# 保存文件设置 可以不动
|
||||||
|
save_path = os.path.join(current_dir, f'../data/avatars/avator_{avatar_id}')
|
||||||
|
save_full_path = os.path.join(current_dir, f'../data/avatars/avator_{avatar_id}/full_imgs')
|
||||||
|
create_dir(save_path)
|
||||||
|
create_dir(save_full_path)
|
||||||
|
mask_out_path = os.path.join(current_dir, f'../data/avatars/avator_{avatar_id}/mask')
|
||||||
|
create_dir(mask_out_path)
|
||||||
|
|
||||||
|
# 模型
|
||||||
|
mask_coords_path = os.path.join(current_dir, f'{save_path}/mask_coords.pkl')
|
||||||
|
coords_path = os.path.join(current_dir, f'{save_path}/coords.pkl')
|
||||||
|
latents_out_path = os.path.join(current_dir, f'{save_path}/latents.pt')
|
||||||
|
|
||||||
|
with open(os.path.join(current_dir, f'{save_path}/avator_info.json'), "w") as f:
|
||||||
|
json.dump({
|
||||||
|
"avatar_id": avatar_id,
|
||||||
|
"video_path": file,
|
||||||
|
"bbox_shift": 5
|
||||||
|
}, f)
|
||||||
|
|
||||||
|
if os.path.isfile(file):
|
||||||
|
if is_video_file(file):
|
||||||
|
video2imgs(file, save_full_path, ext='png')
|
||||||
|
else:
|
||||||
|
shutil.copyfile(file, f"{save_full_path}/{os.path.basename(file)}")
|
||||||
|
else:
|
||||||
|
files = os.listdir(file)
|
||||||
|
files.sort()
|
||||||
|
files = [file for file in files if file.split(".")[-1] == "png"]
|
||||||
|
for filename in files:
|
||||||
|
shutil.copyfile(f"{file}/{filename}", f"{save_full_path}/{filename}")
|
||||||
|
input_img_list = sorted(glob.glob(os.path.join(save_full_path, '*.[jpJP][pnPN]*[gG]')))
|
||||||
|
print("extracting landmarks...")
|
||||||
|
coord_list, frame_list = get_landmark_and_bbox(input_img_list, 5)
|
||||||
|
input_latent_list = []
|
||||||
|
idx = -1
|
||||||
|
# maker if the bbox is not sufficient
|
||||||
|
coord_placeholder = (0.0, 0.0, 0.0, 0.0)
|
||||||
|
for bbox, frame in zip(coord_list, frame_list):
|
||||||
|
idx = idx + 1
|
||||||
|
if bbox == coord_placeholder:
|
||||||
|
continue
|
||||||
|
x1, y1, x2, y2 = bbox
|
||||||
|
crop_frame = frame[y1:y2, x1:x2]
|
||||||
|
resized_crop_frame = cv2.resize(crop_frame, (256, 256), interpolation=cv2.INTER_LANCZOS4)
|
||||||
|
latents = get_latents_for_unet(resized_crop_frame)
|
||||||
|
input_latent_list.append(latents)
|
||||||
|
|
||||||
|
frame_list_cycle = frame_list #+ frame_list[::-1]
|
||||||
|
coord_list_cycle = coord_list #+ coord_list[::-1]
|
||||||
|
input_latent_list_cycle = input_latent_list #+ input_latent_list[::-1]
|
||||||
|
mask_coords_list_cycle = []
|
||||||
|
mask_list_cycle = []
|
||||||
|
for i, frame in enumerate(tqdm(frame_list_cycle)):
|
||||||
|
cv2.imwrite(f"{save_full_path}/{str(i).zfill(8)}.png", frame)
|
||||||
|
face_box = coord_list_cycle[i]
|
||||||
|
mask, crop_box = get_image_prepare_material(frame, face_box)
|
||||||
|
cv2.imwrite(f"{mask_out_path}/{str(i).zfill(8)}.png", mask)
|
||||||
|
mask_coords_list_cycle += [crop_box]
|
||||||
|
mask_list_cycle.append(mask)
|
||||||
|
|
||||||
|
with open(mask_coords_path, 'wb') as f:
|
||||||
|
pickle.dump(mask_coords_list_cycle, f)
|
||||||
|
|
||||||
|
with open(coords_path, 'wb') as f:
|
||||||
|
pickle.dump(coord_list_cycle, f)
|
||||||
|
torch.save(input_latent_list_cycle, os.path.join(latents_out_path))
|
||||||
|
|
||||||
|
|
||||||
|
# initialize the mmpose model
|
||||||
|
device = "cuda" if torch.cuda.is_available() else "cpu"
|
||||||
|
fa = FaceAlignment(1, flip_input=False, device=device)
|
||||||
|
config_file = os.path.join(current_dir, 'utils/dwpose/rtmpose-l_8xb32-270e_coco-ubody-wholebody-384x288.py')
|
||||||
|
checkpoint_file = os.path.abspath(os.path.join(current_dir, '../models/dwpose/dw-ll_ucoco_384.pth'))
|
||||||
|
model = init_model(config_file, checkpoint_file, device=device)
|
||||||
|
vae = AutoencoderKL.from_pretrained(os.path.abspath(os.path.join(current_dir, '../models/sd-vae-ft-mse')))
|
||||||
|
vae.to(device)
|
||||||
|
fp = FaceParsing(os.path.abspath(os.path.join(current_dir, '../models/face-parse-bisent/resnet18-5c106cde.pth')),
|
||||||
|
os.path.abspath(os.path.join(current_dir, '../models/face-parse-bisent/79999_iter.pth')))
|
||||||
|
if __name__ == '__main__':
|
||||||
|
# 视频文件地址
|
||||||
|
parser = argparse.ArgumentParser()
|
||||||
|
parser.add_argument("--file",
|
||||||
|
type=str,
|
||||||
|
default=r'D:\ok\00000000.png',
|
||||||
|
)
|
||||||
|
parser.add_argument("--avatar_id",
|
||||||
|
type=str,
|
||||||
|
default='3',
|
||||||
|
)
|
||||||
|
args = parser.parse_args()
|
||||||
|
create_musetalk_human(args.file, args.avatar_id)
|
|
@ -0,0 +1,5 @@
|
||||||
|
import sys
|
||||||
|
from os.path import abspath, dirname
|
||||||
|
current_dir = dirname(abspath(__file__))
|
||||||
|
parent_dir = dirname(current_dir)
|
||||||
|
sys.path.append(parent_dir+'/utils')
|
|
@ -0,0 +1,125 @@
|
||||||
|
from PIL import Image
|
||||||
|
import numpy as np
|
||||||
|
import cv2
|
||||||
|
from face_parsing import FaceParsing
|
||||||
|
import copy
|
||||||
|
|
||||||
|
fp = FaceParsing()
|
||||||
|
|
||||||
|
def get_crop_box(box, expand):
|
||||||
|
x, y, x1, y1 = box
|
||||||
|
x_c, y_c = (x+x1)//2, (y+y1)//2
|
||||||
|
w, h = x1-x, y1-y
|
||||||
|
s = int(max(w, h)//2*expand)
|
||||||
|
crop_box = [x_c-s, y_c-s, x_c+s, y_c+s]
|
||||||
|
return crop_box, s
|
||||||
|
|
||||||
|
def face_seg(image):
|
||||||
|
seg_image = fp(image)
|
||||||
|
if seg_image is None:
|
||||||
|
print("error, no person_segment")
|
||||||
|
return None
|
||||||
|
|
||||||
|
seg_image = seg_image.resize(image.size)
|
||||||
|
return seg_image
|
||||||
|
|
||||||
|
def get_image(image,face,face_box,upper_boundary_ratio = 0.5,expand=1.2):
|
||||||
|
#print(image.shape)
|
||||||
|
#print(face.shape)
|
||||||
|
|
||||||
|
body = Image.fromarray(image[:,:,::-1])
|
||||||
|
face = Image.fromarray(face[:,:,::-1])
|
||||||
|
|
||||||
|
x, y, x1, y1 = face_box
|
||||||
|
#print(x1-x,y1-y)
|
||||||
|
crop_box, s = get_crop_box(face_box, expand)
|
||||||
|
x_s, y_s, x_e, y_e = crop_box
|
||||||
|
face_position = (x, y)
|
||||||
|
|
||||||
|
face_large = body.crop(crop_box)
|
||||||
|
ori_shape = face_large.size
|
||||||
|
|
||||||
|
mask_image = face_seg(face_large)
|
||||||
|
mask_small = mask_image.crop((x-x_s, y-y_s, x1-x_s, y1-y_s))
|
||||||
|
mask_image = Image.new('L', ori_shape, 0)
|
||||||
|
mask_image.paste(mask_small, (x-x_s, y-y_s, x1-x_s, y1-y_s))
|
||||||
|
|
||||||
|
# keep upper_boundary_ratio of talking area
|
||||||
|
width, height = mask_image.size
|
||||||
|
top_boundary = int(height * upper_boundary_ratio)
|
||||||
|
modified_mask_image = Image.new('L', ori_shape, 0)
|
||||||
|
modified_mask_image.paste(mask_image.crop((0, top_boundary, width, height)), (0, top_boundary))
|
||||||
|
|
||||||
|
blur_kernel_size = int(0.1 * ori_shape[0] // 2 * 2) + 1
|
||||||
|
mask_array = cv2.GaussianBlur(np.array(modified_mask_image), (blur_kernel_size, blur_kernel_size), 0)
|
||||||
|
mask_image = Image.fromarray(mask_array)
|
||||||
|
|
||||||
|
face_large.paste(face, (x-x_s, y-y_s, x1-x_s, y1-y_s))
|
||||||
|
body.paste(face_large, crop_box[:2], mask_image)
|
||||||
|
body = np.array(body)
|
||||||
|
return body[:,:,::-1]
|
||||||
|
|
||||||
|
def get_image_prepare_material(image,face_box,upper_boundary_ratio = 0.5,expand=1.2):
|
||||||
|
body = Image.fromarray(image[:,:,::-1])
|
||||||
|
|
||||||
|
x, y, x1, y1 = face_box
|
||||||
|
#print(x1-x,y1-y)
|
||||||
|
crop_box, s = get_crop_box(face_box, expand)
|
||||||
|
x_s, y_s, x_e, y_e = crop_box
|
||||||
|
|
||||||
|
face_large = body.crop(crop_box)
|
||||||
|
ori_shape = face_large.size
|
||||||
|
|
||||||
|
mask_image = face_seg(face_large)
|
||||||
|
mask_small = mask_image.crop((x-x_s, y-y_s, x1-x_s, y1-y_s))
|
||||||
|
mask_image = Image.new('L', ori_shape, 0)
|
||||||
|
mask_image.paste(mask_small, (x-x_s, y-y_s, x1-x_s, y1-y_s))
|
||||||
|
|
||||||
|
# keep upper_boundary_ratio of talking area
|
||||||
|
width, height = mask_image.size
|
||||||
|
top_boundary = int(height * upper_boundary_ratio)
|
||||||
|
modified_mask_image = Image.new('L', ori_shape, 0)
|
||||||
|
modified_mask_image.paste(mask_image.crop((0, top_boundary, width, height)), (0, top_boundary))
|
||||||
|
|
||||||
|
blur_kernel_size = int(0.1 * ori_shape[0] // 2 * 2) + 1
|
||||||
|
mask_array = cv2.GaussianBlur(np.array(modified_mask_image), (blur_kernel_size, blur_kernel_size), 0)
|
||||||
|
return mask_array,crop_box
|
||||||
|
|
||||||
|
# def get_image_blending(image,face,face_box,mask_array,crop_box):
|
||||||
|
# body = Image.fromarray(image[:,:,::-1])
|
||||||
|
# face = Image.fromarray(face[:,:,::-1])
|
||||||
|
|
||||||
|
# x, y, x1, y1 = face_box
|
||||||
|
# x_s, y_s, x_e, y_e = crop_box
|
||||||
|
# face_large = body.crop(crop_box)
|
||||||
|
|
||||||
|
# mask_image = Image.fromarray(mask_array)
|
||||||
|
# mask_image = mask_image.convert("L")
|
||||||
|
# face_large.paste(face, (x-x_s, y-y_s, x1-x_s, y1-y_s))
|
||||||
|
# body.paste(face_large, crop_box[:2], mask_image)
|
||||||
|
# body = np.array(body)
|
||||||
|
# return body[:,:,::-1]
|
||||||
|
|
||||||
|
def get_image_blending(image,face,face_box,mask_array,crop_box):
|
||||||
|
body = image
|
||||||
|
x, y, x1, y1 = face_box
|
||||||
|
x_s, y_s, x_e, y_e = crop_box
|
||||||
|
face_large = copy.deepcopy(body[y_s:y_e, x_s:x_e])
|
||||||
|
face_large[y-y_s:y1-y_s, x-x_s:x1-x_s]=face
|
||||||
|
|
||||||
|
mask_image = cv2.cvtColor(mask_array,cv2.COLOR_BGR2GRAY)
|
||||||
|
mask_image = (mask_image/255).astype(np.float32)
|
||||||
|
|
||||||
|
# mask_not = cv2.bitwise_not(mask_array)
|
||||||
|
# prospect_tmp = cv2.bitwise_and(face_large, face_large, mask=mask_array)
|
||||||
|
# background_img = body[y_s:y_e, x_s:x_e]
|
||||||
|
# background_img = cv2.bitwise_and(background_img, background_img, mask=mask_not)
|
||||||
|
# body[y_s:y_e, x_s:x_e] = prospect_tmp + background_img
|
||||||
|
|
||||||
|
#print(mask_image.shape)
|
||||||
|
#print(cv2.minMaxLoc(mask_image))
|
||||||
|
|
||||||
|
body[y_s:y_e, x_s:x_e] = cv2.blendLinear(face_large,body[y_s:y_e, x_s:x_e],mask_image,1-mask_image)
|
||||||
|
|
||||||
|
#body.paste(face_large, crop_box[:2], mask_image)
|
||||||
|
return body
|
|
@ -0,0 +1,54 @@
|
||||||
|
default_scope = 'mmpose'
|
||||||
|
|
||||||
|
# hooks
|
||||||
|
default_hooks = dict(
|
||||||
|
timer=dict(type='IterTimerHook'),
|
||||||
|
logger=dict(type='LoggerHook', interval=50),
|
||||||
|
param_scheduler=dict(type='ParamSchedulerHook'),
|
||||||
|
checkpoint=dict(type='CheckpointHook', interval=10),
|
||||||
|
sampler_seed=dict(type='DistSamplerSeedHook'),
|
||||||
|
visualization=dict(type='PoseVisualizationHook', enable=False),
|
||||||
|
badcase=dict(
|
||||||
|
type='BadCaseAnalysisHook',
|
||||||
|
enable=False,
|
||||||
|
out_dir='badcase',
|
||||||
|
metric_type='loss',
|
||||||
|
badcase_thr=5))
|
||||||
|
|
||||||
|
# custom hooks
|
||||||
|
custom_hooks = [
|
||||||
|
# Synchronize model buffers such as running_mean and running_var in BN
|
||||||
|
# at the end of each epoch
|
||||||
|
dict(type='SyncBuffersHook')
|
||||||
|
]
|
||||||
|
|
||||||
|
# multi-processing backend
|
||||||
|
env_cfg = dict(
|
||||||
|
cudnn_benchmark=False,
|
||||||
|
mp_cfg=dict(mp_start_method='fork', opencv_num_threads=0),
|
||||||
|
dist_cfg=dict(backend='nccl'),
|
||||||
|
)
|
||||||
|
|
||||||
|
# visualizer
|
||||||
|
vis_backends = [
|
||||||
|
dict(type='LocalVisBackend'),
|
||||||
|
# dict(type='TensorboardVisBackend'),
|
||||||
|
# dict(type='WandbVisBackend'),
|
||||||
|
]
|
||||||
|
visualizer = dict(
|
||||||
|
type='PoseLocalVisualizer', vis_backends=vis_backends, name='visualizer')
|
||||||
|
|
||||||
|
# logger
|
||||||
|
log_processor = dict(
|
||||||
|
type='LogProcessor', window_size=50, by_epoch=True, num_digits=6)
|
||||||
|
log_level = 'INFO'
|
||||||
|
load_from = None
|
||||||
|
resume = False
|
||||||
|
|
||||||
|
# file I/O backend
|
||||||
|
backend_args = dict(backend='local')
|
||||||
|
|
||||||
|
# training/validation/testing progress
|
||||||
|
train_cfg = dict(by_epoch=True)
|
||||||
|
val_cfg = dict()
|
||||||
|
test_cfg = dict()
|
|
@ -0,0 +1,257 @@
|
||||||
|
#_base_ = ['../../../_base_/default_runtime.py']
|
||||||
|
_base_ = ['default_runtime.py']
|
||||||
|
|
||||||
|
# runtime
|
||||||
|
max_epochs = 270
|
||||||
|
stage2_num_epochs = 30
|
||||||
|
base_lr = 4e-3
|
||||||
|
train_batch_size = 32
|
||||||
|
val_batch_size = 32
|
||||||
|
|
||||||
|
train_cfg = dict(max_epochs=max_epochs, val_interval=10)
|
||||||
|
randomness = dict(seed=21)
|
||||||
|
|
||||||
|
# optimizer
|
||||||
|
optim_wrapper = dict(
|
||||||
|
type='OptimWrapper',
|
||||||
|
optimizer=dict(type='AdamW', lr=base_lr, weight_decay=0.05),
|
||||||
|
paramwise_cfg=dict(
|
||||||
|
norm_decay_mult=0, bias_decay_mult=0, bypass_duplicate=True))
|
||||||
|
|
||||||
|
# learning rate
|
||||||
|
param_scheduler = [
|
||||||
|
dict(
|
||||||
|
type='LinearLR',
|
||||||
|
start_factor=1.0e-5,
|
||||||
|
by_epoch=False,
|
||||||
|
begin=0,
|
||||||
|
end=1000),
|
||||||
|
dict(
|
||||||
|
# use cosine lr from 150 to 300 epoch
|
||||||
|
type='CosineAnnealingLR',
|
||||||
|
eta_min=base_lr * 0.05,
|
||||||
|
begin=max_epochs // 2,
|
||||||
|
end=max_epochs,
|
||||||
|
T_max=max_epochs // 2,
|
||||||
|
by_epoch=True,
|
||||||
|
convert_to_iter_based=True),
|
||||||
|
]
|
||||||
|
|
||||||
|
# automatically scaling LR based on the actual training batch size
|
||||||
|
auto_scale_lr = dict(base_batch_size=512)
|
||||||
|
|
||||||
|
# codec settings
|
||||||
|
codec = dict(
|
||||||
|
type='SimCCLabel',
|
||||||
|
input_size=(288, 384),
|
||||||
|
sigma=(6., 6.93),
|
||||||
|
simcc_split_ratio=2.0,
|
||||||
|
normalize=False,
|
||||||
|
use_dark=False)
|
||||||
|
|
||||||
|
# model settings
|
||||||
|
model = dict(
|
||||||
|
type='TopdownPoseEstimator',
|
||||||
|
data_preprocessor=dict(
|
||||||
|
type='PoseDataPreprocessor',
|
||||||
|
mean=[123.675, 116.28, 103.53],
|
||||||
|
std=[58.395, 57.12, 57.375],
|
||||||
|
bgr_to_rgb=True),
|
||||||
|
backbone=dict(
|
||||||
|
_scope_='mmdet',
|
||||||
|
type='CSPNeXt',
|
||||||
|
arch='P5',
|
||||||
|
expand_ratio=0.5,
|
||||||
|
deepen_factor=1.,
|
||||||
|
widen_factor=1.,
|
||||||
|
out_indices=(4, ),
|
||||||
|
channel_attention=True,
|
||||||
|
norm_cfg=dict(type='SyncBN'),
|
||||||
|
act_cfg=dict(type='SiLU'),
|
||||||
|
init_cfg=dict(
|
||||||
|
type='Pretrained',
|
||||||
|
prefix='backbone.',
|
||||||
|
checkpoint='https://download.openmmlab.com/mmpose/v1/projects/'
|
||||||
|
'rtmpose/cspnext-l_udp-aic-coco_210e-256x192-273b7631_20230130.pth' # noqa: E501
|
||||||
|
)),
|
||||||
|
head=dict(
|
||||||
|
type='RTMCCHead',
|
||||||
|
in_channels=1024,
|
||||||
|
out_channels=133,
|
||||||
|
input_size=codec['input_size'],
|
||||||
|
in_featuremap_size=(9, 12),
|
||||||
|
simcc_split_ratio=codec['simcc_split_ratio'],
|
||||||
|
final_layer_kernel_size=7,
|
||||||
|
gau_cfg=dict(
|
||||||
|
hidden_dims=256,
|
||||||
|
s=128,
|
||||||
|
expansion_factor=2,
|
||||||
|
dropout_rate=0.,
|
||||||
|
drop_path=0.,
|
||||||
|
act_fn='SiLU',
|
||||||
|
use_rel_bias=False,
|
||||||
|
pos_enc=False),
|
||||||
|
loss=dict(
|
||||||
|
type='KLDiscretLoss',
|
||||||
|
use_target_weight=True,
|
||||||
|
beta=10.,
|
||||||
|
label_softmax=True),
|
||||||
|
decoder=codec),
|
||||||
|
test_cfg=dict(flip_test=True, ))
|
||||||
|
|
||||||
|
# base dataset settings
|
||||||
|
dataset_type = 'UBody2dDataset'
|
||||||
|
data_mode = 'topdown'
|
||||||
|
data_root = 'data/UBody/'
|
||||||
|
|
||||||
|
backend_args = dict(backend='local')
|
||||||
|
|
||||||
|
scenes = [
|
||||||
|
'Magic_show', 'Entertainment', 'ConductMusic', 'Online_class', 'TalkShow',
|
||||||
|
'Speech', 'Fitness', 'Interview', 'Olympic', 'TVShow', 'Singing',
|
||||||
|
'SignLanguage', 'Movie', 'LiveVlog', 'VideoConference'
|
||||||
|
]
|
||||||
|
|
||||||
|
train_datasets = [
|
||||||
|
dict(
|
||||||
|
type='CocoWholeBodyDataset',
|
||||||
|
data_root='data/coco/',
|
||||||
|
data_mode=data_mode,
|
||||||
|
ann_file='annotations/coco_wholebody_train_v1.0.json',
|
||||||
|
data_prefix=dict(img='train2017/'),
|
||||||
|
pipeline=[])
|
||||||
|
]
|
||||||
|
|
||||||
|
for scene in scenes:
|
||||||
|
train_dataset = dict(
|
||||||
|
type=dataset_type,
|
||||||
|
data_root=data_root,
|
||||||
|
data_mode=data_mode,
|
||||||
|
ann_file=f'annotations/{scene}/train_annotations.json',
|
||||||
|
data_prefix=dict(img='images/'),
|
||||||
|
pipeline=[],
|
||||||
|
sample_interval=10)
|
||||||
|
train_datasets.append(train_dataset)
|
||||||
|
|
||||||
|
# pipelines
|
||||||
|
train_pipeline = [
|
||||||
|
dict(type='LoadImage', backend_args=backend_args),
|
||||||
|
dict(type='GetBBoxCenterScale'),
|
||||||
|
dict(type='RandomFlip', direction='horizontal'),
|
||||||
|
dict(type='RandomHalfBody'),
|
||||||
|
dict(
|
||||||
|
type='RandomBBoxTransform', scale_factor=[0.5, 1.5], rotate_factor=90),
|
||||||
|
dict(type='TopdownAffine', input_size=codec['input_size']),
|
||||||
|
dict(type='mmdet.YOLOXHSVRandomAug'),
|
||||||
|
dict(
|
||||||
|
type='Albumentation',
|
||||||
|
transforms=[
|
||||||
|
dict(type='Blur', p=0.1),
|
||||||
|
dict(type='MedianBlur', p=0.1),
|
||||||
|
dict(
|
||||||
|
type='CoarseDropout',
|
||||||
|
max_holes=1,
|
||||||
|
max_height=0.4,
|
||||||
|
max_width=0.4,
|
||||||
|
min_holes=1,
|
||||||
|
min_height=0.2,
|
||||||
|
min_width=0.2,
|
||||||
|
p=1.0),
|
||||||
|
]),
|
||||||
|
dict(type='GenerateTarget', encoder=codec),
|
||||||
|
dict(type='PackPoseInputs')
|
||||||
|
]
|
||||||
|
val_pipeline = [
|
||||||
|
dict(type='LoadImage', backend_args=backend_args),
|
||||||
|
dict(type='GetBBoxCenterScale'),
|
||||||
|
dict(type='TopdownAffine', input_size=codec['input_size']),
|
||||||
|
dict(type='PackPoseInputs')
|
||||||
|
]
|
||||||
|
|
||||||
|
train_pipeline_stage2 = [
|
||||||
|
dict(type='LoadImage', backend_args=backend_args),
|
||||||
|
dict(type='GetBBoxCenterScale'),
|
||||||
|
dict(type='RandomFlip', direction='horizontal'),
|
||||||
|
dict(type='RandomHalfBody'),
|
||||||
|
dict(
|
||||||
|
type='RandomBBoxTransform',
|
||||||
|
shift_factor=0.,
|
||||||
|
scale_factor=[0.5, 1.5],
|
||||||
|
rotate_factor=90),
|
||||||
|
dict(type='TopdownAffine', input_size=codec['input_size']),
|
||||||
|
dict(type='mmdet.YOLOXHSVRandomAug'),
|
||||||
|
dict(
|
||||||
|
type='Albumentation',
|
||||||
|
transforms=[
|
||||||
|
dict(type='Blur', p=0.1),
|
||||||
|
dict(type='MedianBlur', p=0.1),
|
||||||
|
dict(
|
||||||
|
type='CoarseDropout',
|
||||||
|
max_holes=1,
|
||||||
|
max_height=0.4,
|
||||||
|
max_width=0.4,
|
||||||
|
min_holes=1,
|
||||||
|
min_height=0.2,
|
||||||
|
min_width=0.2,
|
||||||
|
p=0.5),
|
||||||
|
]),
|
||||||
|
dict(type='GenerateTarget', encoder=codec),
|
||||||
|
dict(type='PackPoseInputs')
|
||||||
|
]
|
||||||
|
|
||||||
|
# data loaders
|
||||||
|
train_dataloader = dict(
|
||||||
|
batch_size=train_batch_size,
|
||||||
|
num_workers=10,
|
||||||
|
persistent_workers=True,
|
||||||
|
sampler=dict(type='DefaultSampler', shuffle=True),
|
||||||
|
dataset=dict(
|
||||||
|
type='CombinedDataset',
|
||||||
|
metainfo=dict(from_file='configs/_base_/datasets/coco_wholebody.py'),
|
||||||
|
datasets=train_datasets,
|
||||||
|
pipeline=train_pipeline,
|
||||||
|
test_mode=False,
|
||||||
|
))
|
||||||
|
|
||||||
|
val_dataloader = dict(
|
||||||
|
batch_size=val_batch_size,
|
||||||
|
num_workers=10,
|
||||||
|
persistent_workers=True,
|
||||||
|
drop_last=False,
|
||||||
|
sampler=dict(type='DefaultSampler', shuffle=False, round_up=False),
|
||||||
|
dataset=dict(
|
||||||
|
type='CocoWholeBodyDataset',
|
||||||
|
data_root=data_root,
|
||||||
|
data_mode=data_mode,
|
||||||
|
ann_file='data/coco/annotations/coco_wholebody_val_v1.0.json',
|
||||||
|
bbox_file='data/coco/person_detection_results/'
|
||||||
|
'COCO_val2017_detections_AP_H_56_person.json',
|
||||||
|
data_prefix=dict(img='coco/val2017/'),
|
||||||
|
test_mode=True,
|
||||||
|
pipeline=val_pipeline,
|
||||||
|
))
|
||||||
|
test_dataloader = val_dataloader
|
||||||
|
|
||||||
|
# hooks
|
||||||
|
default_hooks = dict(
|
||||||
|
checkpoint=dict(
|
||||||
|
save_best='coco-wholebody/AP', rule='greater', max_keep_ckpts=1))
|
||||||
|
|
||||||
|
custom_hooks = [
|
||||||
|
dict(
|
||||||
|
type='EMAHook',
|
||||||
|
ema_type='ExpMomentumEMA',
|
||||||
|
momentum=0.0002,
|
||||||
|
update_buffers=True,
|
||||||
|
priority=49),
|
||||||
|
dict(
|
||||||
|
type='mmdet.PipelineSwitchHook',
|
||||||
|
switch_epoch=max_epochs - stage2_num_epochs,
|
||||||
|
switch_pipeline=train_pipeline_stage2)
|
||||||
|
]
|
||||||
|
|
||||||
|
# evaluators
|
||||||
|
val_evaluator = dict(
|
||||||
|
type='CocoWholeBodyMetric',
|
||||||
|
ann_file='data/coco/annotations/coco_wholebody_val_v1.0.json')
|
||||||
|
test_evaluator = val_evaluator
|
|
@ -0,0 +1 @@
|
||||||
|
The code for Face Detection in this folder has been taken from the wonderful [face_alignment](https://github.com/1adrianb/face-alignment) repository. This has been modified to take batches of faces at a time.
|
|
@ -0,0 +1,7 @@
|
||||||
|
# -*- coding: utf-8 -*-
|
||||||
|
|
||||||
|
__author__ = """Adrian Bulat"""
|
||||||
|
__email__ = 'adrian.bulat@nottingham.ac.uk'
|
||||||
|
__version__ = '1.0.1'
|
||||||
|
|
||||||
|
from .api import FaceAlignment, LandmarksType, NetworkSize, YOLOv8_face
|
|
@ -0,0 +1,240 @@
|
||||||
|
from __future__ import print_function
|
||||||
|
import os
|
||||||
|
import torch
|
||||||
|
from torch.utils.model_zoo import load_url
|
||||||
|
from enum import Enum
|
||||||
|
import numpy as np
|
||||||
|
import cv2
|
||||||
|
try:
|
||||||
|
import urllib.request as request_file
|
||||||
|
except BaseException:
|
||||||
|
import urllib as request_file
|
||||||
|
|
||||||
|
from .models import FAN, ResNetDepth
|
||||||
|
from .utils import *
|
||||||
|
|
||||||
|
|
||||||
|
class LandmarksType(Enum):
|
||||||
|
"""Enum class defining the type of landmarks to detect.
|
||||||
|
|
||||||
|
``_2D`` - the detected points ``(x,y)`` are detected in a 2D space and follow the visible contour of the face
|
||||||
|
``_2halfD`` - this points represent the projection of the 3D points into 3D
|
||||||
|
``_3D`` - detect the points ``(x,y,z)``` in a 3D space
|
||||||
|
|
||||||
|
"""
|
||||||
|
_2D = 1
|
||||||
|
_2halfD = 2
|
||||||
|
_3D = 3
|
||||||
|
|
||||||
|
|
||||||
|
class NetworkSize(Enum):
|
||||||
|
# TINY = 1
|
||||||
|
# SMALL = 2
|
||||||
|
# MEDIUM = 3
|
||||||
|
LARGE = 4
|
||||||
|
|
||||||
|
def __new__(cls, value):
|
||||||
|
member = object.__new__(cls)
|
||||||
|
member._value_ = value
|
||||||
|
return member
|
||||||
|
|
||||||
|
def __int__(self):
|
||||||
|
return self.value
|
||||||
|
|
||||||
|
|
||||||
|
|
||||||
|
class FaceAlignment:
|
||||||
|
def __init__(self, landmarks_type, network_size=NetworkSize.LARGE,
|
||||||
|
device='cuda', flip_input=False, face_detector='sfd', verbose=False):
|
||||||
|
self.device = device
|
||||||
|
self.flip_input = flip_input
|
||||||
|
self.landmarks_type = landmarks_type
|
||||||
|
self.verbose = verbose
|
||||||
|
|
||||||
|
network_size = int(network_size)
|
||||||
|
|
||||||
|
if 'cuda' in device:
|
||||||
|
torch.backends.cudnn.benchmark = True
|
||||||
|
# torch.backends.cuda.matmul.allow_tf32 = False
|
||||||
|
# torch.backends.cudnn.benchmark = True
|
||||||
|
# torch.backends.cudnn.deterministic = False
|
||||||
|
# torch.backends.cudnn.allow_tf32 = True
|
||||||
|
print('cuda start')
|
||||||
|
|
||||||
|
|
||||||
|
# Get the face detector
|
||||||
|
face_detector_module = __import__('face_detection.detection.' + face_detector,
|
||||||
|
globals(), locals(), [face_detector], 0)
|
||||||
|
|
||||||
|
self.face_detector = face_detector_module.FaceDetector(device=device, verbose=verbose)
|
||||||
|
|
||||||
|
def get_detections_for_batch(self, images):
|
||||||
|
images = images[..., ::-1]
|
||||||
|
detected_faces = self.face_detector.detect_from_batch(images.copy())
|
||||||
|
results = []
|
||||||
|
|
||||||
|
for i, d in enumerate(detected_faces):
|
||||||
|
if len(d) == 0:
|
||||||
|
results.append(None)
|
||||||
|
continue
|
||||||
|
d = d[0]
|
||||||
|
d = np.clip(d, 0, None)
|
||||||
|
|
||||||
|
x1, y1, x2, y2 = map(int, d[:-1])
|
||||||
|
results.append((x1, y1, x2, y2))
|
||||||
|
|
||||||
|
return results
|
||||||
|
|
||||||
|
|
||||||
|
class YOLOv8_face:
|
||||||
|
def __init__(self, path = 'face_detection/weights/yolov8n-face.onnx', conf_thres=0.2, iou_thres=0.5):
|
||||||
|
self.conf_threshold = conf_thres
|
||||||
|
self.iou_threshold = iou_thres
|
||||||
|
self.class_names = ['face']
|
||||||
|
self.num_classes = len(self.class_names)
|
||||||
|
# Initialize model
|
||||||
|
self.net = cv2.dnn.readNet(path)
|
||||||
|
self.input_height = 640
|
||||||
|
self.input_width = 640
|
||||||
|
self.reg_max = 16
|
||||||
|
|
||||||
|
self.project = np.arange(self.reg_max)
|
||||||
|
self.strides = (8, 16, 32)
|
||||||
|
self.feats_hw = [(math.ceil(self.input_height / self.strides[i]), math.ceil(self.input_width / self.strides[i])) for i in range(len(self.strides))]
|
||||||
|
self.anchors = self.make_anchors(self.feats_hw)
|
||||||
|
|
||||||
|
def make_anchors(self, feats_hw, grid_cell_offset=0.5):
|
||||||
|
"""Generate anchors from features."""
|
||||||
|
anchor_points = {}
|
||||||
|
for i, stride in enumerate(self.strides):
|
||||||
|
h,w = feats_hw[i]
|
||||||
|
x = np.arange(0, w) + grid_cell_offset # shift x
|
||||||
|
y = np.arange(0, h) + grid_cell_offset # shift y
|
||||||
|
sx, sy = np.meshgrid(x, y)
|
||||||
|
# sy, sx = np.meshgrid(y, x)
|
||||||
|
anchor_points[stride] = np.stack((sx, sy), axis=-1).reshape(-1, 2)
|
||||||
|
return anchor_points
|
||||||
|
|
||||||
|
def softmax(self, x, axis=1):
|
||||||
|
x_exp = np.exp(x)
|
||||||
|
# 如果是列向量,则axis=0
|
||||||
|
x_sum = np.sum(x_exp, axis=axis, keepdims=True)
|
||||||
|
s = x_exp / x_sum
|
||||||
|
return s
|
||||||
|
|
||||||
|
def resize_image(self, srcimg, keep_ratio=True):
|
||||||
|
top, left, newh, neww = 0, 0, self.input_width, self.input_height
|
||||||
|
if keep_ratio and srcimg.shape[0] != srcimg.shape[1]:
|
||||||
|
hw_scale = srcimg.shape[0] / srcimg.shape[1]
|
||||||
|
if hw_scale > 1:
|
||||||
|
newh, neww = self.input_height, int(self.input_width / hw_scale)
|
||||||
|
img = cv2.resize(srcimg, (neww, newh), interpolation=cv2.INTER_AREA)
|
||||||
|
left = int((self.input_width - neww) * 0.5)
|
||||||
|
img = cv2.copyMakeBorder(img, 0, 0, left, self.input_width - neww - left, cv2.BORDER_CONSTANT,
|
||||||
|
value=(0, 0, 0)) # add border
|
||||||
|
else:
|
||||||
|
newh, neww = int(self.input_height * hw_scale), self.input_width
|
||||||
|
img = cv2.resize(srcimg, (neww, newh), interpolation=cv2.INTER_AREA)
|
||||||
|
top = int((self.input_height - newh) * 0.5)
|
||||||
|
img = cv2.copyMakeBorder(img, top, self.input_height - newh - top, 0, 0, cv2.BORDER_CONSTANT,
|
||||||
|
value=(0, 0, 0))
|
||||||
|
else:
|
||||||
|
img = cv2.resize(srcimg, (self.input_width, self.input_height), interpolation=cv2.INTER_AREA)
|
||||||
|
return img, newh, neww, top, left
|
||||||
|
|
||||||
|
def detect(self, srcimg):
|
||||||
|
input_img, newh, neww, padh, padw = self.resize_image(cv2.cvtColor(srcimg, cv2.COLOR_BGR2RGB))
|
||||||
|
scale_h, scale_w = srcimg.shape[0]/newh, srcimg.shape[1]/neww
|
||||||
|
input_img = input_img.astype(np.float32) / 255.0
|
||||||
|
|
||||||
|
blob = cv2.dnn.blobFromImage(input_img)
|
||||||
|
self.net.setInput(blob)
|
||||||
|
outputs = self.net.forward(self.net.getUnconnectedOutLayersNames())
|
||||||
|
# if isinstance(outputs, tuple):
|
||||||
|
# outputs = list(outputs)
|
||||||
|
# if float(cv2.__version__[:3])>=4.7:
|
||||||
|
# outputs = [outputs[2], outputs[0], outputs[1]] ###opencv4.7需要这一步,opencv4.5不需要
|
||||||
|
# Perform inference on the image
|
||||||
|
det_bboxes, det_conf, det_classid, landmarks = self.post_process(outputs, scale_h, scale_w, padh, padw)
|
||||||
|
return det_bboxes, det_conf, det_classid, landmarks
|
||||||
|
|
||||||
|
def post_process(self, preds, scale_h, scale_w, padh, padw):
|
||||||
|
bboxes, scores, landmarks = [], [], []
|
||||||
|
for i, pred in enumerate(preds):
|
||||||
|
stride = int(self.input_height/pred.shape[2])
|
||||||
|
pred = pred.transpose((0, 2, 3, 1))
|
||||||
|
|
||||||
|
box = pred[..., :self.reg_max * 4]
|
||||||
|
cls = 1 / (1 + np.exp(-pred[..., self.reg_max * 4:-15])).reshape((-1,1))
|
||||||
|
kpts = pred[..., -15:].reshape((-1,15)) ### x1,y1,score1, ..., x5,y5,score5
|
||||||
|
|
||||||
|
# tmp = box.reshape(self.feats_hw[i][0], self.feats_hw[i][1], 4, self.reg_max)
|
||||||
|
tmp = box.reshape(-1, 4, self.reg_max)
|
||||||
|
bbox_pred = self.softmax(tmp, axis=-1)
|
||||||
|
bbox_pred = np.dot(bbox_pred, self.project).reshape((-1,4))
|
||||||
|
|
||||||
|
bbox = self.distance2bbox(self.anchors[stride], bbox_pred, max_shape=(self.input_height, self.input_width)) * stride
|
||||||
|
kpts[:, 0::3] = (kpts[:, 0::3] * 2.0 + (self.anchors[stride][:, 0].reshape((-1,1)) - 0.5)) * stride
|
||||||
|
kpts[:, 1::3] = (kpts[:, 1::3] * 2.0 + (self.anchors[stride][:, 1].reshape((-1,1)) - 0.5)) * stride
|
||||||
|
kpts[:, 2::3] = 1 / (1+np.exp(-kpts[:, 2::3]))
|
||||||
|
|
||||||
|
bbox -= np.array([[padw, padh, padw, padh]]) ###合理使用广播法则
|
||||||
|
bbox *= np.array([[scale_w, scale_h, scale_w, scale_h]])
|
||||||
|
kpts -= np.tile(np.array([padw, padh, 0]), 5).reshape((1,15))
|
||||||
|
kpts *= np.tile(np.array([scale_w, scale_h, 1]), 5).reshape((1,15))
|
||||||
|
|
||||||
|
bboxes.append(bbox)
|
||||||
|
scores.append(cls)
|
||||||
|
landmarks.append(kpts)
|
||||||
|
|
||||||
|
bboxes = np.concatenate(bboxes, axis=0)
|
||||||
|
scores = np.concatenate(scores, axis=0)
|
||||||
|
landmarks = np.concatenate(landmarks, axis=0)
|
||||||
|
|
||||||
|
bboxes_wh = bboxes.copy()
|
||||||
|
bboxes_wh[:, 2:4] = bboxes[:, 2:4] - bboxes[:, 0:2] ####xywh
|
||||||
|
classIds = np.argmax(scores, axis=1)
|
||||||
|
confidences = np.max(scores, axis=1) ####max_class_confidence
|
||||||
|
|
||||||
|
mask = confidences>self.conf_threshold
|
||||||
|
bboxes_wh = bboxes_wh[mask] ###合理使用广播法则
|
||||||
|
confidences = confidences[mask]
|
||||||
|
classIds = classIds[mask]
|
||||||
|
landmarks = landmarks[mask]
|
||||||
|
|
||||||
|
indices = cv2.dnn.NMSBoxes(bboxes_wh.tolist(), confidences.tolist(), self.conf_threshold,
|
||||||
|
self.iou_threshold).flatten()
|
||||||
|
if len(indices) > 0:
|
||||||
|
mlvl_bboxes = bboxes_wh[indices]
|
||||||
|
confidences = confidences[indices]
|
||||||
|
classIds = classIds[indices]
|
||||||
|
landmarks = landmarks[indices]
|
||||||
|
return mlvl_bboxes, confidences, classIds, landmarks
|
||||||
|
else:
|
||||||
|
print('nothing detect')
|
||||||
|
return np.array([]), np.array([]), np.array([]), np.array([])
|
||||||
|
|
||||||
|
def distance2bbox(self, points, distance, max_shape=None):
|
||||||
|
x1 = points[:, 0] - distance[:, 0]
|
||||||
|
y1 = points[:, 1] - distance[:, 1]
|
||||||
|
x2 = points[:, 0] + distance[:, 2]
|
||||||
|
y2 = points[:, 1] + distance[:, 3]
|
||||||
|
if max_shape is not None:
|
||||||
|
x1 = np.clip(x1, 0, max_shape[1])
|
||||||
|
y1 = np.clip(y1, 0, max_shape[0])
|
||||||
|
x2 = np.clip(x2, 0, max_shape[1])
|
||||||
|
y2 = np.clip(y2, 0, max_shape[0])
|
||||||
|
return np.stack([x1, y1, x2, y2], axis=-1)
|
||||||
|
|
||||||
|
def draw_detections(self, image, boxes, scores, kpts):
|
||||||
|
for box, score, kp in zip(boxes, scores, kpts):
|
||||||
|
x, y, w, h = box.astype(int)
|
||||||
|
# Draw rectangle
|
||||||
|
cv2.rectangle(image, (x, y), (x + w, y + h), (0, 0, 255), thickness=3)
|
||||||
|
cv2.putText(image, "face:"+str(round(score,2)), (x, y - 5), cv2.FONT_HERSHEY_SIMPLEX, 1, (0, 0, 255), thickness=2)
|
||||||
|
for i in range(5):
|
||||||
|
cv2.circle(image, (int(kp[i * 3]), int(kp[i * 3 + 1])), 4, (0, 255, 0), thickness=-1)
|
||||||
|
# cv2.putText(image, str(i), (int(kp[i * 3]), int(kp[i * 3 + 1]) - 10), cv2.FONT_HERSHEY_SIMPLEX, 1, (255, 0, 0), thickness=1)
|
||||||
|
return image
|
||||||
|
|
||||||
|
ROOT = os.path.dirname(os.path.abspath(__file__))
|
|
@ -0,0 +1 @@
|
||||||
|
from .core import FaceDetector
|
|
@ -0,0 +1,130 @@
|
||||||
|
import logging
|
||||||
|
import glob
|
||||||
|
from tqdm import tqdm
|
||||||
|
import numpy as np
|
||||||
|
import torch
|
||||||
|
import cv2
|
||||||
|
|
||||||
|
|
||||||
|
class FaceDetector(object):
|
||||||
|
"""An abstract class representing a face detector.
|
||||||
|
|
||||||
|
Any other face detection implementation must subclass it. All subclasses
|
||||||
|
must implement ``detect_from_image``, that return a list of detected
|
||||||
|
bounding boxes. Optionally, for speed considerations detect from path is
|
||||||
|
recommended.
|
||||||
|
"""
|
||||||
|
|
||||||
|
def __init__(self, device, verbose):
|
||||||
|
self.device = device
|
||||||
|
self.verbose = verbose
|
||||||
|
|
||||||
|
if verbose:
|
||||||
|
if 'cpu' in device:
|
||||||
|
logger = logging.getLogger(__name__)
|
||||||
|
logger.warning("Detection running on CPU, this may be potentially slow.")
|
||||||
|
|
||||||
|
if 'cpu' not in device and 'cuda' not in device:
|
||||||
|
if verbose:
|
||||||
|
logger.error("Expected values for device are: {cpu, cuda} but got: %s", device)
|
||||||
|
raise ValueError
|
||||||
|
|
||||||
|
def detect_from_image(self, tensor_or_path):
|
||||||
|
"""Detects faces in a given image.
|
||||||
|
|
||||||
|
This function detects the faces present in a provided BGR(usually)
|
||||||
|
image. The input can be either the image itself or the path to it.
|
||||||
|
|
||||||
|
Arguments:
|
||||||
|
tensor_or_path {numpy.ndarray, torch.tensor or string} -- the path
|
||||||
|
to an image or the image itself.
|
||||||
|
|
||||||
|
Example::
|
||||||
|
|
||||||
|
>>> path_to_image = 'data/image_01.jpg'
|
||||||
|
... detected_faces = detect_from_image(path_to_image)
|
||||||
|
[A list of bounding boxes (x1, y1, x2, y2)]
|
||||||
|
>>> image = cv2.imread(path_to_image)
|
||||||
|
... detected_faces = detect_from_image(image)
|
||||||
|
[A list of bounding boxes (x1, y1, x2, y2)]
|
||||||
|
|
||||||
|
"""
|
||||||
|
raise NotImplementedError
|
||||||
|
|
||||||
|
def detect_from_directory(self, path, extensions=['.jpg', '.png'], recursive=False, show_progress_bar=True):
|
||||||
|
"""Detects faces from all the images present in a given directory.
|
||||||
|
|
||||||
|
Arguments:
|
||||||
|
path {string} -- a string containing a path that points to the folder containing the images
|
||||||
|
|
||||||
|
Keyword Arguments:
|
||||||
|
extensions {list} -- list of string containing the extensions to be
|
||||||
|
consider in the following format: ``.extension_name`` (default:
|
||||||
|
{['.jpg', '.png']}) recursive {bool} -- option wherever to scan the
|
||||||
|
folder recursively (default: {False}) show_progress_bar {bool} --
|
||||||
|
display a progressbar (default: {True})
|
||||||
|
|
||||||
|
Example:
|
||||||
|
>>> directory = 'data'
|
||||||
|
... detected_faces = detect_from_directory(directory)
|
||||||
|
{A dictionary of [lists containing bounding boxes(x1, y1, x2, y2)]}
|
||||||
|
|
||||||
|
"""
|
||||||
|
if self.verbose:
|
||||||
|
logger = logging.getLogger(__name__)
|
||||||
|
|
||||||
|
if len(extensions) == 0:
|
||||||
|
if self.verbose:
|
||||||
|
logger.error("Expected at list one extension, but none was received.")
|
||||||
|
raise ValueError
|
||||||
|
|
||||||
|
if self.verbose:
|
||||||
|
logger.info("Constructing the list of images.")
|
||||||
|
additional_pattern = '/**/*' if recursive else '/*'
|
||||||
|
files = []
|
||||||
|
for extension in extensions:
|
||||||
|
files.extend(glob.glob(path + additional_pattern + extension, recursive=recursive))
|
||||||
|
|
||||||
|
if self.verbose:
|
||||||
|
logger.info("Finished searching for images. %s images found", len(files))
|
||||||
|
logger.info("Preparing to run the detection.")
|
||||||
|
|
||||||
|
predictions = {}
|
||||||
|
for image_path in tqdm(files, disable=not show_progress_bar):
|
||||||
|
if self.verbose:
|
||||||
|
logger.info("Running the face detector on image: %s", image_path)
|
||||||
|
predictions[image_path] = self.detect_from_image(image_path)
|
||||||
|
|
||||||
|
if self.verbose:
|
||||||
|
logger.info("The detector was successfully run on all %s images", len(files))
|
||||||
|
|
||||||
|
return predictions
|
||||||
|
|
||||||
|
@property
|
||||||
|
def reference_scale(self):
|
||||||
|
raise NotImplementedError
|
||||||
|
|
||||||
|
@property
|
||||||
|
def reference_x_shift(self):
|
||||||
|
raise NotImplementedError
|
||||||
|
|
||||||
|
@property
|
||||||
|
def reference_y_shift(self):
|
||||||
|
raise NotImplementedError
|
||||||
|
|
||||||
|
@staticmethod
|
||||||
|
def tensor_or_path_to_ndarray(tensor_or_path, rgb=True):
|
||||||
|
"""Convert path (represented as a string) or torch.tensor to a numpy.ndarray
|
||||||
|
|
||||||
|
Arguments:
|
||||||
|
tensor_or_path {numpy.ndarray, torch.tensor or string} -- path to the image, or the image itself
|
||||||
|
"""
|
||||||
|
if isinstance(tensor_or_path, str):
|
||||||
|
return cv2.imread(tensor_or_path) if not rgb else cv2.imread(tensor_or_path)[..., ::-1]
|
||||||
|
elif torch.is_tensor(tensor_or_path):
|
||||||
|
# Call cpu in case its coming from cuda
|
||||||
|
return tensor_or_path.cpu().numpy()[..., ::-1].copy() if not rgb else tensor_or_path.cpu().numpy()
|
||||||
|
elif isinstance(tensor_or_path, np.ndarray):
|
||||||
|
return tensor_or_path[..., ::-1].copy() if not rgb else tensor_or_path
|
||||||
|
else:
|
||||||
|
raise TypeError
|
|
@ -0,0 +1 @@
|
||||||
|
from .sfd_detector import SFDDetector as FaceDetector
|
|
@ -0,0 +1,129 @@
|
||||||
|
from __future__ import print_function
|
||||||
|
import os
|
||||||
|
import sys
|
||||||
|
import cv2
|
||||||
|
import random
|
||||||
|
import datetime
|
||||||
|
import time
|
||||||
|
import math
|
||||||
|
import argparse
|
||||||
|
import numpy as np
|
||||||
|
import torch
|
||||||
|
|
||||||
|
try:
|
||||||
|
from iou import IOU
|
||||||
|
except BaseException:
|
||||||
|
# IOU cython speedup 10x
|
||||||
|
def IOU(ax1, ay1, ax2, ay2, bx1, by1, bx2, by2):
|
||||||
|
sa = abs((ax2 - ax1) * (ay2 - ay1))
|
||||||
|
sb = abs((bx2 - bx1) * (by2 - by1))
|
||||||
|
x1, y1 = max(ax1, bx1), max(ay1, by1)
|
||||||
|
x2, y2 = min(ax2, bx2), min(ay2, by2)
|
||||||
|
w = x2 - x1
|
||||||
|
h = y2 - y1
|
||||||
|
if w < 0 or h < 0:
|
||||||
|
return 0.0
|
||||||
|
else:
|
||||||
|
return 1.0 * w * h / (sa + sb - w * h)
|
||||||
|
|
||||||
|
|
||||||
|
def bboxlog(x1, y1, x2, y2, axc, ayc, aww, ahh):
|
||||||
|
xc, yc, ww, hh = (x2 + x1) / 2, (y2 + y1) / 2, x2 - x1, y2 - y1
|
||||||
|
dx, dy = (xc - axc) / aww, (yc - ayc) / ahh
|
||||||
|
dw, dh = math.log(ww / aww), math.log(hh / ahh)
|
||||||
|
return dx, dy, dw, dh
|
||||||
|
|
||||||
|
|
||||||
|
def bboxloginv(dx, dy, dw, dh, axc, ayc, aww, ahh):
|
||||||
|
xc, yc = dx * aww + axc, dy * ahh + ayc
|
||||||
|
ww, hh = math.exp(dw) * aww, math.exp(dh) * ahh
|
||||||
|
x1, x2, y1, y2 = xc - ww / 2, xc + ww / 2, yc - hh / 2, yc + hh / 2
|
||||||
|
return x1, y1, x2, y2
|
||||||
|
|
||||||
|
|
||||||
|
def nms(dets, thresh):
|
||||||
|
if 0 == len(dets):
|
||||||
|
return []
|
||||||
|
x1, y1, x2, y2, scores = dets[:, 0], dets[:, 1], dets[:, 2], dets[:, 3], dets[:, 4]
|
||||||
|
areas = (x2 - x1 + 1) * (y2 - y1 + 1)
|
||||||
|
order = scores.argsort()[::-1]
|
||||||
|
|
||||||
|
keep = []
|
||||||
|
while order.size > 0:
|
||||||
|
i = order[0]
|
||||||
|
keep.append(i)
|
||||||
|
xx1, yy1 = np.maximum(x1[i], x1[order[1:]]), np.maximum(y1[i], y1[order[1:]])
|
||||||
|
xx2, yy2 = np.minimum(x2[i], x2[order[1:]]), np.minimum(y2[i], y2[order[1:]])
|
||||||
|
|
||||||
|
w, h = np.maximum(0.0, xx2 - xx1 + 1), np.maximum(0.0, yy2 - yy1 + 1)
|
||||||
|
ovr = w * h / (areas[i] + areas[order[1:]] - w * h)
|
||||||
|
|
||||||
|
inds = np.where(ovr <= thresh)[0]
|
||||||
|
order = order[inds + 1]
|
||||||
|
|
||||||
|
return keep
|
||||||
|
|
||||||
|
|
||||||
|
def encode(matched, priors, variances):
|
||||||
|
"""Encode the variances from the priorbox layers into the ground truth boxes
|
||||||
|
we have matched (based on jaccard overlap) with the prior boxes.
|
||||||
|
Args:
|
||||||
|
matched: (tensor) Coords of ground truth for each prior in point-form
|
||||||
|
Shape: [num_priors, 4].
|
||||||
|
priors: (tensor) Prior boxes in center-offset form
|
||||||
|
Shape: [num_priors,4].
|
||||||
|
variances: (list[float]) Variances of priorboxes
|
||||||
|
Return:
|
||||||
|
encoded boxes (tensor), Shape: [num_priors, 4]
|
||||||
|
"""
|
||||||
|
|
||||||
|
# dist b/t match center and prior's center
|
||||||
|
g_cxcy = (matched[:, :2] + matched[:, 2:]) / 2 - priors[:, :2]
|
||||||
|
# encode variance
|
||||||
|
g_cxcy /= (variances[0] * priors[:, 2:])
|
||||||
|
# match wh / prior wh
|
||||||
|
g_wh = (matched[:, 2:] - matched[:, :2]) / priors[:, 2:]
|
||||||
|
g_wh = torch.log(g_wh) / variances[1]
|
||||||
|
# return target for smooth_l1_loss
|
||||||
|
return torch.cat([g_cxcy, g_wh], 1) # [num_priors,4]
|
||||||
|
|
||||||
|
|
||||||
|
def decode(loc, priors, variances):
|
||||||
|
"""Decode locations from predictions using priors to undo
|
||||||
|
the encoding we did for offset regression at train time.
|
||||||
|
Args:
|
||||||
|
loc (tensor): location predictions for loc layers,
|
||||||
|
Shape: [num_priors,4]
|
||||||
|
priors (tensor): Prior boxes in center-offset form.
|
||||||
|
Shape: [num_priors,4].
|
||||||
|
variances: (list[float]) Variances of priorboxes
|
||||||
|
Return:
|
||||||
|
decoded bounding box predictions
|
||||||
|
"""
|
||||||
|
|
||||||
|
boxes = torch.cat((
|
||||||
|
priors[:, :2] + loc[:, :2] * variances[0] * priors[:, 2:],
|
||||||
|
priors[:, 2:] * torch.exp(loc[:, 2:] * variances[1])), 1)
|
||||||
|
boxes[:, :2] -= boxes[:, 2:] / 2
|
||||||
|
boxes[:, 2:] += boxes[:, :2]
|
||||||
|
return boxes
|
||||||
|
|
||||||
|
def batch_decode(loc, priors, variances):
|
||||||
|
"""Decode locations from predictions using priors to undo
|
||||||
|
the encoding we did for offset regression at train time.
|
||||||
|
Args:
|
||||||
|
loc (tensor): location predictions for loc layers,
|
||||||
|
Shape: [num_priors,4]
|
||||||
|
priors (tensor): Prior boxes in center-offset form.
|
||||||
|
Shape: [num_priors,4].
|
||||||
|
variances: (list[float]) Variances of priorboxes
|
||||||
|
Return:
|
||||||
|
decoded bounding box predictions
|
||||||
|
"""
|
||||||
|
|
||||||
|
boxes = torch.cat((
|
||||||
|
priors[:, :, :2] + loc[:, :, :2] * variances[0] * priors[:, :, 2:],
|
||||||
|
priors[:, :, 2:] * torch.exp(loc[:, :, 2:] * variances[1])), 2)
|
||||||
|
boxes[:, :, :2] -= boxes[:, :, 2:] / 2
|
||||||
|
boxes[:, :, 2:] += boxes[:, :, :2]
|
||||||
|
return boxes
|
|
@ -0,0 +1,114 @@
|
||||||
|
import torch
|
||||||
|
import torch.nn.functional as F
|
||||||
|
|
||||||
|
import os
|
||||||
|
import sys
|
||||||
|
import cv2
|
||||||
|
import random
|
||||||
|
import datetime
|
||||||
|
import math
|
||||||
|
import argparse
|
||||||
|
import numpy as np
|
||||||
|
|
||||||
|
import scipy.io as sio
|
||||||
|
import zipfile
|
||||||
|
from .net_s3fd import s3fd
|
||||||
|
from .bbox import *
|
||||||
|
|
||||||
|
|
||||||
|
def detect(net, img, device):
|
||||||
|
img = img - np.array([104, 117, 123])
|
||||||
|
img = img.transpose(2, 0, 1)
|
||||||
|
img = img.reshape((1,) + img.shape)
|
||||||
|
|
||||||
|
if 'cuda' in device:
|
||||||
|
torch.backends.cudnn.benchmark = True
|
||||||
|
|
||||||
|
img = torch.from_numpy(img).float().to(device)
|
||||||
|
BB, CC, HH, WW = img.size()
|
||||||
|
with torch.no_grad():
|
||||||
|
olist = net(img)
|
||||||
|
|
||||||
|
bboxlist = []
|
||||||
|
for i in range(len(olist) // 2):
|
||||||
|
olist[i * 2] = F.softmax(olist[i * 2], dim=1)
|
||||||
|
olist = [oelem.data.cpu() for oelem in olist]
|
||||||
|
for i in range(len(olist) // 2):
|
||||||
|
ocls, oreg = olist[i * 2], olist[i * 2 + 1]
|
||||||
|
FB, FC, FH, FW = ocls.size() # feature map size
|
||||||
|
stride = 2**(i + 2) # 4,8,16,32,64,128
|
||||||
|
anchor = stride * 4
|
||||||
|
poss = zip(*np.where(ocls[:, 1, :, :] > 0.05))
|
||||||
|
for Iindex, hindex, windex in poss:
|
||||||
|
axc, ayc = stride / 2 + windex * stride, stride / 2 + hindex * stride
|
||||||
|
score = ocls[0, 1, hindex, windex]
|
||||||
|
loc = oreg[0, :, hindex, windex].contiguous().view(1, 4)
|
||||||
|
priors = torch.Tensor([[axc / 1.0, ayc / 1.0, stride * 4 / 1.0, stride * 4 / 1.0]])
|
||||||
|
variances = [0.1, 0.2]
|
||||||
|
box = decode(loc, priors, variances)
|
||||||
|
x1, y1, x2, y2 = box[0] * 1.0
|
||||||
|
# cv2.rectangle(imgshow,(int(x1),int(y1)),(int(x2),int(y2)),(0,0,255),1)
|
||||||
|
bboxlist.append([x1, y1, x2, y2, score])
|
||||||
|
bboxlist = np.array(bboxlist)
|
||||||
|
if 0 == len(bboxlist):
|
||||||
|
bboxlist = np.zeros((1, 5))
|
||||||
|
|
||||||
|
return bboxlist
|
||||||
|
|
||||||
|
def batch_detect(net, imgs, device):
|
||||||
|
imgs = imgs - np.array([104, 117, 123])
|
||||||
|
imgs = imgs.transpose(0, 3, 1, 2)
|
||||||
|
|
||||||
|
if 'cuda' in device:
|
||||||
|
torch.backends.cudnn.benchmark = True
|
||||||
|
|
||||||
|
imgs = torch.from_numpy(imgs).float().to(device)
|
||||||
|
BB, CC, HH, WW = imgs.size()
|
||||||
|
with torch.no_grad():
|
||||||
|
olist = net(imgs)
|
||||||
|
# print(olist)
|
||||||
|
|
||||||
|
bboxlist = []
|
||||||
|
for i in range(len(olist) // 2):
|
||||||
|
olist[i * 2] = F.softmax(olist[i * 2], dim=1)
|
||||||
|
|
||||||
|
olist = [oelem.cpu() for oelem in olist]
|
||||||
|
for i in range(len(olist) // 2):
|
||||||
|
ocls, oreg = olist[i * 2], olist[i * 2 + 1]
|
||||||
|
FB, FC, FH, FW = ocls.size() # feature map size
|
||||||
|
stride = 2**(i + 2) # 4,8,16,32,64,128
|
||||||
|
anchor = stride * 4
|
||||||
|
poss = zip(*np.where(ocls[:, 1, :, :] > 0.05))
|
||||||
|
for Iindex, hindex, windex in poss:
|
||||||
|
axc, ayc = stride / 2 + windex * stride, stride / 2 + hindex * stride
|
||||||
|
score = ocls[:, 1, hindex, windex]
|
||||||
|
loc = oreg[:, :, hindex, windex].contiguous().view(BB, 1, 4)
|
||||||
|
priors = torch.Tensor([[axc / 1.0, ayc / 1.0, stride * 4 / 1.0, stride * 4 / 1.0]]).view(1, 1, 4)
|
||||||
|
variances = [0.1, 0.2]
|
||||||
|
box = batch_decode(loc, priors, variances)
|
||||||
|
box = box[:, 0] * 1.0
|
||||||
|
# cv2.rectangle(imgshow,(int(x1),int(y1)),(int(x2),int(y2)),(0,0,255),1)
|
||||||
|
bboxlist.append(torch.cat([box, score.unsqueeze(1)], 1).cpu().numpy())
|
||||||
|
bboxlist = np.array(bboxlist)
|
||||||
|
if 0 == len(bboxlist):
|
||||||
|
bboxlist = np.zeros((1, BB, 5))
|
||||||
|
|
||||||
|
return bboxlist
|
||||||
|
|
||||||
|
def flip_detect(net, img, device):
|
||||||
|
img = cv2.flip(img, 1)
|
||||||
|
b = detect(net, img, device)
|
||||||
|
|
||||||
|
bboxlist = np.zeros(b.shape)
|
||||||
|
bboxlist[:, 0] = img.shape[1] - b[:, 2]
|
||||||
|
bboxlist[:, 1] = b[:, 1]
|
||||||
|
bboxlist[:, 2] = img.shape[1] - b[:, 0]
|
||||||
|
bboxlist[:, 3] = b[:, 3]
|
||||||
|
bboxlist[:, 4] = b[:, 4]
|
||||||
|
return bboxlist
|
||||||
|
|
||||||
|
|
||||||
|
def pts_to_bb(pts):
|
||||||
|
min_x, min_y = np.min(pts, axis=0)
|
||||||
|
max_x, max_y = np.max(pts, axis=0)
|
||||||
|
return np.array([min_x, min_y, max_x, max_y])
|
|
@ -0,0 +1,129 @@
|
||||||
|
import torch
|
||||||
|
import torch.nn as nn
|
||||||
|
import torch.nn.functional as F
|
||||||
|
|
||||||
|
|
||||||
|
class L2Norm(nn.Module):
|
||||||
|
def __init__(self, n_channels, scale=1.0):
|
||||||
|
super(L2Norm, self).__init__()
|
||||||
|
self.n_channels = n_channels
|
||||||
|
self.scale = scale
|
||||||
|
self.eps = 1e-10
|
||||||
|
self.weight = nn.Parameter(torch.Tensor(self.n_channels))
|
||||||
|
self.weight.data *= 0.0
|
||||||
|
self.weight.data += self.scale
|
||||||
|
|
||||||
|
def forward(self, x):
|
||||||
|
norm = x.pow(2).sum(dim=1, keepdim=True).sqrt() + self.eps
|
||||||
|
x = x / norm * self.weight.view(1, -1, 1, 1)
|
||||||
|
return x
|
||||||
|
|
||||||
|
|
||||||
|
class s3fd(nn.Module):
|
||||||
|
def __init__(self):
|
||||||
|
super(s3fd, self).__init__()
|
||||||
|
self.conv1_1 = nn.Conv2d(3, 64, kernel_size=3, stride=1, padding=1)
|
||||||
|
self.conv1_2 = nn.Conv2d(64, 64, kernel_size=3, stride=1, padding=1)
|
||||||
|
|
||||||
|
self.conv2_1 = nn.Conv2d(64, 128, kernel_size=3, stride=1, padding=1)
|
||||||
|
self.conv2_2 = nn.Conv2d(128, 128, kernel_size=3, stride=1, padding=1)
|
||||||
|
|
||||||
|
self.conv3_1 = nn.Conv2d(128, 256, kernel_size=3, stride=1, padding=1)
|
||||||
|
self.conv3_2 = nn.Conv2d(256, 256, kernel_size=3, stride=1, padding=1)
|
||||||
|
self.conv3_3 = nn.Conv2d(256, 256, kernel_size=3, stride=1, padding=1)
|
||||||
|
|
||||||
|
self.conv4_1 = nn.Conv2d(256, 512, kernel_size=3, stride=1, padding=1)
|
||||||
|
self.conv4_2 = nn.Conv2d(512, 512, kernel_size=3, stride=1, padding=1)
|
||||||
|
self.conv4_3 = nn.Conv2d(512, 512, kernel_size=3, stride=1, padding=1)
|
||||||
|
|
||||||
|
self.conv5_1 = nn.Conv2d(512, 512, kernel_size=3, stride=1, padding=1)
|
||||||
|
self.conv5_2 = nn.Conv2d(512, 512, kernel_size=3, stride=1, padding=1)
|
||||||
|
self.conv5_3 = nn.Conv2d(512, 512, kernel_size=3, stride=1, padding=1)
|
||||||
|
|
||||||
|
self.fc6 = nn.Conv2d(512, 1024, kernel_size=3, stride=1, padding=3)
|
||||||
|
self.fc7 = nn.Conv2d(1024, 1024, kernel_size=1, stride=1, padding=0)
|
||||||
|
|
||||||
|
self.conv6_1 = nn.Conv2d(1024, 256, kernel_size=1, stride=1, padding=0)
|
||||||
|
self.conv6_2 = nn.Conv2d(256, 512, kernel_size=3, stride=2, padding=1)
|
||||||
|
|
||||||
|
self.conv7_1 = nn.Conv2d(512, 128, kernel_size=1, stride=1, padding=0)
|
||||||
|
self.conv7_2 = nn.Conv2d(128, 256, kernel_size=3, stride=2, padding=1)
|
||||||
|
|
||||||
|
self.conv3_3_norm = L2Norm(256, scale=10)
|
||||||
|
self.conv4_3_norm = L2Norm(512, scale=8)
|
||||||
|
self.conv5_3_norm = L2Norm(512, scale=5)
|
||||||
|
|
||||||
|
self.conv3_3_norm_mbox_conf = nn.Conv2d(256, 4, kernel_size=3, stride=1, padding=1)
|
||||||
|
self.conv3_3_norm_mbox_loc = nn.Conv2d(256, 4, kernel_size=3, stride=1, padding=1)
|
||||||
|
self.conv4_3_norm_mbox_conf = nn.Conv2d(512, 2, kernel_size=3, stride=1, padding=1)
|
||||||
|
self.conv4_3_norm_mbox_loc = nn.Conv2d(512, 4, kernel_size=3, stride=1, padding=1)
|
||||||
|
self.conv5_3_norm_mbox_conf = nn.Conv2d(512, 2, kernel_size=3, stride=1, padding=1)
|
||||||
|
self.conv5_3_norm_mbox_loc = nn.Conv2d(512, 4, kernel_size=3, stride=1, padding=1)
|
||||||
|
|
||||||
|
self.fc7_mbox_conf = nn.Conv2d(1024, 2, kernel_size=3, stride=1, padding=1)
|
||||||
|
self.fc7_mbox_loc = nn.Conv2d(1024, 4, kernel_size=3, stride=1, padding=1)
|
||||||
|
self.conv6_2_mbox_conf = nn.Conv2d(512, 2, kernel_size=3, stride=1, padding=1)
|
||||||
|
self.conv6_2_mbox_loc = nn.Conv2d(512, 4, kernel_size=3, stride=1, padding=1)
|
||||||
|
self.conv7_2_mbox_conf = nn.Conv2d(256, 2, kernel_size=3, stride=1, padding=1)
|
||||||
|
self.conv7_2_mbox_loc = nn.Conv2d(256, 4, kernel_size=3, stride=1, padding=1)
|
||||||
|
|
||||||
|
def forward(self, x):
|
||||||
|
h = F.relu(self.conv1_1(x))
|
||||||
|
h = F.relu(self.conv1_2(h))
|
||||||
|
h = F.max_pool2d(h, 2, 2)
|
||||||
|
|
||||||
|
h = F.relu(self.conv2_1(h))
|
||||||
|
h = F.relu(self.conv2_2(h))
|
||||||
|
h = F.max_pool2d(h, 2, 2)
|
||||||
|
|
||||||
|
h = F.relu(self.conv3_1(h))
|
||||||
|
h = F.relu(self.conv3_2(h))
|
||||||
|
h = F.relu(self.conv3_3(h))
|
||||||
|
f3_3 = h
|
||||||
|
h = F.max_pool2d(h, 2, 2)
|
||||||
|
|
||||||
|
h = F.relu(self.conv4_1(h))
|
||||||
|
h = F.relu(self.conv4_2(h))
|
||||||
|
h = F.relu(self.conv4_3(h))
|
||||||
|
f4_3 = h
|
||||||
|
h = F.max_pool2d(h, 2, 2)
|
||||||
|
|
||||||
|
h = F.relu(self.conv5_1(h))
|
||||||
|
h = F.relu(self.conv5_2(h))
|
||||||
|
h = F.relu(self.conv5_3(h))
|
||||||
|
f5_3 = h
|
||||||
|
h = F.max_pool2d(h, 2, 2)
|
||||||
|
|
||||||
|
h = F.relu(self.fc6(h))
|
||||||
|
h = F.relu(self.fc7(h))
|
||||||
|
ffc7 = h
|
||||||
|
h = F.relu(self.conv6_1(h))
|
||||||
|
h = F.relu(self.conv6_2(h))
|
||||||
|
f6_2 = h
|
||||||
|
h = F.relu(self.conv7_1(h))
|
||||||
|
h = F.relu(self.conv7_2(h))
|
||||||
|
f7_2 = h
|
||||||
|
|
||||||
|
f3_3 = self.conv3_3_norm(f3_3)
|
||||||
|
f4_3 = self.conv4_3_norm(f4_3)
|
||||||
|
f5_3 = self.conv5_3_norm(f5_3)
|
||||||
|
|
||||||
|
cls1 = self.conv3_3_norm_mbox_conf(f3_3)
|
||||||
|
reg1 = self.conv3_3_norm_mbox_loc(f3_3)
|
||||||
|
cls2 = self.conv4_3_norm_mbox_conf(f4_3)
|
||||||
|
reg2 = self.conv4_3_norm_mbox_loc(f4_3)
|
||||||
|
cls3 = self.conv5_3_norm_mbox_conf(f5_3)
|
||||||
|
reg3 = self.conv5_3_norm_mbox_loc(f5_3)
|
||||||
|
cls4 = self.fc7_mbox_conf(ffc7)
|
||||||
|
reg4 = self.fc7_mbox_loc(ffc7)
|
||||||
|
cls5 = self.conv6_2_mbox_conf(f6_2)
|
||||||
|
reg5 = self.conv6_2_mbox_loc(f6_2)
|
||||||
|
cls6 = self.conv7_2_mbox_conf(f7_2)
|
||||||
|
reg6 = self.conv7_2_mbox_loc(f7_2)
|
||||||
|
|
||||||
|
# max-out background label
|
||||||
|
chunk = torch.chunk(cls1, 4, 1)
|
||||||
|
bmax = torch.max(torch.max(chunk[0], chunk[1]), chunk[2])
|
||||||
|
cls1 = torch.cat([bmax, chunk[3]], dim=1)
|
||||||
|
|
||||||
|
return [cls1, reg1, cls2, reg2, cls3, reg3, cls4, reg4, cls5, reg5, cls6, reg6]
|
|
@ -0,0 +1,59 @@
|
||||||
|
import os
|
||||||
|
import cv2
|
||||||
|
from torch.utils.model_zoo import load_url
|
||||||
|
|
||||||
|
from ..core import FaceDetector
|
||||||
|
|
||||||
|
from .net_s3fd import s3fd
|
||||||
|
from .bbox import *
|
||||||
|
from .detect import *
|
||||||
|
|
||||||
|
models_urls = {
|
||||||
|
's3fd': 'https://www.adrianbulat.com/downloads/python-fan/s3fd-619a316812.pth',
|
||||||
|
}
|
||||||
|
|
||||||
|
|
||||||
|
class SFDDetector(FaceDetector):
|
||||||
|
def __init__(self, device, path_to_detector=os.path.join(os.path.dirname(os.path.abspath(__file__)), 's3fd.pth'), verbose=False):
|
||||||
|
super(SFDDetector, self).__init__(device, verbose)
|
||||||
|
|
||||||
|
# Initialise the face detector
|
||||||
|
if not os.path.isfile(path_to_detector):
|
||||||
|
model_weights = load_url(models_urls['s3fd'])
|
||||||
|
else:
|
||||||
|
model_weights = torch.load(path_to_detector)
|
||||||
|
|
||||||
|
self.face_detector = s3fd()
|
||||||
|
self.face_detector.load_state_dict(model_weights)
|
||||||
|
self.face_detector.to(device)
|
||||||
|
self.face_detector.eval()
|
||||||
|
|
||||||
|
def detect_from_image(self, tensor_or_path):
|
||||||
|
image = self.tensor_or_path_to_ndarray(tensor_or_path)
|
||||||
|
|
||||||
|
bboxlist = detect(self.face_detector, image, device=self.device)
|
||||||
|
keep = nms(bboxlist, 0.3)
|
||||||
|
bboxlist = bboxlist[keep, :]
|
||||||
|
bboxlist = [x for x in bboxlist if x[-1] > 0.5]
|
||||||
|
|
||||||
|
return bboxlist
|
||||||
|
|
||||||
|
def detect_from_batch(self, images):
|
||||||
|
bboxlists = batch_detect(self.face_detector, images, device=self.device)
|
||||||
|
keeps = [nms(bboxlists[:, i, :], 0.3) for i in range(bboxlists.shape[1])]
|
||||||
|
bboxlists = [bboxlists[keep, i, :] for i, keep in enumerate(keeps)]
|
||||||
|
bboxlists = [[x for x in bboxlist if x[-1] > 0.5] for bboxlist in bboxlists]
|
||||||
|
|
||||||
|
return bboxlists
|
||||||
|
|
||||||
|
@property
|
||||||
|
def reference_scale(self):
|
||||||
|
return 195
|
||||||
|
|
||||||
|
@property
|
||||||
|
def reference_x_shift(self):
|
||||||
|
return 0
|
||||||
|
|
||||||
|
@property
|
||||||
|
def reference_y_shift(self):
|
||||||
|
return 0
|
|
@ -0,0 +1,261 @@
|
||||||
|
import torch
|
||||||
|
import torch.nn as nn
|
||||||
|
import torch.nn.functional as F
|
||||||
|
import math
|
||||||
|
|
||||||
|
|
||||||
|
def conv3x3(in_planes, out_planes, strd=1, padding=1, bias=False):
|
||||||
|
"3x3 convolution with padding"
|
||||||
|
return nn.Conv2d(in_planes, out_planes, kernel_size=3,
|
||||||
|
stride=strd, padding=padding, bias=bias)
|
||||||
|
|
||||||
|
|
||||||
|
class ConvBlock(nn.Module):
|
||||||
|
def __init__(self, in_planes, out_planes):
|
||||||
|
super(ConvBlock, self).__init__()
|
||||||
|
self.bn1 = nn.BatchNorm2d(in_planes)
|
||||||
|
self.conv1 = conv3x3(in_planes, int(out_planes / 2))
|
||||||
|
self.bn2 = nn.BatchNorm2d(int(out_planes / 2))
|
||||||
|
self.conv2 = conv3x3(int(out_planes / 2), int(out_planes / 4))
|
||||||
|
self.bn3 = nn.BatchNorm2d(int(out_planes / 4))
|
||||||
|
self.conv3 = conv3x3(int(out_planes / 4), int(out_planes / 4))
|
||||||
|
|
||||||
|
if in_planes != out_planes:
|
||||||
|
self.downsample = nn.Sequential(
|
||||||
|
nn.BatchNorm2d(in_planes),
|
||||||
|
nn.ReLU(True),
|
||||||
|
nn.Conv2d(in_planes, out_planes,
|
||||||
|
kernel_size=1, stride=1, bias=False),
|
||||||
|
)
|
||||||
|
else:
|
||||||
|
self.downsample = None
|
||||||
|
|
||||||
|
def forward(self, x):
|
||||||
|
residual = x
|
||||||
|
|
||||||
|
out1 = self.bn1(x)
|
||||||
|
out1 = F.relu(out1, True)
|
||||||
|
out1 = self.conv1(out1)
|
||||||
|
|
||||||
|
out2 = self.bn2(out1)
|
||||||
|
out2 = F.relu(out2, True)
|
||||||
|
out2 = self.conv2(out2)
|
||||||
|
|
||||||
|
out3 = self.bn3(out2)
|
||||||
|
out3 = F.relu(out3, True)
|
||||||
|
out3 = self.conv3(out3)
|
||||||
|
|
||||||
|
out3 = torch.cat((out1, out2, out3), 1)
|
||||||
|
|
||||||
|
if self.downsample is not None:
|
||||||
|
residual = self.downsample(residual)
|
||||||
|
|
||||||
|
out3 += residual
|
||||||
|
|
||||||
|
return out3
|
||||||
|
|
||||||
|
|
||||||
|
class Bottleneck(nn.Module):
|
||||||
|
|
||||||
|
expansion = 4
|
||||||
|
|
||||||
|
def __init__(self, inplanes, planes, stride=1, downsample=None):
|
||||||
|
super(Bottleneck, self).__init__()
|
||||||
|
self.conv1 = nn.Conv2d(inplanes, planes, kernel_size=1, bias=False)
|
||||||
|
self.bn1 = nn.BatchNorm2d(planes)
|
||||||
|
self.conv2 = nn.Conv2d(planes, planes, kernel_size=3, stride=stride,
|
||||||
|
padding=1, bias=False)
|
||||||
|
self.bn2 = nn.BatchNorm2d(planes)
|
||||||
|
self.conv3 = nn.Conv2d(planes, planes * 4, kernel_size=1, bias=False)
|
||||||
|
self.bn3 = nn.BatchNorm2d(planes * 4)
|
||||||
|
self.relu = nn.ReLU(inplace=True)
|
||||||
|
self.downsample = downsample
|
||||||
|
self.stride = stride
|
||||||
|
|
||||||
|
def forward(self, x):
|
||||||
|
residual = x
|
||||||
|
|
||||||
|
out = self.conv1(x)
|
||||||
|
out = self.bn1(out)
|
||||||
|
out = self.relu(out)
|
||||||
|
|
||||||
|
out = self.conv2(out)
|
||||||
|
out = self.bn2(out)
|
||||||
|
out = self.relu(out)
|
||||||
|
|
||||||
|
out = self.conv3(out)
|
||||||
|
out = self.bn3(out)
|
||||||
|
|
||||||
|
if self.downsample is not None:
|
||||||
|
residual = self.downsample(x)
|
||||||
|
|
||||||
|
out += residual
|
||||||
|
out = self.relu(out)
|
||||||
|
|
||||||
|
return out
|
||||||
|
|
||||||
|
|
||||||
|
class HourGlass(nn.Module):
|
||||||
|
def __init__(self, num_modules, depth, num_features):
|
||||||
|
super(HourGlass, self).__init__()
|
||||||
|
self.num_modules = num_modules
|
||||||
|
self.depth = depth
|
||||||
|
self.features = num_features
|
||||||
|
|
||||||
|
self._generate_network(self.depth)
|
||||||
|
|
||||||
|
def _generate_network(self, level):
|
||||||
|
self.add_module('b1_' + str(level), ConvBlock(self.features, self.features))
|
||||||
|
|
||||||
|
self.add_module('b2_' + str(level), ConvBlock(self.features, self.features))
|
||||||
|
|
||||||
|
if level > 1:
|
||||||
|
self._generate_network(level - 1)
|
||||||
|
else:
|
||||||
|
self.add_module('b2_plus_' + str(level), ConvBlock(self.features, self.features))
|
||||||
|
|
||||||
|
self.add_module('b3_' + str(level), ConvBlock(self.features, self.features))
|
||||||
|
|
||||||
|
def _forward(self, level, inp):
|
||||||
|
# Upper branch
|
||||||
|
up1 = inp
|
||||||
|
up1 = self._modules['b1_' + str(level)](up1)
|
||||||
|
|
||||||
|
# Lower branch
|
||||||
|
low1 = F.avg_pool2d(inp, 2, stride=2)
|
||||||
|
low1 = self._modules['b2_' + str(level)](low1)
|
||||||
|
|
||||||
|
if level > 1:
|
||||||
|
low2 = self._forward(level - 1, low1)
|
||||||
|
else:
|
||||||
|
low2 = low1
|
||||||
|
low2 = self._modules['b2_plus_' + str(level)](low2)
|
||||||
|
|
||||||
|
low3 = low2
|
||||||
|
low3 = self._modules['b3_' + str(level)](low3)
|
||||||
|
|
||||||
|
up2 = F.interpolate(low3, scale_factor=2, mode='nearest')
|
||||||
|
|
||||||
|
return up1 + up2
|
||||||
|
|
||||||
|
def forward(self, x):
|
||||||
|
return self._forward(self.depth, x)
|
||||||
|
|
||||||
|
|
||||||
|
class FAN(nn.Module):
|
||||||
|
|
||||||
|
def __init__(self, num_modules=1):
|
||||||
|
super(FAN, self).__init__()
|
||||||
|
self.num_modules = num_modules
|
||||||
|
|
||||||
|
# Base part
|
||||||
|
self.conv1 = nn.Conv2d(3, 64, kernel_size=7, stride=2, padding=3)
|
||||||
|
self.bn1 = nn.BatchNorm2d(64)
|
||||||
|
self.conv2 = ConvBlock(64, 128)
|
||||||
|
self.conv3 = ConvBlock(128, 128)
|
||||||
|
self.conv4 = ConvBlock(128, 256)
|
||||||
|
|
||||||
|
# Stacking part
|
||||||
|
for hg_module in range(self.num_modules):
|
||||||
|
self.add_module('m' + str(hg_module), HourGlass(1, 4, 256))
|
||||||
|
self.add_module('top_m_' + str(hg_module), ConvBlock(256, 256))
|
||||||
|
self.add_module('conv_last' + str(hg_module),
|
||||||
|
nn.Conv2d(256, 256, kernel_size=1, stride=1, padding=0))
|
||||||
|
self.add_module('bn_end' + str(hg_module), nn.BatchNorm2d(256))
|
||||||
|
self.add_module('l' + str(hg_module), nn.Conv2d(256,
|
||||||
|
68, kernel_size=1, stride=1, padding=0))
|
||||||
|
|
||||||
|
if hg_module < self.num_modules - 1:
|
||||||
|
self.add_module(
|
||||||
|
'bl' + str(hg_module), nn.Conv2d(256, 256, kernel_size=1, stride=1, padding=0))
|
||||||
|
self.add_module('al' + str(hg_module), nn.Conv2d(68,
|
||||||
|
256, kernel_size=1, stride=1, padding=0))
|
||||||
|
|
||||||
|
def forward(self, x):
|
||||||
|
x = F.relu(self.bn1(self.conv1(x)), True)
|
||||||
|
x = F.avg_pool2d(self.conv2(x), 2, stride=2)
|
||||||
|
x = self.conv3(x)
|
||||||
|
x = self.conv4(x)
|
||||||
|
|
||||||
|
previous = x
|
||||||
|
|
||||||
|
outputs = []
|
||||||
|
for i in range(self.num_modules):
|
||||||
|
hg = self._modules['m' + str(i)](previous)
|
||||||
|
|
||||||
|
ll = hg
|
||||||
|
ll = self._modules['top_m_' + str(i)](ll)
|
||||||
|
|
||||||
|
ll = F.relu(self._modules['bn_end' + str(i)]
|
||||||
|
(self._modules['conv_last' + str(i)](ll)), True)
|
||||||
|
|
||||||
|
# Predict heatmaps
|
||||||
|
tmp_out = self._modules['l' + str(i)](ll)
|
||||||
|
outputs.append(tmp_out)
|
||||||
|
|
||||||
|
if i < self.num_modules - 1:
|
||||||
|
ll = self._modules['bl' + str(i)](ll)
|
||||||
|
tmp_out_ = self._modules['al' + str(i)](tmp_out)
|
||||||
|
previous = previous + ll + tmp_out_
|
||||||
|
|
||||||
|
return outputs
|
||||||
|
|
||||||
|
|
||||||
|
class ResNetDepth(nn.Module):
|
||||||
|
|
||||||
|
def __init__(self, block=Bottleneck, layers=[3, 8, 36, 3], num_classes=68):
|
||||||
|
self.inplanes = 64
|
||||||
|
super(ResNetDepth, self).__init__()
|
||||||
|
self.conv1 = nn.Conv2d(3 + 68, 64, kernel_size=7, stride=2, padding=3,
|
||||||
|
bias=False)
|
||||||
|
self.bn1 = nn.BatchNorm2d(64)
|
||||||
|
self.relu = nn.ReLU(inplace=True)
|
||||||
|
self.maxpool = nn.MaxPool2d(kernel_size=3, stride=2, padding=1)
|
||||||
|
self.layer1 = self._make_layer(block, 64, layers[0])
|
||||||
|
self.layer2 = self._make_layer(block, 128, layers[1], stride=2)
|
||||||
|
self.layer3 = self._make_layer(block, 256, layers[2], stride=2)
|
||||||
|
self.layer4 = self._make_layer(block, 512, layers[3], stride=2)
|
||||||
|
self.avgpool = nn.AvgPool2d(7)
|
||||||
|
self.fc = nn.Linear(512 * block.expansion, num_classes)
|
||||||
|
|
||||||
|
for m in self.modules():
|
||||||
|
if isinstance(m, nn.Conv2d):
|
||||||
|
n = m.kernel_size[0] * m.kernel_size[1] * m.out_channels
|
||||||
|
m.weight.data.normal_(0, math.sqrt(2. / n))
|
||||||
|
elif isinstance(m, nn.BatchNorm2d):
|
||||||
|
m.weight.data.fill_(1)
|
||||||
|
m.bias.data.zero_()
|
||||||
|
|
||||||
|
def _make_layer(self, block, planes, blocks, stride=1):
|
||||||
|
downsample = None
|
||||||
|
if stride != 1 or self.inplanes != planes * block.expansion:
|
||||||
|
downsample = nn.Sequential(
|
||||||
|
nn.Conv2d(self.inplanes, planes * block.expansion,
|
||||||
|
kernel_size=1, stride=stride, bias=False),
|
||||||
|
nn.BatchNorm2d(planes * block.expansion),
|
||||||
|
)
|
||||||
|
|
||||||
|
layers = []
|
||||||
|
layers.append(block(self.inplanes, planes, stride, downsample))
|
||||||
|
self.inplanes = planes * block.expansion
|
||||||
|
for i in range(1, blocks):
|
||||||
|
layers.append(block(self.inplanes, planes))
|
||||||
|
|
||||||
|
return nn.Sequential(*layers)
|
||||||
|
|
||||||
|
def forward(self, x):
|
||||||
|
x = self.conv1(x)
|
||||||
|
x = self.bn1(x)
|
||||||
|
x = self.relu(x)
|
||||||
|
x = self.maxpool(x)
|
||||||
|
|
||||||
|
x = self.layer1(x)
|
||||||
|
x = self.layer2(x)
|
||||||
|
x = self.layer3(x)
|
||||||
|
x = self.layer4(x)
|
||||||
|
|
||||||
|
x = self.avgpool(x)
|
||||||
|
x = x.view(x.size(0), -1)
|
||||||
|
x = self.fc(x)
|
||||||
|
|
||||||
|
return x
|
|
@ -0,0 +1,313 @@
|
||||||
|
from __future__ import print_function
|
||||||
|
import os
|
||||||
|
import sys
|
||||||
|
import time
|
||||||
|
import torch
|
||||||
|
import math
|
||||||
|
import numpy as np
|
||||||
|
import cv2
|
||||||
|
|
||||||
|
|
||||||
|
def _gaussian(
|
||||||
|
size=3, sigma=0.25, amplitude=1, normalize=False, width=None,
|
||||||
|
height=None, sigma_horz=None, sigma_vert=None, mean_horz=0.5,
|
||||||
|
mean_vert=0.5):
|
||||||
|
# handle some defaults
|
||||||
|
if width is None:
|
||||||
|
width = size
|
||||||
|
if height is None:
|
||||||
|
height = size
|
||||||
|
if sigma_horz is None:
|
||||||
|
sigma_horz = sigma
|
||||||
|
if sigma_vert is None:
|
||||||
|
sigma_vert = sigma
|
||||||
|
center_x = mean_horz * width + 0.5
|
||||||
|
center_y = mean_vert * height + 0.5
|
||||||
|
gauss = np.empty((height, width), dtype=np.float32)
|
||||||
|
# generate kernel
|
||||||
|
for i in range(height):
|
||||||
|
for j in range(width):
|
||||||
|
gauss[i][j] = amplitude * math.exp(-(math.pow((j + 1 - center_x) / (
|
||||||
|
sigma_horz * width), 2) / 2.0 + math.pow((i + 1 - center_y) / (sigma_vert * height), 2) / 2.0))
|
||||||
|
if normalize:
|
||||||
|
gauss = gauss / np.sum(gauss)
|
||||||
|
return gauss
|
||||||
|
|
||||||
|
|
||||||
|
def draw_gaussian(image, point, sigma):
|
||||||
|
# Check if the gaussian is inside
|
||||||
|
ul = [math.floor(point[0] - 3 * sigma), math.floor(point[1] - 3 * sigma)]
|
||||||
|
br = [math.floor(point[0] + 3 * sigma), math.floor(point[1] + 3 * sigma)]
|
||||||
|
if (ul[0] > image.shape[1] or ul[1] > image.shape[0] or br[0] < 1 or br[1] < 1):
|
||||||
|
return image
|
||||||
|
size = 6 * sigma + 1
|
||||||
|
g = _gaussian(size)
|
||||||
|
g_x = [int(max(1, -ul[0])), int(min(br[0], image.shape[1])) - int(max(1, ul[0])) + int(max(1, -ul[0]))]
|
||||||
|
g_y = [int(max(1, -ul[1])), int(min(br[1], image.shape[0])) - int(max(1, ul[1])) + int(max(1, -ul[1]))]
|
||||||
|
img_x = [int(max(1, ul[0])), int(min(br[0], image.shape[1]))]
|
||||||
|
img_y = [int(max(1, ul[1])), int(min(br[1], image.shape[0]))]
|
||||||
|
assert (g_x[0] > 0 and g_y[1] > 0)
|
||||||
|
image[img_y[0] - 1:img_y[1], img_x[0] - 1:img_x[1]
|
||||||
|
] = image[img_y[0] - 1:img_y[1], img_x[0] - 1:img_x[1]] + g[g_y[0] - 1:g_y[1], g_x[0] - 1:g_x[1]]
|
||||||
|
image[image > 1] = 1
|
||||||
|
return image
|
||||||
|
|
||||||
|
|
||||||
|
def transform(point, center, scale, resolution, invert=False):
|
||||||
|
"""Generate and affine transformation matrix.
|
||||||
|
|
||||||
|
Given a set of points, a center, a scale and a targer resolution, the
|
||||||
|
function generates and affine transformation matrix. If invert is ``True``
|
||||||
|
it will produce the inverse transformation.
|
||||||
|
|
||||||
|
Arguments:
|
||||||
|
point {torch.tensor} -- the input 2D point
|
||||||
|
center {torch.tensor or numpy.array} -- the center around which to perform the transformations
|
||||||
|
scale {float} -- the scale of the face/object
|
||||||
|
resolution {float} -- the output resolution
|
||||||
|
|
||||||
|
Keyword Arguments:
|
||||||
|
invert {bool} -- define wherever the function should produce the direct or the
|
||||||
|
inverse transformation matrix (default: {False})
|
||||||
|
"""
|
||||||
|
_pt = torch.ones(3)
|
||||||
|
_pt[0] = point[0]
|
||||||
|
_pt[1] = point[1]
|
||||||
|
|
||||||
|
h = 200.0 * scale
|
||||||
|
t = torch.eye(3)
|
||||||
|
t[0, 0] = resolution / h
|
||||||
|
t[1, 1] = resolution / h
|
||||||
|
t[0, 2] = resolution * (-center[0] / h + 0.5)
|
||||||
|
t[1, 2] = resolution * (-center[1] / h + 0.5)
|
||||||
|
|
||||||
|
if invert:
|
||||||
|
t = torch.inverse(t)
|
||||||
|
|
||||||
|
new_point = (torch.matmul(t, _pt))[0:2]
|
||||||
|
|
||||||
|
return new_point.int()
|
||||||
|
|
||||||
|
|
||||||
|
def crop(image, center, scale, resolution=256.0):
|
||||||
|
"""Center crops an image or set of heatmaps
|
||||||
|
|
||||||
|
Arguments:
|
||||||
|
image {numpy.array} -- an rgb image
|
||||||
|
center {numpy.array} -- the center of the object, usually the same as of the bounding box
|
||||||
|
scale {float} -- scale of the face
|
||||||
|
|
||||||
|
Keyword Arguments:
|
||||||
|
resolution {float} -- the size of the output cropped image (default: {256.0})
|
||||||
|
|
||||||
|
Returns:
|
||||||
|
[type] -- [description]
|
||||||
|
""" # Crop around the center point
|
||||||
|
""" Crops the image around the center. Input is expected to be an np.ndarray """
|
||||||
|
ul = transform([1, 1], center, scale, resolution, True)
|
||||||
|
br = transform([resolution, resolution], center, scale, resolution, True)
|
||||||
|
# pad = math.ceil(torch.norm((ul - br).float()) / 2.0 - (br[0] - ul[0]) / 2.0)
|
||||||
|
if image.ndim > 2:
|
||||||
|
newDim = np.array([br[1] - ul[1], br[0] - ul[0],
|
||||||
|
image.shape[2]], dtype=np.int32)
|
||||||
|
newImg = np.zeros(newDim, dtype=np.uint8)
|
||||||
|
else:
|
||||||
|
newDim = np.array([br[1] - ul[1], br[0] - ul[0]], dtype=np.int)
|
||||||
|
newImg = np.zeros(newDim, dtype=np.uint8)
|
||||||
|
ht = image.shape[0]
|
||||||
|
wd = image.shape[1]
|
||||||
|
newX = np.array(
|
||||||
|
[max(1, -ul[0] + 1), min(br[0], wd) - ul[0]], dtype=np.int32)
|
||||||
|
newY = np.array(
|
||||||
|
[max(1, -ul[1] + 1), min(br[1], ht) - ul[1]], dtype=np.int32)
|
||||||
|
oldX = np.array([max(1, ul[0] + 1), min(br[0], wd)], dtype=np.int32)
|
||||||
|
oldY = np.array([max(1, ul[1] + 1), min(br[1], ht)], dtype=np.int32)
|
||||||
|
newImg[newY[0] - 1:newY[1], newX[0] - 1:newX[1]
|
||||||
|
] = image[oldY[0] - 1:oldY[1], oldX[0] - 1:oldX[1], :]
|
||||||
|
newImg = cv2.resize(newImg, dsize=(int(resolution), int(resolution)),
|
||||||
|
interpolation=cv2.INTER_LINEAR)
|
||||||
|
return newImg
|
||||||
|
|
||||||
|
|
||||||
|
def get_preds_fromhm(hm, center=None, scale=None):
|
||||||
|
"""Obtain (x,y) coordinates given a set of N heatmaps. If the center
|
||||||
|
and the scale is provided the function will return the points also in
|
||||||
|
the original coordinate frame.
|
||||||
|
|
||||||
|
Arguments:
|
||||||
|
hm {torch.tensor} -- the predicted heatmaps, of shape [B, N, W, H]
|
||||||
|
|
||||||
|
Keyword Arguments:
|
||||||
|
center {torch.tensor} -- the center of the bounding box (default: {None})
|
||||||
|
scale {float} -- face scale (default: {None})
|
||||||
|
"""
|
||||||
|
max, idx = torch.max(
|
||||||
|
hm.view(hm.size(0), hm.size(1), hm.size(2) * hm.size(3)), 2)
|
||||||
|
idx += 1
|
||||||
|
preds = idx.view(idx.size(0), idx.size(1), 1).repeat(1, 1, 2).float()
|
||||||
|
preds[..., 0].apply_(lambda x: (x - 1) % hm.size(3) + 1)
|
||||||
|
preds[..., 1].add_(-1).div_(hm.size(2)).floor_().add_(1)
|
||||||
|
|
||||||
|
for i in range(preds.size(0)):
|
||||||
|
for j in range(preds.size(1)):
|
||||||
|
hm_ = hm[i, j, :]
|
||||||
|
pX, pY = int(preds[i, j, 0]) - 1, int(preds[i, j, 1]) - 1
|
||||||
|
if pX > 0 and pX < 63 and pY > 0 and pY < 63:
|
||||||
|
diff = torch.FloatTensor(
|
||||||
|
[hm_[pY, pX + 1] - hm_[pY, pX - 1],
|
||||||
|
hm_[pY + 1, pX] - hm_[pY - 1, pX]])
|
||||||
|
preds[i, j].add_(diff.sign_().mul_(.25))
|
||||||
|
|
||||||
|
preds.add_(-.5)
|
||||||
|
|
||||||
|
preds_orig = torch.zeros(preds.size())
|
||||||
|
if center is not None and scale is not None:
|
||||||
|
for i in range(hm.size(0)):
|
||||||
|
for j in range(hm.size(1)):
|
||||||
|
preds_orig[i, j] = transform(
|
||||||
|
preds[i, j], center, scale, hm.size(2), True)
|
||||||
|
|
||||||
|
return preds, preds_orig
|
||||||
|
|
||||||
|
def get_preds_fromhm_batch(hm, centers=None, scales=None):
|
||||||
|
"""Obtain (x,y) coordinates given a set of N heatmaps. If the centers
|
||||||
|
and the scales is provided the function will return the points also in
|
||||||
|
the original coordinate frame.
|
||||||
|
|
||||||
|
Arguments:
|
||||||
|
hm {torch.tensor} -- the predicted heatmaps, of shape [B, N, W, H]
|
||||||
|
|
||||||
|
Keyword Arguments:
|
||||||
|
centers {torch.tensor} -- the centers of the bounding box (default: {None})
|
||||||
|
scales {float} -- face scales (default: {None})
|
||||||
|
"""
|
||||||
|
max, idx = torch.max(
|
||||||
|
hm.view(hm.size(0), hm.size(1), hm.size(2) * hm.size(3)), 2)
|
||||||
|
idx += 1
|
||||||
|
preds = idx.view(idx.size(0), idx.size(1), 1).repeat(1, 1, 2).float()
|
||||||
|
preds[..., 0].apply_(lambda x: (x - 1) % hm.size(3) + 1)
|
||||||
|
preds[..., 1].add_(-1).div_(hm.size(2)).floor_().add_(1)
|
||||||
|
|
||||||
|
for i in range(preds.size(0)):
|
||||||
|
for j in range(preds.size(1)):
|
||||||
|
hm_ = hm[i, j, :]
|
||||||
|
pX, pY = int(preds[i, j, 0]) - 1, int(preds[i, j, 1]) - 1
|
||||||
|
if pX > 0 and pX < 63 and pY > 0 and pY < 63:
|
||||||
|
diff = torch.FloatTensor(
|
||||||
|
[hm_[pY, pX + 1] - hm_[pY, pX - 1],
|
||||||
|
hm_[pY + 1, pX] - hm_[pY - 1, pX]])
|
||||||
|
preds[i, j].add_(diff.sign_().mul_(.25))
|
||||||
|
|
||||||
|
preds.add_(-.5)
|
||||||
|
|
||||||
|
preds_orig = torch.zeros(preds.size())
|
||||||
|
if centers is not None and scales is not None:
|
||||||
|
for i in range(hm.size(0)):
|
||||||
|
for j in range(hm.size(1)):
|
||||||
|
preds_orig[i, j] = transform(
|
||||||
|
preds[i, j], centers[i], scales[i], hm.size(2), True)
|
||||||
|
|
||||||
|
return preds, preds_orig
|
||||||
|
|
||||||
|
def shuffle_lr(parts, pairs=None):
|
||||||
|
"""Shuffle the points left-right according to the axis of symmetry
|
||||||
|
of the object.
|
||||||
|
|
||||||
|
Arguments:
|
||||||
|
parts {torch.tensor} -- a 3D or 4D object containing the
|
||||||
|
heatmaps.
|
||||||
|
|
||||||
|
Keyword Arguments:
|
||||||
|
pairs {list of integers} -- [order of the flipped points] (default: {None})
|
||||||
|
"""
|
||||||
|
if pairs is None:
|
||||||
|
pairs = [16, 15, 14, 13, 12, 11, 10, 9, 8, 7, 6, 5, 4, 3, 2, 1, 0,
|
||||||
|
26, 25, 24, 23, 22, 21, 20, 19, 18, 17, 27, 28, 29, 30, 35,
|
||||||
|
34, 33, 32, 31, 45, 44, 43, 42, 47, 46, 39, 38, 37, 36, 41,
|
||||||
|
40, 54, 53, 52, 51, 50, 49, 48, 59, 58, 57, 56, 55, 64, 63,
|
||||||
|
62, 61, 60, 67, 66, 65]
|
||||||
|
if parts.ndimension() == 3:
|
||||||
|
parts = parts[pairs, ...]
|
||||||
|
else:
|
||||||
|
parts = parts[:, pairs, ...]
|
||||||
|
|
||||||
|
return parts
|
||||||
|
|
||||||
|
|
||||||
|
def flip(tensor, is_label=False):
|
||||||
|
"""Flip an image or a set of heatmaps left-right
|
||||||
|
|
||||||
|
Arguments:
|
||||||
|
tensor {numpy.array or torch.tensor} -- [the input image or heatmaps]
|
||||||
|
|
||||||
|
Keyword Arguments:
|
||||||
|
is_label {bool} -- [denote wherever the input is an image or a set of heatmaps ] (default: {False})
|
||||||
|
"""
|
||||||
|
if not torch.is_tensor(tensor):
|
||||||
|
tensor = torch.from_numpy(tensor)
|
||||||
|
|
||||||
|
if is_label:
|
||||||
|
tensor = shuffle_lr(tensor).flip(tensor.ndimension() - 1)
|
||||||
|
else:
|
||||||
|
tensor = tensor.flip(tensor.ndimension() - 1)
|
||||||
|
|
||||||
|
return tensor
|
||||||
|
|
||||||
|
# From pyzolib/paths.py (https://bitbucket.org/pyzo/pyzolib/src/tip/paths.py)
|
||||||
|
|
||||||
|
|
||||||
|
def appdata_dir(appname=None, roaming=False):
|
||||||
|
""" appdata_dir(appname=None, roaming=False)
|
||||||
|
|
||||||
|
Get the path to the application directory, where applications are allowed
|
||||||
|
to write user specific files (e.g. configurations). For non-user specific
|
||||||
|
data, consider using common_appdata_dir().
|
||||||
|
If appname is given, a subdir is appended (and created if necessary).
|
||||||
|
If roaming is True, will prefer a roaming directory (Windows Vista/7).
|
||||||
|
"""
|
||||||
|
|
||||||
|
# Define default user directory
|
||||||
|
userDir = os.getenv('FACEALIGNMENT_USERDIR', None)
|
||||||
|
if userDir is None:
|
||||||
|
userDir = os.path.expanduser('~')
|
||||||
|
if not os.path.isdir(userDir): # pragma: no cover
|
||||||
|
userDir = '/var/tmp' # issue #54
|
||||||
|
|
||||||
|
# Get system app data dir
|
||||||
|
path = None
|
||||||
|
if sys.platform.startswith('win'):
|
||||||
|
path1, path2 = os.getenv('LOCALAPPDATA'), os.getenv('APPDATA')
|
||||||
|
path = (path2 or path1) if roaming else (path1 or path2)
|
||||||
|
elif sys.platform.startswith('darwin'):
|
||||||
|
path = os.path.join(userDir, 'Library', 'Application Support')
|
||||||
|
# On Linux and as fallback
|
||||||
|
if not (path and os.path.isdir(path)):
|
||||||
|
path = userDir
|
||||||
|
|
||||||
|
# Maybe we should store things local to the executable (in case of a
|
||||||
|
# portable distro or a frozen application that wants to be portable)
|
||||||
|
prefix = sys.prefix
|
||||||
|
if getattr(sys, 'frozen', None):
|
||||||
|
prefix = os.path.abspath(os.path.dirname(sys.executable))
|
||||||
|
for reldir in ('settings', '../settings'):
|
||||||
|
localpath = os.path.abspath(os.path.join(prefix, reldir))
|
||||||
|
if os.path.isdir(localpath): # pragma: no cover
|
||||||
|
try:
|
||||||
|
open(os.path.join(localpath, 'test.write'), 'wb').close()
|
||||||
|
os.remove(os.path.join(localpath, 'test.write'))
|
||||||
|
except IOError:
|
||||||
|
pass # We cannot write in this directory
|
||||||
|
else:
|
||||||
|
path = localpath
|
||||||
|
break
|
||||||
|
|
||||||
|
# Get path specific for this app
|
||||||
|
if appname:
|
||||||
|
if path == userDir:
|
||||||
|
appname = '.' + appname.lstrip('.') # Make it a hidden directory
|
||||||
|
path = os.path.join(path, appname)
|
||||||
|
if not os.path.isdir(path): # pragma: no cover
|
||||||
|
os.mkdir(path)
|
||||||
|
|
||||||
|
# Done
|
||||||
|
return path
|
|
@ -0,0 +1,57 @@
|
||||||
|
import torch
|
||||||
|
import time
|
||||||
|
import os
|
||||||
|
import cv2
|
||||||
|
import numpy as np
|
||||||
|
from PIL import Image
|
||||||
|
from .model import BiSeNet
|
||||||
|
import torchvision.transforms as transforms
|
||||||
|
|
||||||
|
class FaceParsing():
|
||||||
|
def __init__(self,resnet_path='./models/face-parse-bisent/resnet18-5c106cde.pth',
|
||||||
|
model_pth='./models/face-parse-bisent/79999_iter.pth'):
|
||||||
|
self.net = self.model_init(resnet_path,model_pth)
|
||||||
|
self.preprocess = self.image_preprocess()
|
||||||
|
|
||||||
|
def model_init(self,
|
||||||
|
resnet_path,
|
||||||
|
model_pth):
|
||||||
|
net = BiSeNet(resnet_path)
|
||||||
|
if torch.cuda.is_available():
|
||||||
|
net.cuda()
|
||||||
|
net.load_state_dict(torch.load(model_pth))
|
||||||
|
else:
|
||||||
|
net.load_state_dict(torch.load(model_pth, map_location=torch.device('cpu')))
|
||||||
|
net.eval()
|
||||||
|
return net
|
||||||
|
|
||||||
|
def image_preprocess(self):
|
||||||
|
return transforms.Compose([
|
||||||
|
transforms.ToTensor(),
|
||||||
|
transforms.Normalize((0.485, 0.456, 0.406), (0.229, 0.224, 0.225)),
|
||||||
|
])
|
||||||
|
|
||||||
|
def __call__(self, image, size=(512, 512)):
|
||||||
|
if isinstance(image, str):
|
||||||
|
image = Image.open(image)
|
||||||
|
|
||||||
|
width, height = image.size
|
||||||
|
with torch.no_grad():
|
||||||
|
image = image.resize(size, Image.BILINEAR)
|
||||||
|
img = self.preprocess(image)
|
||||||
|
if torch.cuda.is_available():
|
||||||
|
img = torch.unsqueeze(img, 0).cuda()
|
||||||
|
else:
|
||||||
|
img = torch.unsqueeze(img, 0)
|
||||||
|
out = self.net(img)[0]
|
||||||
|
parsing = out.squeeze(0).cpu().numpy().argmax(0)
|
||||||
|
parsing[np.where(parsing>13)] = 0
|
||||||
|
parsing[np.where(parsing>=1)] = 255
|
||||||
|
parsing = Image.fromarray(parsing.astype(np.uint8))
|
||||||
|
return parsing
|
||||||
|
|
||||||
|
if __name__ == "__main__":
|
||||||
|
fp = FaceParsing()
|
||||||
|
segmap = fp('154_small.png')
|
||||||
|
segmap.save('res.png')
|
||||||
|
|
|
@ -0,0 +1,283 @@
|
||||||
|
#!/usr/bin/python
|
||||||
|
# -*- encoding: utf-8 -*-
|
||||||
|
|
||||||
|
|
||||||
|
import torch
|
||||||
|
import torch.nn as nn
|
||||||
|
import torch.nn.functional as F
|
||||||
|
import torchvision
|
||||||
|
|
||||||
|
from .resnet import Resnet18
|
||||||
|
# from modules.bn import InPlaceABNSync as BatchNorm2d
|
||||||
|
|
||||||
|
|
||||||
|
class ConvBNReLU(nn.Module):
|
||||||
|
def __init__(self, in_chan, out_chan, ks=3, stride=1, padding=1, *args, **kwargs):
|
||||||
|
super(ConvBNReLU, self).__init__()
|
||||||
|
self.conv = nn.Conv2d(in_chan,
|
||||||
|
out_chan,
|
||||||
|
kernel_size = ks,
|
||||||
|
stride = stride,
|
||||||
|
padding = padding,
|
||||||
|
bias = False)
|
||||||
|
self.bn = nn.BatchNorm2d(out_chan)
|
||||||
|
self.init_weight()
|
||||||
|
|
||||||
|
def forward(self, x):
|
||||||
|
x = self.conv(x)
|
||||||
|
x = F.relu(self.bn(x))
|
||||||
|
return x
|
||||||
|
|
||||||
|
def init_weight(self):
|
||||||
|
for ly in self.children():
|
||||||
|
if isinstance(ly, nn.Conv2d):
|
||||||
|
nn.init.kaiming_normal_(ly.weight, a=1)
|
||||||
|
if not ly.bias is None: nn.init.constant_(ly.bias, 0)
|
||||||
|
|
||||||
|
class BiSeNetOutput(nn.Module):
|
||||||
|
def __init__(self, in_chan, mid_chan, n_classes, *args, **kwargs):
|
||||||
|
super(BiSeNetOutput, self).__init__()
|
||||||
|
self.conv = ConvBNReLU(in_chan, mid_chan, ks=3, stride=1, padding=1)
|
||||||
|
self.conv_out = nn.Conv2d(mid_chan, n_classes, kernel_size=1, bias=False)
|
||||||
|
self.init_weight()
|
||||||
|
|
||||||
|
def forward(self, x):
|
||||||
|
x = self.conv(x)
|
||||||
|
x = self.conv_out(x)
|
||||||
|
return x
|
||||||
|
|
||||||
|
def init_weight(self):
|
||||||
|
for ly in self.children():
|
||||||
|
if isinstance(ly, nn.Conv2d):
|
||||||
|
nn.init.kaiming_normal_(ly.weight, a=1)
|
||||||
|
if not ly.bias is None: nn.init.constant_(ly.bias, 0)
|
||||||
|
|
||||||
|
def get_params(self):
|
||||||
|
wd_params, nowd_params = [], []
|
||||||
|
for name, module in self.named_modules():
|
||||||
|
if isinstance(module, nn.Linear) or isinstance(module, nn.Conv2d):
|
||||||
|
wd_params.append(module.weight)
|
||||||
|
if not module.bias is None:
|
||||||
|
nowd_params.append(module.bias)
|
||||||
|
elif isinstance(module, nn.BatchNorm2d):
|
||||||
|
nowd_params += list(module.parameters())
|
||||||
|
return wd_params, nowd_params
|
||||||
|
|
||||||
|
|
||||||
|
class AttentionRefinementModule(nn.Module):
|
||||||
|
def __init__(self, in_chan, out_chan, *args, **kwargs):
|
||||||
|
super(AttentionRefinementModule, self).__init__()
|
||||||
|
self.conv = ConvBNReLU(in_chan, out_chan, ks=3, stride=1, padding=1)
|
||||||
|
self.conv_atten = nn.Conv2d(out_chan, out_chan, kernel_size= 1, bias=False)
|
||||||
|
self.bn_atten = nn.BatchNorm2d(out_chan)
|
||||||
|
self.sigmoid_atten = nn.Sigmoid()
|
||||||
|
self.init_weight()
|
||||||
|
|
||||||
|
def forward(self, x):
|
||||||
|
feat = self.conv(x)
|
||||||
|
atten = F.avg_pool2d(feat, feat.size()[2:])
|
||||||
|
atten = self.conv_atten(atten)
|
||||||
|
atten = self.bn_atten(atten)
|
||||||
|
atten = self.sigmoid_atten(atten)
|
||||||
|
out = torch.mul(feat, atten)
|
||||||
|
return out
|
||||||
|
|
||||||
|
def init_weight(self):
|
||||||
|
for ly in self.children():
|
||||||
|
if isinstance(ly, nn.Conv2d):
|
||||||
|
nn.init.kaiming_normal_(ly.weight, a=1)
|
||||||
|
if not ly.bias is None: nn.init.constant_(ly.bias, 0)
|
||||||
|
|
||||||
|
|
||||||
|
class ContextPath(nn.Module):
|
||||||
|
def __init__(self, resnet_path, *args, **kwargs):
|
||||||
|
super(ContextPath, self).__init__()
|
||||||
|
self.resnet = Resnet18(resnet_path)
|
||||||
|
self.arm16 = AttentionRefinementModule(256, 128)
|
||||||
|
self.arm32 = AttentionRefinementModule(512, 128)
|
||||||
|
self.conv_head32 = ConvBNReLU(128, 128, ks=3, stride=1, padding=1)
|
||||||
|
self.conv_head16 = ConvBNReLU(128, 128, ks=3, stride=1, padding=1)
|
||||||
|
self.conv_avg = ConvBNReLU(512, 128, ks=1, stride=1, padding=0)
|
||||||
|
|
||||||
|
self.init_weight()
|
||||||
|
|
||||||
|
def forward(self, x):
|
||||||
|
H0, W0 = x.size()[2:]
|
||||||
|
feat8, feat16, feat32 = self.resnet(x)
|
||||||
|
H8, W8 = feat8.size()[2:]
|
||||||
|
H16, W16 = feat16.size()[2:]
|
||||||
|
H32, W32 = feat32.size()[2:]
|
||||||
|
|
||||||
|
avg = F.avg_pool2d(feat32, feat32.size()[2:])
|
||||||
|
avg = self.conv_avg(avg)
|
||||||
|
avg_up = F.interpolate(avg, (H32, W32), mode='nearest')
|
||||||
|
|
||||||
|
feat32_arm = self.arm32(feat32)
|
||||||
|
feat32_sum = feat32_arm + avg_up
|
||||||
|
feat32_up = F.interpolate(feat32_sum, (H16, W16), mode='nearest')
|
||||||
|
feat32_up = self.conv_head32(feat32_up)
|
||||||
|
|
||||||
|
feat16_arm = self.arm16(feat16)
|
||||||
|
feat16_sum = feat16_arm + feat32_up
|
||||||
|
feat16_up = F.interpolate(feat16_sum, (H8, W8), mode='nearest')
|
||||||
|
feat16_up = self.conv_head16(feat16_up)
|
||||||
|
|
||||||
|
return feat8, feat16_up, feat32_up # x8, x8, x16
|
||||||
|
|
||||||
|
def init_weight(self):
|
||||||
|
for ly in self.children():
|
||||||
|
if isinstance(ly, nn.Conv2d):
|
||||||
|
nn.init.kaiming_normal_(ly.weight, a=1)
|
||||||
|
if not ly.bias is None: nn.init.constant_(ly.bias, 0)
|
||||||
|
|
||||||
|
def get_params(self):
|
||||||
|
wd_params, nowd_params = [], []
|
||||||
|
for name, module in self.named_modules():
|
||||||
|
if isinstance(module, (nn.Linear, nn.Conv2d)):
|
||||||
|
wd_params.append(module.weight)
|
||||||
|
if not module.bias is None:
|
||||||
|
nowd_params.append(module.bias)
|
||||||
|
elif isinstance(module, nn.BatchNorm2d):
|
||||||
|
nowd_params += list(module.parameters())
|
||||||
|
return wd_params, nowd_params
|
||||||
|
|
||||||
|
|
||||||
|
### This is not used, since I replace this with the resnet feature with the same size
|
||||||
|
class SpatialPath(nn.Module):
|
||||||
|
def __init__(self, *args, **kwargs):
|
||||||
|
super(SpatialPath, self).__init__()
|
||||||
|
self.conv1 = ConvBNReLU(3, 64, ks=7, stride=2, padding=3)
|
||||||
|
self.conv2 = ConvBNReLU(64, 64, ks=3, stride=2, padding=1)
|
||||||
|
self.conv3 = ConvBNReLU(64, 64, ks=3, stride=2, padding=1)
|
||||||
|
self.conv_out = ConvBNReLU(64, 128, ks=1, stride=1, padding=0)
|
||||||
|
self.init_weight()
|
||||||
|
|
||||||
|
def forward(self, x):
|
||||||
|
feat = self.conv1(x)
|
||||||
|
feat = self.conv2(feat)
|
||||||
|
feat = self.conv3(feat)
|
||||||
|
feat = self.conv_out(feat)
|
||||||
|
return feat
|
||||||
|
|
||||||
|
def init_weight(self):
|
||||||
|
for ly in self.children():
|
||||||
|
if isinstance(ly, nn.Conv2d):
|
||||||
|
nn.init.kaiming_normal_(ly.weight, a=1)
|
||||||
|
if not ly.bias is None: nn.init.constant_(ly.bias, 0)
|
||||||
|
|
||||||
|
def get_params(self):
|
||||||
|
wd_params, nowd_params = [], []
|
||||||
|
for name, module in self.named_modules():
|
||||||
|
if isinstance(module, nn.Linear) or isinstance(module, nn.Conv2d):
|
||||||
|
wd_params.append(module.weight)
|
||||||
|
if not module.bias is None:
|
||||||
|
nowd_params.append(module.bias)
|
||||||
|
elif isinstance(module, nn.BatchNorm2d):
|
||||||
|
nowd_params += list(module.parameters())
|
||||||
|
return wd_params, nowd_params
|
||||||
|
|
||||||
|
|
||||||
|
class FeatureFusionModule(nn.Module):
|
||||||
|
def __init__(self, in_chan, out_chan, *args, **kwargs):
|
||||||
|
super(FeatureFusionModule, self).__init__()
|
||||||
|
self.convblk = ConvBNReLU(in_chan, out_chan, ks=1, stride=1, padding=0)
|
||||||
|
self.conv1 = nn.Conv2d(out_chan,
|
||||||
|
out_chan//4,
|
||||||
|
kernel_size = 1,
|
||||||
|
stride = 1,
|
||||||
|
padding = 0,
|
||||||
|
bias = False)
|
||||||
|
self.conv2 = nn.Conv2d(out_chan//4,
|
||||||
|
out_chan,
|
||||||
|
kernel_size = 1,
|
||||||
|
stride = 1,
|
||||||
|
padding = 0,
|
||||||
|
bias = False)
|
||||||
|
self.relu = nn.ReLU(inplace=True)
|
||||||
|
self.sigmoid = nn.Sigmoid()
|
||||||
|
self.init_weight()
|
||||||
|
|
||||||
|
def forward(self, fsp, fcp):
|
||||||
|
fcat = torch.cat([fsp, fcp], dim=1)
|
||||||
|
feat = self.convblk(fcat)
|
||||||
|
atten = F.avg_pool2d(feat, feat.size()[2:])
|
||||||
|
atten = self.conv1(atten)
|
||||||
|
atten = self.relu(atten)
|
||||||
|
atten = self.conv2(atten)
|
||||||
|
atten = self.sigmoid(atten)
|
||||||
|
feat_atten = torch.mul(feat, atten)
|
||||||
|
feat_out = feat_atten + feat
|
||||||
|
return feat_out
|
||||||
|
|
||||||
|
def init_weight(self):
|
||||||
|
for ly in self.children():
|
||||||
|
if isinstance(ly, nn.Conv2d):
|
||||||
|
nn.init.kaiming_normal_(ly.weight, a=1)
|
||||||
|
if not ly.bias is None: nn.init.constant_(ly.bias, 0)
|
||||||
|
|
||||||
|
def get_params(self):
|
||||||
|
wd_params, nowd_params = [], []
|
||||||
|
for name, module in self.named_modules():
|
||||||
|
if isinstance(module, nn.Linear) or isinstance(module, nn.Conv2d):
|
||||||
|
wd_params.append(module.weight)
|
||||||
|
if not module.bias is None:
|
||||||
|
nowd_params.append(module.bias)
|
||||||
|
elif isinstance(module, nn.BatchNorm2d):
|
||||||
|
nowd_params += list(module.parameters())
|
||||||
|
return wd_params, nowd_params
|
||||||
|
|
||||||
|
|
||||||
|
class BiSeNet(nn.Module):
|
||||||
|
def __init__(self, resnet_path='models/resnet18-5c106cde.pth', n_classes=19, *args, **kwargs):
|
||||||
|
super(BiSeNet, self).__init__()
|
||||||
|
self.cp = ContextPath(resnet_path)
|
||||||
|
## here self.sp is deleted
|
||||||
|
self.ffm = FeatureFusionModule(256, 256)
|
||||||
|
self.conv_out = BiSeNetOutput(256, 256, n_classes)
|
||||||
|
self.conv_out16 = BiSeNetOutput(128, 64, n_classes)
|
||||||
|
self.conv_out32 = BiSeNetOutput(128, 64, n_classes)
|
||||||
|
self.init_weight()
|
||||||
|
|
||||||
|
def forward(self, x):
|
||||||
|
H, W = x.size()[2:]
|
||||||
|
feat_res8, feat_cp8, feat_cp16 = self.cp(x) # here return res3b1 feature
|
||||||
|
feat_sp = feat_res8 # use res3b1 feature to replace spatial path feature
|
||||||
|
feat_fuse = self.ffm(feat_sp, feat_cp8)
|
||||||
|
|
||||||
|
feat_out = self.conv_out(feat_fuse)
|
||||||
|
feat_out16 = self.conv_out16(feat_cp8)
|
||||||
|
feat_out32 = self.conv_out32(feat_cp16)
|
||||||
|
|
||||||
|
feat_out = F.interpolate(feat_out, (H, W), mode='bilinear', align_corners=True)
|
||||||
|
feat_out16 = F.interpolate(feat_out16, (H, W), mode='bilinear', align_corners=True)
|
||||||
|
feat_out32 = F.interpolate(feat_out32, (H, W), mode='bilinear', align_corners=True)
|
||||||
|
return feat_out, feat_out16, feat_out32
|
||||||
|
|
||||||
|
def init_weight(self):
|
||||||
|
for ly in self.children():
|
||||||
|
if isinstance(ly, nn.Conv2d):
|
||||||
|
nn.init.kaiming_normal_(ly.weight, a=1)
|
||||||
|
if not ly.bias is None: nn.init.constant_(ly.bias, 0)
|
||||||
|
|
||||||
|
def get_params(self):
|
||||||
|
wd_params, nowd_params, lr_mul_wd_params, lr_mul_nowd_params = [], [], [], []
|
||||||
|
for name, child in self.named_children():
|
||||||
|
child_wd_params, child_nowd_params = child.get_params()
|
||||||
|
if isinstance(child, FeatureFusionModule) or isinstance(child, BiSeNetOutput):
|
||||||
|
lr_mul_wd_params += child_wd_params
|
||||||
|
lr_mul_nowd_params += child_nowd_params
|
||||||
|
else:
|
||||||
|
wd_params += child_wd_params
|
||||||
|
nowd_params += child_nowd_params
|
||||||
|
return wd_params, nowd_params, lr_mul_wd_params, lr_mul_nowd_params
|
||||||
|
|
||||||
|
|
||||||
|
if __name__ == "__main__":
|
||||||
|
net = BiSeNet(19)
|
||||||
|
net.cuda()
|
||||||
|
net.eval()
|
||||||
|
in_ten = torch.randn(16, 3, 640, 480).cuda()
|
||||||
|
out, out16, out32 = net(in_ten)
|
||||||
|
print(out.shape)
|
||||||
|
|
||||||
|
net.get_params()
|
Some files were not shown because too many files have changed in this diff Show More
Loading…
Reference in New Issue